Recent progress in LLMs has considerably improved understanding of natural language, reasoning and generation. These models now excel in various tasks such as mathematical problems of problems and the generation of contextually appropriate text. However, a persistent challenge remains: the LLM often generates hallucinations – fluent but factually incorrect responses. These hallucinations undermine the reliability of LLM, in particular in the fields with high issues, which caused an urgent need for effective detection mechanisms. Although the use of LLMS to detect hallucinations seems promising, empirical evidence suggests that they fail in relation to human judgment and generally require external and annotated comments to function better. This raises a fundamental question: is the task of automated hallucination detection intrinsically difficult, or could it become more feasible as the models improve?
Theoretical and empirical studies have sought to respond to this. Drawing on frameworks of classical learning theory like Gold-English and recent adaptations to the generation of languages, researchers have analyzed if the reliable and representative generation is achievable under various constraints. Some studies highlight the intrinsic complexity of the detection of hallucinations, linking it to limits in the architectures of the model, such as the difficulties of transformers with the composition of large -scale function. On the empirical side, methods such as Selfcheckgpt assess the consistency of the answer, while others take advantage of the states of the internal model and supervised learning to report hallucinated content. Although supervised approaches using labeled data considerably improves detection, current -based detectors are still struggling without robust external guidance. These results suggest that during the progression, fully automated hallucination detection can face inherent theoretical and practical barriers.
Researchers at the University of Yale have a theoretical framework to assess whether hallucinations in LLM results can be detected automatically. Drawing on the Gold-English model for the identification of language, they show that hallucination detection is equivalent to identify if the results of an LLM belong to a correct language K. Their key conclusion is that the detection is fundamentally impossible when the training only uses correct (positive) examples. However, when negative examples – explicitly marked hallucinations – are included, detection becomes possible. This underlines the need for feedback marked by experts and supports methods such as learning to strengthen human feedback to improve LLM reliability.
The approach begins by showing that any algorithm capable of identifying a language within the limit can be transformed into the one that detects hallucinations in the limit. This implies using a language identification algorithm to compare LLM outputs with a language known over time. If deviations occur, hallucinations are detected. Conversely, the second part proves that the identification of language is not more difficult than the detection of hallucination. By combining a method of checking consistency with a hallucination detector, the algorithm identifies the correct language by excluding incoherent or mind -blowing candidates, finally selecting the smallest coherent and non -mind -blowing language.
The study defines a formal model where an learner interacts with an opponent to detect hallucinations – stages outside a target language – based on sequential examples. Each target language is a subset of a countable area, and the learner observes elements over time while questioning a set of candidates for membership. The main result shows that the detection of hallucinations within the limit is as difficult as identifying the correct language, which aligns with the characterization of angles. However, if the learner also receives labeled examples indicating whether the elements belong to the language, the hallucination detection becomes universally achievable for any countable collection of languages.

In conclusion, the study presents a theoretical framework to analyze the feasibility of automated hallucination detection in LLM. Researchers prove that the detection of hallucinations is equivalent to the problem of identification of the classic language, which is generally unrealizable when using correct examples. However, they show that the incorporation of incorrect (negative) labeled examples makes it possible to detect hallucination in all swapable languages. This highlights the importance of expert comments, such as RLHF, to improve the reliability of the LLM. Future guidelines include quantification of the quantity of negative data required, the management of noisy labels and the exploration of relaxed detection objectives based on the hallucination density thresholds.
Discover the Paper. Also, don't forget to follow us Twitter.
Here is a brief overview of what we build on Marktechpost:
ML new community – R / MACHINAGNINGNEWS (92K + members)
Bulletin- Airesearchinsights.com/(30k + subscribers)
Minicon AI events – minicon.marktechpost.com
AI reports and magazines – Magazine.Marktechpost.com
Ai Dev & Research News – marktechpost.com (1m + monthly readers)
Sana Hassan, consulting trainee at Marktechpost and double -degree student at Iit Madras, is passionate about the application of technology and AI to meet the challenges of the real world. With a great interest in solving practical problems, it brings a new perspective to the intersection of AI and real life solutions.
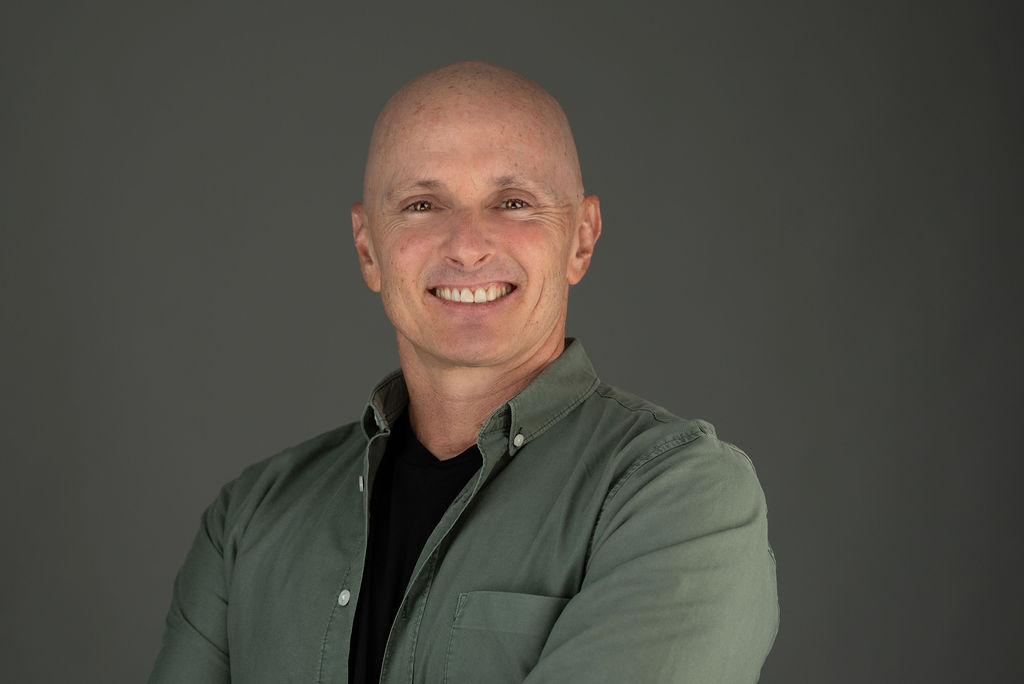