As agent AI systems are evolving, the complexity of ensuring their reliability, safety and safety increases accordingly. Recognizing this, the AI ββRed team of Microsoft (Airt) has published a Detailed taxonomy concerning the failure modes inherent in agent architectures. This report provides an essential basis for practitioners to design and maintain resilient agent systems.
Characterization of agentic AI and emerging challenges
Agenic AI systems are defined as autonomous entities that observe and act on their environment to achieve predefined objectives. These systems generally incorporate capacities such as autonomy, environmental observation, environmental interaction, memory and collaboration. Although these characteristics improve functionality, they also introduce a broader attack surface and new safety problems.
To inform their taxonomy, the Microsoft AI Red Red team conducted interviews with external practitioners, collaborated between internal research groups and expelled operational experience in the test of generative AI systems. The result is a structured analysis that distinguishes new modes of failure unique to agency systems and the amplification of risks already observed in generative IA contexts.
A framework for failure methods
Microsoft classifies two -dimensional failure modes: security And securityeach comprising the two novel And existing types.
- New safety failures: Including the compromise of agents, agent injection, agent identity, manipulation of agent flow and multi-agent jailbreaks.
- New safety failures: Covering problems such as intra-agent concerns responsible for AI (RAI), biases of the allocation of resources between several users, the degradation of organizational knowledge and the risks of prioritization having an impact on user safety.
- Existing safety failures: Encompassing memory intoxication, rapid injection of the cross -domain (XPIA), human puncture vulnerabilities in the loop, incorrect management of authorizations and insufficient isolation.
- Existing safety failures: Highlight risks such as the amplification of biases, hallucinations, the erroneous interpretation of instructions and a sufficient lack of transparency for a significant consent of users.
Each failure mode is detailed with its description, potential impacts, where it is likely to occur and illustrative examples.
Consequences of the failure of agent systems
The report identifies several systemic effects of these failures:
- Misalignment of agents: Differences in relation to the objectives of the user or the system provided.
- Agent abuse of action: Malicious exploitation of agent capacities.
- Service disturbance: Denial of the planned features.
- Incorrect decision -making: Defective outings caused by compromised processes.
- Erosion of user confidence: Loss of user confidence due to the unpredictability of the system.
- Environmental landing: Effects extending beyond the planned operational limits.
- Loss: Organizational or societal degradation of critical knowledge due to the overcoming of agents.
Attenuation strategies for agental AI systems
Taxonomy is accompanied by a set of design considerations aimed at reducing the identified risks:
- Identity management: Assign unique identifiers and granular roles to each agent.
- Directory of memory: Implementation of confidence limits for access to memory and rigorous surveillance.
- Control flow regulation: Determously governing the execution paths of agent workflows.
- Environmental isolation: Restrict the interaction of the predefined environmental boundaries agent.
- Transparent ux design: Ensure that users can provide informed consent according to the behavior of the clear system.
- Journalization and surveillance: Capture verifiable newspapers to allow a post-incidental analysis and detection of threats in real time.
- XPIA Defense: Minimize dependence on external unreliable data sources and separate data from the executable content.
These practices emphasize architectural provident and operational discipline to maintain the integrity of the system.
Case study: memory intoxication attack against an agency email assistant
Microsoft's report includes a case study demonstrating a memory intoxication attack against an E-mail assistant from AI implemented using Langchain, Langgraph and GPT-4O. The assistant, in charge of e-mail management, used a CLOTH– Memory system based.
An opponent introduced the poisoned content via a benign e-mail, exploiting the assistant's autonomous memory update mechanism. The agent was encouraged to transmit internal communications sensitive to an unauthorized external address. Initial tests have shown a success rate of 40%, which increased more than 80% after changing the assistant's invitation to prioritize memory.
This case illustrates the critical need for authenticated memorization, contextual validation of memory content and coherent memory recovery protocols.
Conclusion: towards secure and reliable agent systems
Microsoft's taxonomy provides a rigorous framework to anticipate and mitigate failure in agental AI systems. As the deployment of autonomous AI agents becomes more widespread, the systematic approaches to the identification and the fight against security and security will be vital.
Developers and architects must in depth the security and principles of AI responsible in the design of the aging system. Proactive attention to failure methods, associated with disciplined operational practices, will be necessary to ensure that agentic AI systems achieve their expected results without presenting unacceptable risks.
Discover the Guide. Also, don't forget to follow us Twitter And join our Telegram And Linkedin Group. Don't forget to join our 90K + ML Subdreddit.
Sana Hassan, consulting trainee at Marktechpost and double -degree student at Iit Madras, is passionate about the application of technology and AI to meet the challenges of the real world. With a great interest in solving practical problems, it brings a new perspective to the intersection of AI and real life solutions.
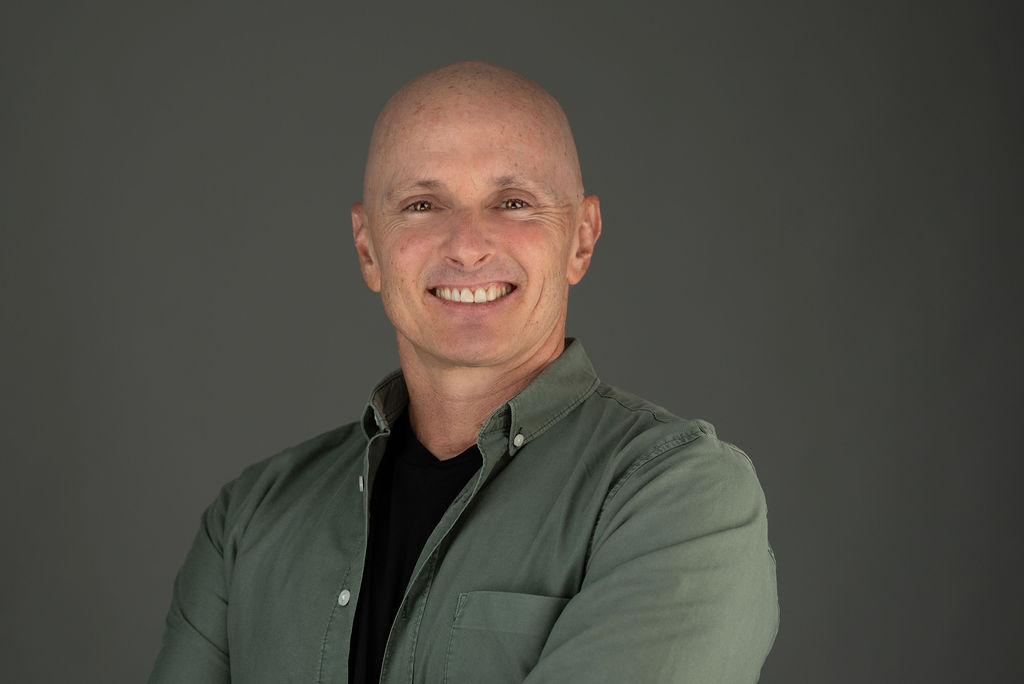