Artificial intelligence has developed beyond language-oriented systems, evolving towards models capable of processing several types of entry, such as text, images, audio and video. This area, known as multimodal learning, aims to reproduce natural human capacity to integrate and interpret various sensory data. Unlike conventional AI models which manage a single modality, multimodal general practitioners are designed to treat and respond between formats. The objective is to get closer to the creation of systems that imitate human cognition by transparently combining different types of knowledge and perception.
The challenge encountered in this area is to allow these multimodal systems to demonstrate real generalization. Although many models can process several entries, they often fail to transfer learning between tasks or methods. This lack of improvement in crossed tasks – known as synergy – Hindus are progressing towards smarter and adaptive systems. A model can exceed separately in the classification of images and text generation, but it cannot be considered as a robust general practitioner without the capacity to connect the skills of the two areas. The realization of this synergy is essential to develop more competent autonomous AI systems.
Many current tools are strongly based on models of large languages (LLM) at the base. These LLMs are often supplemented by external and specialized components adapted to image recognition or speech analysis tasks. For example, existing models such as Clip or Flamingo integrate language into vision but do not deeply connect both. Instead of operating as a unified system, they depend on cowardly coupled modules that imitate multimodal intelligence. This fragmented approach means that models do not have the internal architecture necessary for significant inter-modal learning, resulting in isolated task performance rather than holistic understanding.
Researchers from the National University of Singapore (NUSS), the Nanyang Technological University (NTU), the University of Zhejiang (ZJU), the University of Beijing (PKU) and others proposed an IA framework appointed general and a reference called General Banc. These tools are designed to measure and promote synergy between methods and tasks. The general level establishes five classification levels according to the way in which a model incorporates understanding, generation and linguistic tasks. The reference is supported by General Bench, a large set of data encompassing more than 700 tasks and 325,800 annotated examples from text, images, audio, video and 3D data.
The general level evaluation method is built on the concept of synergy. The models are evaluated by the performance of the tasks and their ability to exceed advanced specialized scores (SOTA) using shared knowledge. The researchers define three types of synergy – task task, generation of understanding and modality of modality and require an increasing capacity at each level. For example, a level 2 model takes care of many methods and tasks, while a level 4 model must present a synergy between understanding and generation. The scores are weighted to reduce the biases of the domination of the modality and encourage models to support a balanced range of tasks.
The researchers tested 172 major models, including more than 100 mllm the most efficient, against the general bench. The results have revealed that most models do not demonstrate the synergy necessary to qualify as general practitioners of higher level. Even advanced models like GPT-4V and GPT-4O have not reached level 5, which requires models to use non-languages inputs to improve language understanding. The most efficient models only managed basic multimodal interactions, and none has shown proof of total synergy between tasks and methods. For example, the reference showed 702 tasks evaluated on 145 skills, but no model has obtained domination in all areas. The coverage of General-Bench in 29 disciplines, using 58 evaluation measures, has established a new standard for exhaustiveness.
This research clarifies the gap between current multimodal systems and the ideal general model. Researchers approach a basic problem in multimodal AI by introducing hierarchical tools by integration on specialization. With the general level and the general bench, they offer a rigorous path to follow to assess and build models that manage various entries and learn and reason through them. Their approach helps to direct the terrain towards smarter systems with flexibility of the real world and an inter-model understanding.
Discover the Paper And Project page. All the merit of this research goes to researchers in this project. Also, don't hesitate to follow us Twitter And don't forget to join our 90K + ML Subdreddit.
Here is a brief overview of what we build on Marktechpost:
Nikhil is an intern consultant at Marktechpost. It pursues a double degree integrated into materials at the Indian Kharagpur Institute of Technology. Nikhil is an IA / ML enthusiast who is still looking for applications in fields like biomaterials and biomedical sciences. With a strong experience in material science, he explores new progress and creates opportunities to contribute.
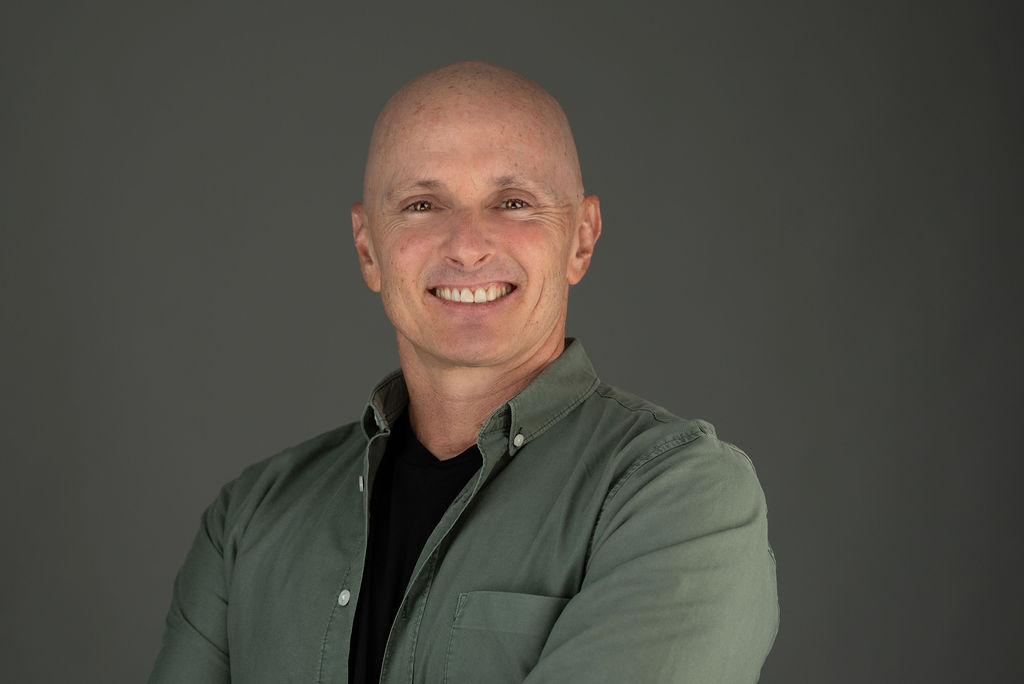