The recent progress of LM agents have shown promising potential to automate complex world tasks. These agents generally operate by offering and performing actions via APIs, by supporting applications such as software engineering, robotics and scientific experimentation. As these tasks become more complex, LM agent executives have evolved to include several agents, recovery in several steps and tailor -made scaffolding to optimize performance. A central challenge lies in the effective exploration and understanding of the environment, which caused the development of modified scaffolding using tools, memory mechanisms and personalized pipelines. However, most existing methods require partial observability, forcing agents to collect observations gradually. Although this hypothesis is in dynamic or unknown environments, it is less applicable in fully observable parameters like Swe-Bench, where all the relevant information is accessible from the start.
In software engineering, research on LM agents has focused on two main strategies: executives based on agents and structured pipelines. Systems based on agents, such as Swe-Agent and the Openhands Codet, allow LMS to interact independently with code bases, often via personalized interfaces and recovery tools. Other models such as Moatless and Autocoderoverover improve location through research techniques, while Specrover refines the design of scaffolding. Alternatively, structured pipelines – such as agent and codmonkey tasks – make up sequential phases such as location, repair and validation. Although these approaches depend on engineering components for performance, this study proposes to take advantage of LMS with long context (LCLMS) to directly interpret the environment of the task. The progress of LCLM architecture and infrastructure now allow these models to surpass systems from recovery in many contexts, reducing dependence on complex external scaffolding.
Researchers from Stanford, IBM and the University of Toronto have explored if a complex scaffolding is necessary for LM agents to tackle tasks like Swe-Bench. They show that simply the use of LCLM, such as Gemini-1.5-Pro, with an appropriate incentive and without scaffolding, can reach competitive performance-by reducing 38% on Swe-Bench-Verified. Gemini-2.5-Pro, using the same simple configuration, reached 50.8%. Their work suggests that many complex agent conceptions could be replaced by a single powerful LCLM, simplifying architecture and training. In addition, a hybrid approach in two stages using Gemini-1.5-Pro and Claude-3.7 reaches a resolution rate of 48.6%, supporting this simplified direction more.
Traditional LM agents depend on interactive exploration due to partial observability, but many tasks, as the software debugged, allow complete observability. The study offers state agents in context that exploit LCLM to directly treat complete or compressed environmental states, bypassing the need for complex agent scaffolding. For large code bases, compression based on ranking selects relevant files to adapt within context limits. Two methods are introduced: directs, where LCLM resolves tasks using the full context; And select, where LCLM locates the relevant files for LM (SCLMS) with a short context to be resolved. Both use targeted patch formats and validation to ensure precision and reduce hallucination.
Experiences assess a simplified agent manager using LLMS on the Swe-Bench verified reference, which includes 500 real software engineering tasks. The proposed methods, carry out and select, use LCLM like Gemini-1.5-Pro and Gemini-2.5-Pro, and in Selectsolave, an additional SCLM (Claude-3.7-Sonnet) for the generation of patchs. The results show that Difficace overperforms of complex agent approaches such as the agent without agent and the code with minimum engineering. Selectsolave further improves precision by taking advantage of the stronger correction models. Ablation studies highlight the importance of incitement to the COT, the retirement of the code and of the thrifty contextual design in tokens. In addition, the positioning of the files relevant at the start of the prompt improves performance, highlighting the limitations of long -context treatment.
In conclusion, the cost of using LCLM -based methods is currently higher than existing approaches such as the agent without an agent and the CODACT, on average $ 2.60 per instance, against $ 0.25 and $ 0.87, respectively. However, rapid reductions in inference costs and the increase in context lengths make LCLM more practical. Techniques such as KV cache considerably reduce costs after initial races, which reduced it to around $ 0.725. Although slight code base changes always limit the benefits of chatting, new improvements could help. The study also suggests that LCLM can manage long interaction stories, reducing the need for complex memory and recovery mechanisms. In particular, unrelated LCLM models can work competitively on Swe-Bench tasks.
Discover the Paper. All the merit of this research goes to researchers in this project. Also, don't hesitate to follow us Twitter And don't forget to join our 90K + ML Subdreddit.
Sana Hassan, consulting trainee at Marktechpost and double -degree student at Iit Madras, is passionate about the application of technology and AI to meet the challenges of the real world. With a great interest in solving practical problems, it brings a new perspective to the intersection of AI and real life solutions.
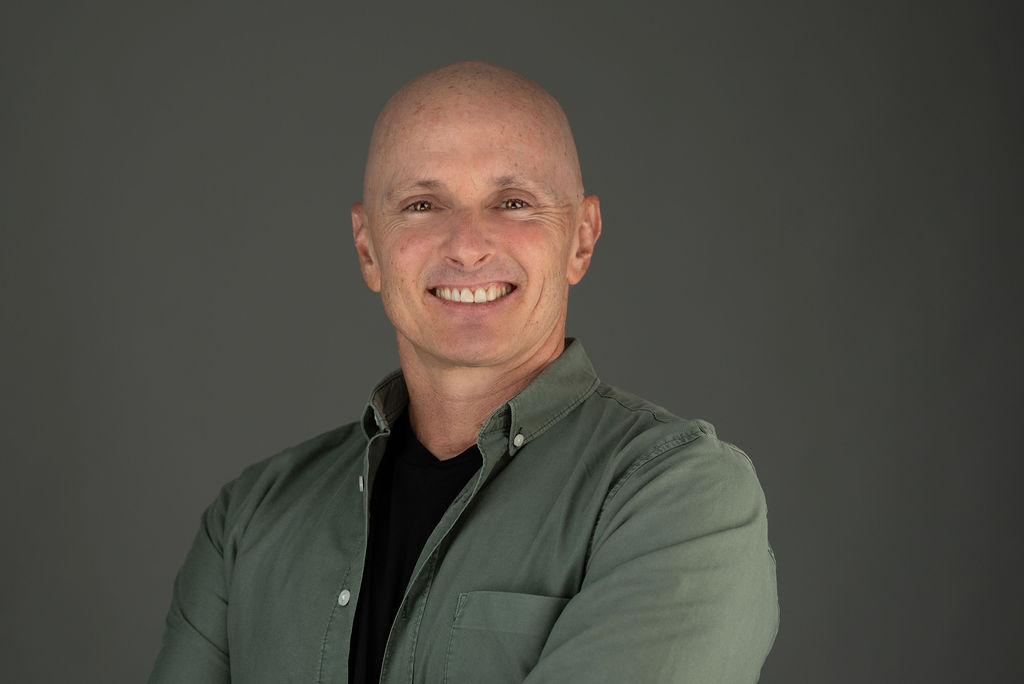