Large models of reasoning (LRM) have shown impressive capacities in mathematics, coding and scientific reasoning. However, they face important limits when they meet the complex information research needs when they only rely on internal knowledge. These models are struggling to search for in -depth web information and generate specific scientific relationships thanks to reasoning processes in several stages. Thus, the deep integration of LRM's reasoning capacities with the exploration of web information is a practical demand, initiating a series of in -depth research initiatives. However, existing open source research agents use CLOTH Techniques with rigid and predefined workflows, restrict the ability of LRM to explore deeper web information and hinder effective interaction between LRM and search engines.
LRMs as Openai-O1, Qwen-QWQ and Deepseek-R1 improve performance thanks to extensive reasoning capacities. Various strategies have been proposed to obtain advanced reasoning capacities, in particular intentional errors in reasoning during training, distilled training data and strengthening learning approaches to develop long chain capacities. However, these methods are fundamentally limited by their static and parameteric architectures that do not have access to external global knowledge. RAG incorporates recovery mechanisms with generative models, allowing access to external knowledge. Recent advances cover several dimensions, including the need for recovery, reformulation of queries, compression of documents, clearing and monitoring of instructions.
Researchers at the University of Renmin of China, Baai and Huawei Fish Lab proposed a deep research agent called Webthinker which allows LRMs to search for independent on the web, navigate web pages and write research reports during the reasoning process. Webthinker introduces a deep web explorer module that allows LRMs to search, navigate dynamically and extract web information when they meet knowledge gaps. He uses an autonomous thinking and research strategy, allowing models to combine reasoning, information collection and reporting in real time. In addition, a training strategy based on RL is implemented to improve the use of research tools thanks to the iterative optimization of direct online preferences.
Webthinker Framework works in two main modes: problem solving mode and gear generation mode. In problem solving mode, webthinker deals with complex tasks using the deep web explorer tool, which the LRM can invoke during reasoning. In report generation mode, the LRM autonomously produces detailed reports and uses an LLM assistant to implement report writing tools. To improve LRM with research tools via RL, webthinker generates various reasoning trajectories by applying its framework to an extensive set of reasoning datasets and generation of complex reports, including Supergpqa, Webwalkerqa, OpenHoughts, Naturalreason, NuminaMath and Glaive. For each request, the initial LRM produces several distinct trajectories.
The basic webthinker-32B model surpasses previous methods such as Search-O1 in all references on complex problem solving, with an improvement of 22.9% on webwalkerqa and 20.4% on Hle. WebThinker obtains the highest overall score of 8.0, exceeding basic rag lines and deep research systems advanced in scientific report generation tasks, including research in Gemini (7.9). Adaptability through different LRM skeletons is remarkable, with R1 -based webthinker models surpassing direct reasoning and standard base lines. With the backbone Deepseek-R1-7B, it reaches relative improvements of 174.4% on Gaia and 422.6% on webwalkerqa compared to direct generation, and 82.9% on Gaia and 161.3% on Webwalkerqa compared to the implementations of standard rags.
In conclusion, the researchers introduced Webthinker, which provides SGLB in -depth research capacities, addressing their limits in real tasks with high intensity of knowledge such as complex reasoning and generation of scientific relationships. The frame allows LRMS to explore the web independently and produce complete outputs thanks to continuous reasoning processes. The results highlight the potential of webthinker to advance the in -depth research capacities of LRMs, creating more powerful intelligent systems capable of meeting complex challenges of the real world. Future work includes the integration of multimodal reasoning capacities, exploring advanced tool learning mechanisms and the study of web exploration based on the graphical interface.
Discover the Paper. Also, don't forget to follow us Twitter.
Here is a brief overview of what we build on Marktechpost:
Sajjad Ansari is a last year's first year of the Kharagpur Iit. As a technology enthusiast, he plunges into AI's practical applications by emphasizing the understanding of the impact of AI technologies and their real implications. It aims to articulate complex AI concepts in a clear and accessible way.
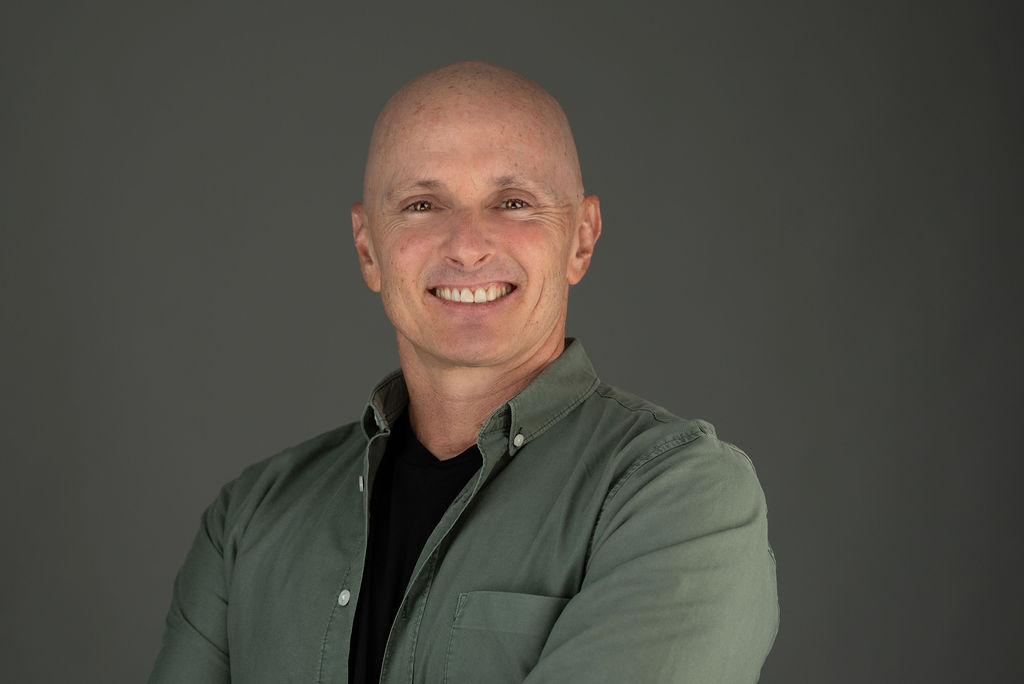