A protein located in the bad part of a cell can contribute to several diseases, such as Alzheimer, cystic fibrosis and cancer. But there are about 70,000 proteins and variants of different proteins in a single human cell, and as scientists can generally only test a handful in a single experience, it is extremely expensive and takes time to identify the locations of proteins manually.
A new generation of calculation techniques seeks to rationalize the process using automatic learning models which often use data sets containing thousands of proteins and their locations, measured on several cell lines. One of the largest data sets of this type is the human protein atlas, which catalogs the subcellular behavior of 13,000 proteins in more than 40 cell lines. But as huge as it is, the human protein atlas has explored only about 0.25% of all possible pairs of all proteins and cell lines in the database.
Now, MIT researchers, Harvard University and Broad Institute of Mit and Harvard have developed a new computer approach that can effectively explore the remaining unknown space. Their method can predict the location of any protein in a human cell line, even when proteins and cells have never been tested before.
Their technique goes a step further than many methods based on AI by locating a protein at the unique level, rather than an average estimate in all cells of a specific type. This monocellular location could identify the location of a protein in a specific cancer cell after treatment, for example.
The researchers combined a protein language model with a special type of computer vision model to capture rich details on a protein and a cell. In the end, the user receives an image of a cell with a highlighting part indicating the prediction of the model of the place where the protein is located. Since the location of a protein indicates its functional status, this technique could help researchers and clinicians diagnose disease more effectively or identify drug targets, while allowing biologists to better understand how complex biological processes are linked to the location of proteins.
“You can do these protein location experiences on a computer without having to touch any laboratory bench, hopefully, saving you months of effort. Although you still need to check the prediction, this technique could act as a first screening for what must test for the biology and conduct program in experimental biology ”, an article in research on this research.
TSEO is joined on the newspaper by the co-driven Xinyi Zhang, a graduate student from the Department of Electric and Computer Science (EECS) and the Eric and Wendy Schmidt Center at the Broad Institute; Yunhao Bai from the Broad Institute; And the senior authors Fei Chen, deputy professor at Harvard and member of the Broad Institute, and Caroline Uhler, Professor Andrew and Erna Viterbi of Engineering in EECS and MIT Institute for Data, Systems and Society (IDSS), who is also Director of Eric and Wendy Schmidt systems and a MIT researcher for information and decision -making systems (Lids). Research appears today in Nature methods.
Collaboration models
Many models for predicting existing proteins can only make predictions according to protein and cell data on which they have been formed or are unable to determine the location of a protein in a single cell.
To overcome these limits, the researchers created a two -part method for the prediction of the subcellular location of non -visible proteins, called puppies.
The first part uses a protein sequence model to capture the properties determining the location of a protein and its 3D structure based on the amino acid chain that shapes it.
The second part incorporates an image cutting model, which is designed to fill the missing parts of an image. This computer vision model examines three colored images of a cell to collect information on the condition of this cell, such as its type, its individual characteristics and if it is stress.
The puppies join the representations created by each model to predict where the protein is located in a single cell, using an image decoder to produce a highlighting image that shows the planned location.
“Different cells within a cellular line have different characteristics, and our model is capable of understanding this nuance,” explains TSEO.
A user enters the sequence of amino acids that form the protein and three cell coloring images – one for the nucleus, one for the microtubules and one for the endoplasmic reticulum. So the puppies do the rest.
A deeper understanding
The researchers used a few tips during the training process to teach puppies how to combine information from each model so that it can make an enlightened supposition on the location of the protein, even if it had not seen this protein before.
For example, they attribute to the model a secondary task during the formation: explicitly appoint the compartment of the location, such as the cell nucleus. This is done in parallel with the main intervention task to help the model learn more effectively.
A good analogy could be a teacher who asks their students to draw all parts of a flower in addition to writing their names. This additional step was found to help the model improve its general understanding of possible cellular compartments.
In addition, the fact that puppies are formed on proteins and cell lines at the same time the help of developing a deeper understanding of the place where in a cell image, proteins tend to locate.
The puppies can even understand, only how different parts of the sequence of a protein contribute separately to its global location.
“Most other methods generally require that you first have a protein stain, so you've ever seen them in your training data. Our approach is unique in that it can generalize between proteins and cell lines at the same time, ”explains Zhang.
Since puppies can be widespread in invisible proteins, it can grasp changes in the location caused by unique protein mutations that are not included in the human protein atlas.
Researchers have verified that puppies could predict the subcellular location of new proteins in invisible cell lines by conducting laboratory experiments and comparing the results. In addition, compared to a basic AI method, puppies have presented on average less prediction error through the proteins they tested.
In the future, researchers want to improve puppies so that the model can understand protein-protein interactions and make location predictions for several proteins within a cell. In the longer term, they want to allow puppies to make predictions in terms of living human tissue, rather than cultivated cells.
This research is funded by the Eric and Wendy Schmidt Center of the Broad Institute, the National Institutes of Health, the National Science Foundation, the Burroughs Welcome Fund, the Searle Scholars Foundation, the Harvard Stem Cell Institute, the Merkin Institute, the Office of Naval Research and the Department of Energy.
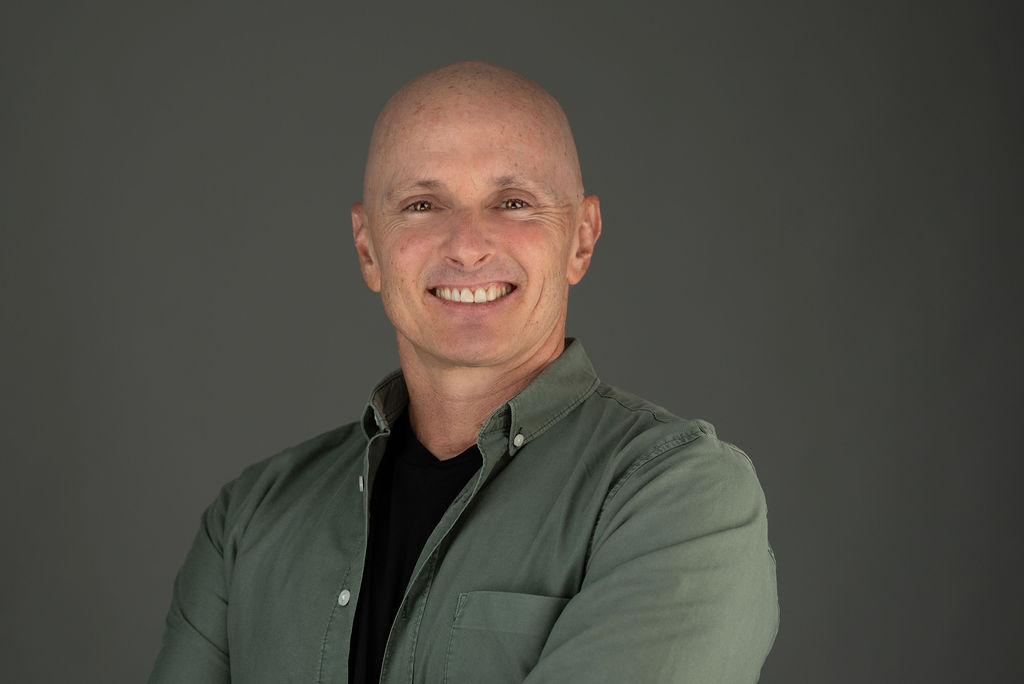