Researchers from the Institute of Computer Technology, Academy of Sciences, introduced LAMA-OMNI2A family of large language models compatible with speech (speech) now available on Face. This research introduces a modular framework which allows a dialogue spoken in real time by integrating the perception of speech and synthesis to the understanding of language. Unlike anterior cascade systems, Llama-OMNI2 works in an end pipeline while retaining modular interpretability and a low drive cost.
Overview of the Lama-OMNI architecture2
LLAMA-OMNI2 includes models ranging from 0.5b to 14b, each built at the top of the QWEN2.5-instrument series. Architecture consists of:
- Speech: Use Whisper-Garg-V3 to transform the word of entry into acoustic representations at the token level.
- Voice adapter: Treats the outputs of the encoder using a sampling reduction layer and a power network to align with the input space of the tongue model.
- Core LLM: QWEN2.5 models serve as a main reasoning engine.
- TTS Streaming decoder: Converts LLM released into speech tokens using a self -regressive transformer, then generates MEL spectrograms via a causal flow rate model inspired by Cosyvoice2.
A trigger mechanism merges LLM hidden states with textual incorporations before speech synthesis, improving contextual fidelity in the generated audio.

Streaming generation with reading planning
The model adopts a reading writing strategy to facilitate the streaming outing. More specifically, for each R
tokens produced by the LLM, W
Speech tokens are generated. This allows a synchronized textual and acoustic generation, minimizing latency without compromising control.
The empirical results suggest that adjustment R = 3 and W = 10 provides a favorable compromise between latency (~ 583 ms), alignment (ASR-WER: 3.26) and perceptual quality (UTMOS: 4.19).
Training approach
Despite competitive performance, Llama -OMNI2 is formed on a relatively compact corpus – 200K of multiple speech dialogue samples. These samples are synthesized from text data games according to the instructions (alpaca, ultrachat), with various entry votes and a coherent output voice generated using Fishspeech and Cosyvoice2 models.
The training is carried out in two stages:
- Step I: Independently optimizes the modules of speech and text and the text-spolate.
- Stadium II: Works the path of the generation of speech and speech, including the components of trigger for trigger and self -regressive decoding.
Reference results
The models are evaluated on the answer to the spoken question and the instruction of speech following tasks using the speech speech modes (S2T) and speech (S2S).
Model | LLAMA Q (S2S) | Web Q (S2S) | GPT-4O score | ASR-WER | Latence (MS) |
---|---|---|---|---|---|
GLM-4-TIFE (9b) | 50.7 | 15.9 | 4.09 | 3.48 | 1562.8 |
LLAMA-OMNI (8b) | 49.0 | 23.7 | 3.52 | 3.67 | 346.7 |
LAMA-OMNI2-7B | 60.7 | 31.3 | 4.15 | 3.26 | 582.9 |
The performance is coherently evolve with the size of the model. In particular, Llama-OMNI2-14B surpasses all the basic lines through tasks, even with training data significantly less than native words such as GLM-4-Voix.
Component analyzes
- Door merger module: The abolition of the trigger mechanism increases Asr-Wer and reduces the quality of speech, confirming its role in the alignment of textual and contextual signals.
- TTS Pre-training: The initialization of the TTS model from QWEN2.5 and the fine adjustment in a streaming configuration gives the best performance. Training from zero does not effectively converge.
- Reading / writing strategies: Adjustment of the R: W report has an impact on latency and quality. The largest W improves UTMOS but at the cost of the response delay.
In addition, the study demonstrates that multi-round dialogue data is more effective than data back in the process of interacting the word of training and that performance platforms approximately 200,000 samples.
Conclusion
LLAMA-OMNI2 shows that the interaction spoken of high quality and low latency with LLM is possible without the need for in-depth pre-training on corpus of massive discourse. By combining modular architecture with self -regressive streaming synthesis, the system offers a practical path for real -time vocal applications.
Discover the Paper,, Model on the embraced face And GitHub page. Also, don't forget to follow us Twitter.
Here is a brief overview of what we build on Marktechpost:
ML new community – R / MACHINAGNINGNEWS (92K + members)
Bulletin- Airesearchinsights.com/(30k + subscribers)
Minicon AI events – minicon.marktechpost.com
AI reports and magazines – Magazine.Marktechpost.com
Ai Dev & Research News – marktechpost.com (1m + monthly readers)
Asif Razzaq is the CEO of Marktechpost Media Inc .. as a visionary entrepreneur and engineer, AIF undertakes to exploit the potential of artificial intelligence for social good. His most recent company is the launch of an artificial intelligence media platform, Marktechpost, which stands out from its in-depth coverage of automatic learning and in-depth learning news which are both technically solid and easily understandable by a large audience. The platform has more than 2 million monthly views, illustrating its popularity with the public.
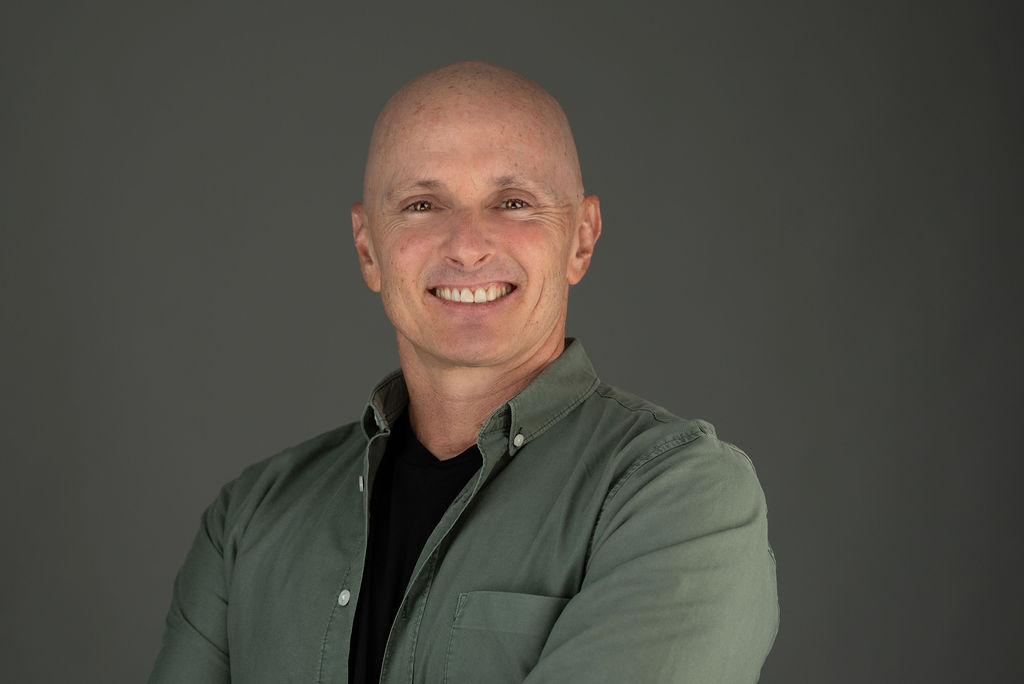