While automatic learning systems are an integral part of various applications, recommendations for autonomous systems, it is increasingly necessary to respond to their environmental sustainability. These systems require extended IT resources, often operating on tailor -made hardware accelerators. Their energy requirements are substantial during the training and inference phases, contributing to carbon operational emissions. In addition, the equipment that feeds these models has its environmental load, called embodied carbon, manufacturing, materials and life cycle operations. The fight against these double carbon sources is essential to reduce the ecological impact of automatic learning technologies, especially since global adoption continues to accelerate between industries and use cases.
Despite increasing awareness, current strategies to mitigate the impact of carbon of automatic learning systems remain fragmented. Most methods focus on operational efficiency, reduction in energy consumption during training and inference, or improving the use of equipment. However, few approaches consider the two sides of the equation: the carbon emitted during the material operation and the one which is integrated into the process of designing and making equipment. This divided perspective neglects the way in which decisions made at the model design stage influence material efficiency and vice versa. Multimodal models, which integrate visual and textual data, exacerbate this problem due to their intrinsically complex and heterogeneous IT requirements.
Several techniques currently used to improve the efficiency of the AI model, including pruning and distillation, aim to maintain precision while reducing inference time or energy consumption. Neuronal architecture research methods (NAS) are aware of the equipment explore architectural variants to refine performance, generally promoting latency or minimization of energy. Despite their sophistication, these methods often do not take into account the embodied carbon, the emissions linked to the construction and life of the physical material. Managers such as Act, Imec.netzero and Llmcarbon have recently started to model the carbon embodied independently, but they do not have the necessary integration for holistic optimization. Similarly, clip adaptations for the use of the edges, including the Tinclip models and based on Vit, prioritize the feasibility and the speed of deployment, overlooking total carbon production. These approaches provide partial solutions that are effective in their scope but insufficient for significant environmental mitigation.
Researchers from Fair At Meta and Georgia Institute of Technology have developed CatransformatorsA frame that introduces carbon as a main design consideration. This innovation allows researchers to cooptimize model architectures and material accelerators by jointly assessing their performance against carbon metrics. The solution targets the devices for the inference of the edges, where the embodied and operational emissions must be controlled due to material constraints. Unlike traditional methods, catransformators allows an exploration of early design spaces using a multi-objective Bayesian optimization engine which assesses compromise between latency, energy consumption, precision and total carbon footprint. This double consideration allows configurations of models that reduce emissions without sacrificing the quality or reactivity of models, offering a significant step towards sustainable AI systems.
The main functionality of the catransformators lies in its three modules architecture:
- A multi-objective optimizer
- A Ml model assessor
- A material estimator
The model assessor generates model variants by pruning a large basic clip model, modifying the dimensions such as the number of layers, the size of the network with direct action, attention heads and incorporation width. These pruned versions are then transmitted to the equipment estimator, which uses profiling tools to estimate the latency, the energy consumption of each configuration and total carbon emissions. The optimizer then selects the most efficient configurations by balancing all measures. This structure allows a rapid evaluation of interdependencies between the design of the model and the material deployment, offering a precise overview of the way in which architectural choices affect total emissions and performance results.
The practical production of catransformators is the family of Carbonclip models, which provides substantial gains on existing small -scale basic lines. Carbonclip-S reaches the same precision as Tinyclip-39m but reduces total carbon emissions by 17% and maintains latency under 15 milliseconds. Carbonclip-XS, a more compact version, offers 8% better precision than Tinyclip-8M while reducing emissions by 3% and guaranteeing latency remains less than 10 milliseconds. In particular, when comparing optimized configurations only for latency, material requirements have often doubled, leading to a significantly higher embodied carbon. On the other hand, the configurations optimized for carbon and latency carried out a reduction of 19 to 20% of total emissions with a minimum of latency compromise. These results highlight the importance of the integrated carbon design.
Several key dishes in catransformators research include:
- Catransformers introduce conscious cooptimization of carbon for automatic learning systems by assessing operational and embodied carbon emissions.
- The framework applies multi-objective Bayesian optimization, the integration of precision, latency, energy and carbon footprint in the research process.
- A family of models based on clips, carbonclip-s and carbonclip-Xs, has been developed using this method.
- Carbonclip-S reaches a 17% reduction in emissions compared to Tinclip-39m, with similar precision and
- Carbonclip-XS offers an improved precision of 8% on Tinyclip-8M while reducing carbon by 3% and reaching
- Optimized conceptions only for latency have led to an increased increase in 2.4 × in embodied carbon, showing the risk of ignoring sustainability.
- Combined optimization strategies have provided 19 to 20% carbon reductions with minimum latency increases, demonstrating a practical compromise path.
- The framework includes pruning strategies, a material estimate and an architectural simulation based on real material models.
- This research sets the basics of the sustainable design of the ML system by integrating environmental measures into the optimization pipeline.
In conclusion, this research highlights a practical path to the construction of environmental AI systems. By aligning the design of the model with the material capacities from the start and taking into account the impact on carbon, the researchers demonstrate that it is possible to make smarter choices that do not only hunt the speed or energy savings but really reduce emissions. The results emphasize that conventional methods can involuntarily cause higher carbon costs when optimized for close objectives such as latency. With catransformators, developers have a tool to rethink the way in which performance and durability can go hand in hand, especially since AI continues to extend between industries.
Discover the Paper And GitHub page. All the merit of this research goes to researchers in this project. Also, don't hesitate to follow us Chirping And don't forget to join our 90K + ML Subdreddit.
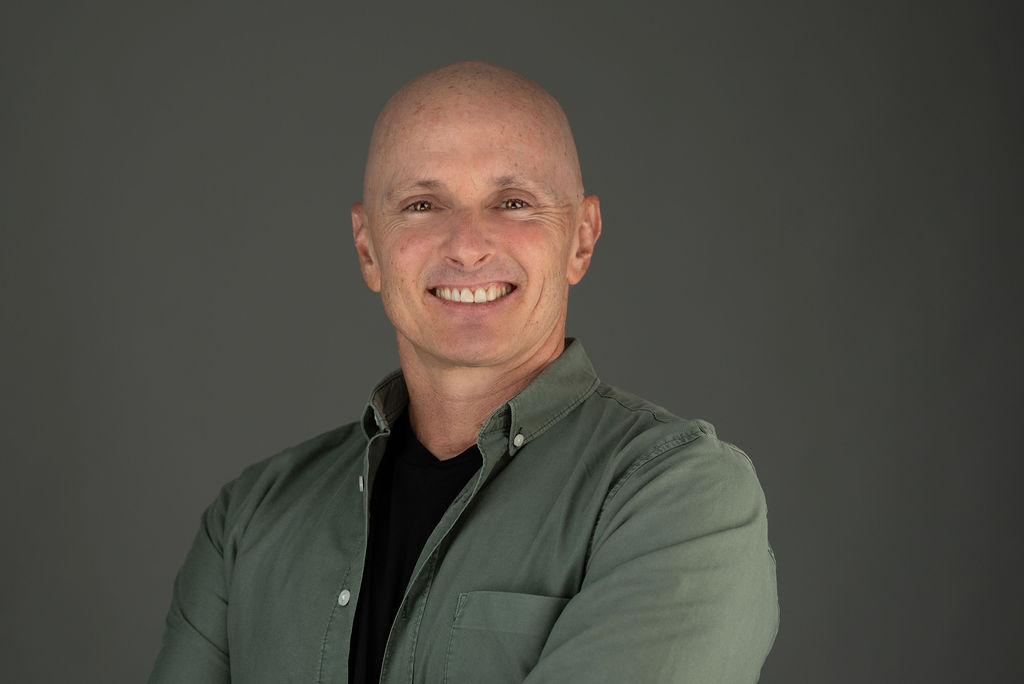