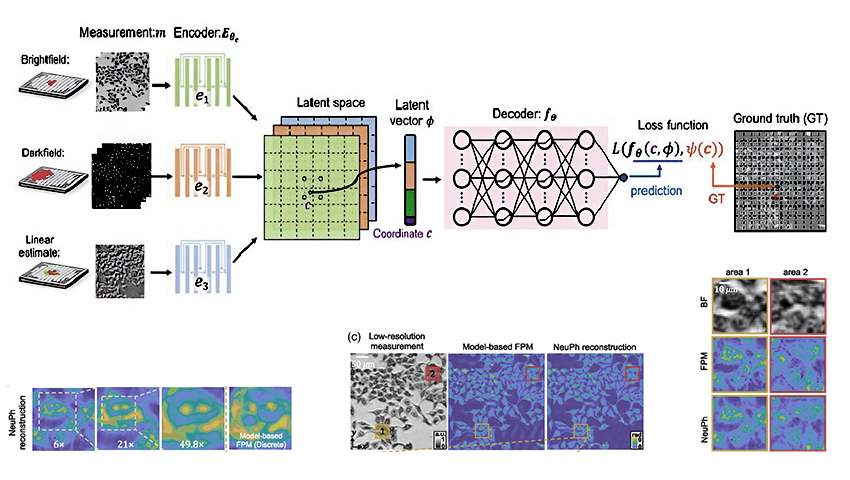
Deep Learning continues to push the limits of computer imaging, providing advanced solutions to challenges in image reconstruction. A recent innovation, developed by researchers from the IT Imaging Systems Laborate of the University of Boston, offers a scalable and generalizable neuronal framework known as Neuph (Neural Phase Retrieval), which considerably improves the reconstruction of high -resolution images from low -resolution data. This new approach combines advanced neural networks with an in -depth understanding of the structures of physical objects, allowing more precise and reliable reconstructions of images.
Historically, the methods of reconstruction of the image were based on discrete representations of pixels, limiting the capacity to capture the continuous and multi-scale nature of objects of the real world. These limitations are particularly obvious in fields such as biomedical imaging, where the capture of complex high -resolution structures is critical. Traditional methods, constrained by the diffraction limit and noise, often find it difficult to provide sufficient details. Neuph addresses this by taking advantage of the in -depth learning models which can interpret and reconstruct the characteristics of continuous objects from noisy and low resolution entrances.
In the heart of Neuph is a neural network architecture in two stages. The system first uses a convolutionary neural network encoder (CNN) which treats low -resolution images, compressing them in a latent space where key information is represented effectively. This latent space allows the system to manage complex structures without the need for a complete high resolution data input.
The second component is a Perceptron multilayer decoder (MLP), responsible for the reconstruction of high -resolution phase information of the latent representation. This approach allows the system to manage information to several scales, providing a more complete and detailed reconstruction than traditional pixel -based models. The result is a high quality image that captures subtle details and minimizes artifacts such as noise and phase non-packaging errors.
One of Neuph's remarkable characteristics is its ability to generalize in different sets of data and experimental conditions. Trained on simulated and experimental data, the system shows remarkable flexibility, functioning well even when the data is rare or imperfect. This generalization capacity is particularly important in real world applications, where training conditions often differ considerably from operational scenarios. Neuph's adaptability is still improved by its ability to reconstruct images that exceed the diffraction limit for entry measurements, reaching “super-resolution”.
Neuph's potential applications are vast. Its ability to provide reconstructions without resolution without resolution from limited data makes it an ideal candidate for various fields, in particular biomedical imagery, materials science and beyond. The combination of in -depth learning with physical models offers a path to more precise and evolving imaging systems, capable of managing the most complex structures and environments.
Explore more details on research in the publication in Spie digital library.
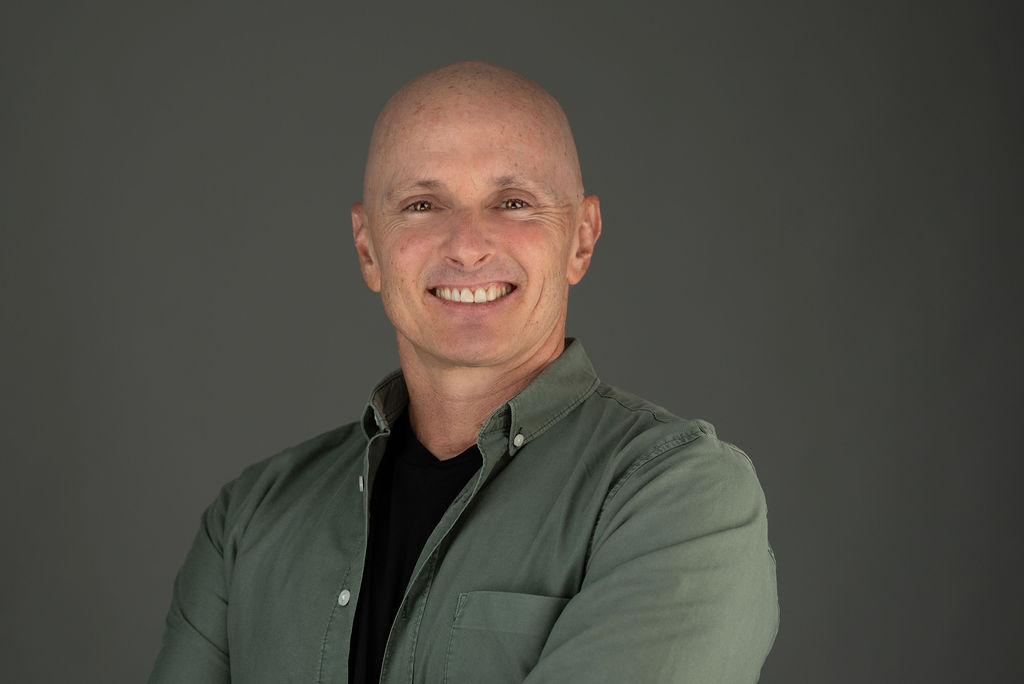