Semantic recovery focuses on understanding the meaning behind the text rather than the correspondence of keywords, allowing systems to provide results that align with the intention of the user. This capacity is essential in the fields which depend on the recovery of large -scale information, such as scientific research, legal analysis and digital assistants. Traditional methods based on keywords do not manage to grasp the nuance of human language, often recovering non -relevant or imprecise results. Modern approaches are based on the conversion of the text into high -dimension vector representations, allowing more significant comparisons between requests and documents. These interests aim to preserve semantic relationships and provide more relevant results contextually during recovery.
Among many, the main challenge of semantic recovery is the effective management of long documents and complex requests. Many models are limited by token windows of fixed length, generally around 512 or 1024 chips, which limits their application in areas that require processing of full length items or multi-paragraph documents. Consequently, the crucial information that appears further in a document can be ignored or truncated. In addition, real -time performance is often compromised due to the cost of calculating the incorporation and the comparison of large documents, in particular when indexing and the request must occur on a large scale. The scalability, precision and generalization to invisible data remain persistent challenges in the deployment of these models in dynamic environments.
In previous research, models like Modernbert and other tools based on phrase transformers have dominated the space of semantic integration. They often use an average pooling or simple aggregation techniques to generate sentences of phrases on contextual incorporations. Although such methods work for short and moderate length documents, they find it difficult to maintain accuracy in the face of longer input sequences. These models are also based on dense vector comparisons, which become expensive in calculation when managing millions of documents. In addition, even if they operate on standard benchmarks like Ms. Marco, they show a reduced generalization to various data sets, and a replay for specific contexts is often necessary.
Lighton AI researchers have introduced GTE-MODERNCOLBERT-V1. This model is based on Colbert architecture, incorporating the Modernbert foundation developed by Alibaba-NLP. By distilling the knowledge of a basic model and optimizing it on the MS Marco data, the team aimed to overcome the limitations linked to the length of the context and to semantic preservation. The model was formed using document entries at 300 tonnes, but has demonstrated the capacity to manage the entries as large as chips 8192. This makes it suitable for indexing and recovery of longer documents with a minimum loss of information. Their work was deployed via Pylate, a library that simplifies indexing and questioning of documents using dense vector models. The model supports semantic correspondence at the level of the token using the operator Maxsim, which assesses the similarity between the incorporations of individual tokens rather than compressing them in a single vector.
GTE-MODERNCOLBERT-V1 transforms the text into dense vectors at 128 dimensions and uses the MaxSIM function to calculate the semantic similarity between the query and document tokens. This method preserves the granular context and allows refined recovery. It fits into the Pylate travel indexing system, which manages large -scale integration using an effective HNSW index (Small World navigable hierarchical). Once the documents are integrated and stored, users can recover the relevant TOP-K documents using the Colbert Retriever. The process supports the complete indexing of pipelines and light replay for recovery systems in the first step. Pylate offers flexibility in modifying the length of the document during inference, allowing users to manage texts much longer than the model was initially formed, an advantage rarely seen in standard incorporation models.
On the Nanoclimat data set, the model obtained a MAXSIM @ 1 precision on 0.360, the precision at 5 of 0.780 and the precision at 10 on 0.860. The precision and recall scores were consistent, with a MAXSIM @ 3 reminder reaching 0.289 and precision @ 3 to 0.233. These scores reflect the capacity of the model to recover specific results even in longer context recovery scenarios. When evaluated on the Beir reference, GTE-Moderncolbert has surpassed the previous models, including Colbert-Small. For example, he marked 54.89 on the FIQA2018, 48.51 data set on NFCORPUS and 83.59 on the TREC-COVIVE task. The average performance between these tasks was significantly higher than the basic variants of Colbert. In particular, in the reference to Longled, the model marked 88.39 in average score and 78.82 in the recovery of the narrative narrative Lemb, exceeding other leading models such as the voyage-multi-multiling-2 (79.17) and the BGE-M3 (58.73).
These results suggest that the model offers robust generalization and effective management of long -context documents, surpassing many contemporaries of almost 10 points on long -context tasks. It is also highly adaptable to different recovery pipelines, supporting indexing and replaying implementations. Such versatility makes it an attractive solution for evolutionary semantic research.
Several key points of research on GTE-Moderncolbert-V1 include:
- GTE-MODERNCOLBERT-V1 uses dense vectors 128 dimensions with a MaxSim similarity at the tokens, based on the foundations of Colbert and Modernbert.
- Although trained on 300 -ton documents, the model is widespread in documents up to 8192 tokens, showing adaptability for long -context recovery tasks.
- The precision @ 10 A reached 0.860, the reminder @ 3 was 0.289 and the precision @ 3 was 0.233, demonstrating a high recovery precision.
- On the Beir benchmark, the model marked 83.59 on TREC-COVVID and 54.89 on the FIQA2018, outperforming Colbert-Small and other basic lines.
- Obtained an average score of 88.39 in the long -term reference and 78.82 in the story of the Lemb QA, exceeding the previous Sota of almost 10 points.
- Integrates into the Pylate Voyager index, supports the reproductive and recovery pipelines and is compatible with effective HNSW indexation.
- The model can be deployed in pipelines requiring rapid and evolving documents, including academic, businesses and multilingual applications.
In conclusion, this research makes a significant contribution to long -document semantic recovery. By combining the forces of correspondence at the level of tokens with evolutionary architecture, GTE-Moderncolbert-V1 addresses several bottlenecks with which the current models are confronted. It introduces a reliable method to process and recover semantically rich information from extensive contexts, considerably improving precision and recall.
Discover the Model on the embraced face. All the merit of this research goes to researchers in this project. Also, don't hesitate to follow us Twitter And don't forget to join our 90K + ML Subdreddit.
Here is a brief overview of what we build on Marktechpost:
Asif Razzaq is the CEO of Marktechpost Media Inc .. as a visionary entrepreneur and engineer, AIF undertakes to exploit the potential of artificial intelligence for social good. His most recent company is the launch of an artificial intelligence media platform, Marktechpost, which stands out from its in-depth coverage of automatic learning and in-depth learning news which are both technically solid and easily understandable by a large audience. The platform has more than 2 million monthly views, illustrating its popularity with the public.
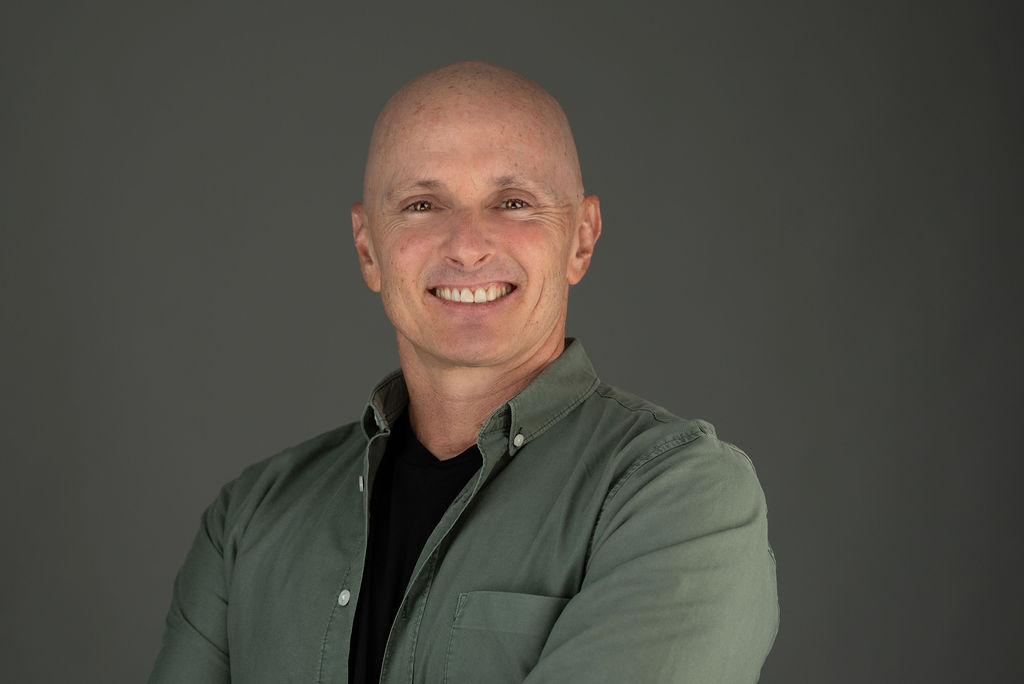