VLMs have become central to building AI systems for general use capable of understanding and interacting in digital and real parameters. By integrating visual and textual data, VLMs have motivated progress in multimodal reasoning, image editing, graphical interface agents, robotics, etc., influencing sectors such as education and health care. Despite these progress, VLMs are still lagging behind human capacities, in particular in tasks involving 3D reasoning, object count, creative visual interpretation and interactive gameplay. A challenge lies in the scarcity of rich and various multimodal data sets, unlike the abundant textual resources available for LLMS. In addition, the complexity of multimodal data poses significant training and evaluation obstacles.
The researchers of Bytedance have developed 1.5-VL seeds, a compact but powerful vision foundation model with a 532 m parameter vision coder and an LLM mixing 20 B settings. Despite its effective architecture, SEED1.5-VL obtains the best results on 38 of the 60 public VLM benchmarks, excellanting in tasks such as graphical interface control, visual reasoning. It is trained on billions of multimodal tokens using the advanced data synthesis and post-training techniques, including human feedback. Innovations in training, such as hybrid parallelism and redistribution of vision tokens, optimize performance. The effectiveness of the model and solid reasoning capacities are suitable for real interactive applications such as chatbots.
The SEED1.5-VL architecture has a vision encoder, an MLP adapter and an LLM. Its personalized vision encoder, Seed-Vit, supports the native resolution image input using 2D strings and deals with images through 14 × 14 fixes, followed by an average pooling and MLP. Pre-training implies modeling masked images, contrastive learning and omni-modal alignment using images, text and video-audio-copping. The model uses a dynamic sampling approach to image resolution for video coding that adapts the image frequencies and resolutions based on the complexity of the content, the balance between efficiency and details. This method allows an effective spatial-temporal understanding in a token budget, guaranteeing a complete video representation through various lengths and complexities.
The pre-training of SEED1.5-VL involved the conservation of 3 billions of high quality tokens in various fields. Image text pairs from the web have been filtered using clip scores, size / aspect and deduplication -to -redplication reports to reduce noise. By using sampling and duplication strategies based on the field, rare visual concepts have been over -represented to treat the imbalance in classes. Specialized data sets have been added for the OCR using images, graphics and tables rich in annotated and synthetic text – Earth and counting tasks of the objects used, delimitation boxes, points and web data for automatic marking. Additional tasks included a 3D spatial understanding using depth annotations and a video understanding via multi-trames subtitling, QA and temporal landing to support dynamic content analysis.
The evaluation highlights the competitive performance of Seed-Vit and Seed1.5-VL through the tasks of vision. Seed-Vit, despite having much fewer parameters, to correspond or to surpass larger models such as Intervl-C and EVA-Clip on zero images classification tasks, showing high precision and robustness on data sets such as Imagenet-A and ObjectNE. SEED1.5-VL demonstrates strong capacities of multimodal reasoning, general VQA, understanding of documents and earthing. He performs advanced references, in particular in the complex tasks of reasoning, counting and interpretation of the graphics. The “thought” mode of the model, which incorporates longer reasoning chains, still improves performance, indicating its strong capacity for visual understanding and generalization of detailed tasks.
In conclusion, Seed1.5-VL is a visual language foundation model with a vision encoder of 532 m parameters and a model of mixing language of 20 B parameters. Despite its compact size, it obtains advanced results on 38 of the 60 public landmarks and excels in complex reasoning, OCR, interpretation of the diagram, 3D spatial understanding and video analysis. It also works well in tasks focused on agents such as graphical interface control and gameplay, overtaking models like Openai Cua and Claude 3.7. The model shows a strong generalization to tasks beyond its training range. The study describes its architecture, its data pipeline and its training methods and identifies future orientations, in particular the improvement of the capacities for the use of tools and visual reasoning.
Discover the Paper And Project page. All the merit of this research goes to researchers in this project. Also, don't hesitate to follow us Twitter And don't forget to join our 90K + ML Subdreddit.
Sana Hassan, consulting trainee at Marktechpost and double -degree student at Iit Madras, is passionate about the application of technology and AI to meet the challenges of the real world. With a great interest in solving practical problems, it brings a new perspective to the intersection of AI and real life solutions.
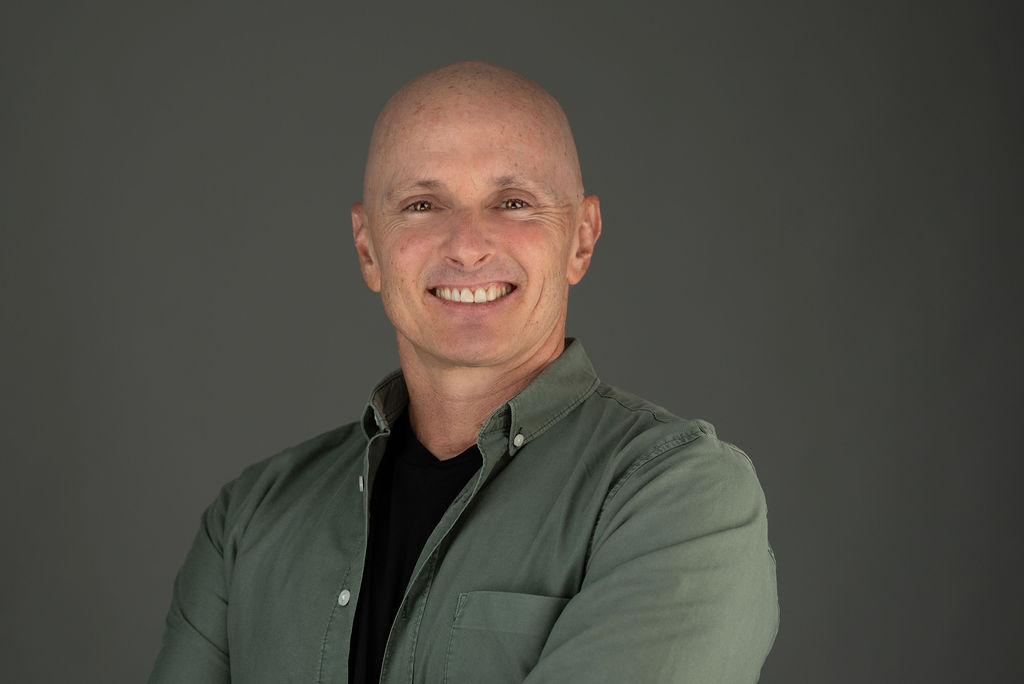