The manipulation of lighting conditions in the post-capture images is difficult. Traditional approaches are based on 3D graphic methods that reconstruct geometry and scene properties of several catches before simulating new lighting using physical lighting models. Although these techniques provide explicit control over light sources, recovery of precise 3D models from unique images remains a problem that frequently leads to unsatisfactory results. Modern dissemination -based image assembly methods have become alternatives that use solid statistical prayers to bypass physical modeling requirements. However, these approaches fight with precise parametric control due to their inherent stochasticity and their dependence on textual conditioning.
Generating image modification methods have been adapted for various reduction tasks with mixed results. Portrait reduction approaches often use light phase data to supervise generative models, while object reduction methods can refine dissemination models using synthetic data sets packaged on environment cards. Certain methods assume a single dominant source of light for external scenes, such as the sun, while the interior scenes have more complex multi-illumination challenges. Various approaches approach these problems, including reverse rendering networks and methods that handle the latent space of stylegan. Research on flash photography shows progress in multi-illumming publishing thanks to techniques that use flash / flash pairs to disentangle and manipulate stage illuminants.
Researchers from Google, the University of Tel Aviv, Reichman University and the Hebrew University of Jerusalem proposed Lightlab, a method based on diffusion allowing an explicit parametric control of light sources in the images. It targets two fundamental properties of light sources, intensity and color. Lightlab provides a control over ambient lighting and tone cartography effects, creating a full set of publishing tools that allow users to handle the overall appearance and sensation of an image through lighting adjustments. The method shows the effectiveness on the interior images containing visible light sources, although additional results are promising for external scenes and examples outside the field. Comparative analysis confirms that Lightlab is a pioneer in the provision of a precise and precise control of visible local light sources.
Lightlab uses a pair of images to implicitly model the light changes in the image space, which then forms a specialized diffusion model. Data collection combines real photographs with synthetic renderings. The photography data set consists of 600 pairs of raw images captured using mobile devices on tripods, each pair showing identical scenes where only a visible light source is lit or deactivated. The parameters of the self-exposure and post-capal calibration guarantee appropriate exposure. A wider set of synthetic images is rendered from 20 interior 3D scenes created by artists to increase this collection using physically based on Blender. This synthetic pipeline randomly samples the views of the camera around target objects and prohibited the light source parameters, including the intensity, the color temperature, the size of the cone.
Comparative analysis shows that the use of a weighted mixture of real catches and synthetic rendering obtains optimal results in all parameters. The quantitative improvement in the addition of synthetic data to actual catches is relatively modest at only 2.2% of the PSNR, probably because important local illumination changes are overshadowed by low -frequency details at the image scale in these metrics. Qualitative comparisons on assessment data sets show Lightlab's superiority over competing methods like Omnigen, RGB ↔ X, Scribblelight and IC-Light. These alternatives often introduce unwanted lighting changes, a distortion of colors or geometric inconsistencies. On the other hand, Lightlab provides faithful control over target light sources while generating physically plausible lighting effects throughout the scene.
In conclusion, the researchers introduced Lightlab, a progression of the manipulation of the light source based on the diffusion for the images. Using light linearity principles and synthetic 3D data, researchers have created high -quality paired images that implicitly model complex lighting changes. Despite its forces, Lightlab faces limits of the bias of the data set, in particular with regard to the types of light source. This could be treated by integration with unpaid fine adjustment methods. In addition, although the simplistic data capture process using consumer mobile devices with a post-cap exposure calibration has facilitated data collection, it prevents a precise reduction in absolute physical units, indicating a place for additional refinement in future iterations.
Discover the Paper And Project page. All the merit of this research goes to researchers in this project. Also, don't hesitate to follow us Twitter And don't forget to join our 90K + ML Subdreddit.
Sajjad Ansari is a last year's first year of the Kharagpur Iit. As a technology enthusiast, he plunges into AI's practical applications by emphasizing the understanding of the impact of AI technologies and their real implications. It aims to articulate complex AI concepts in a clear and accessible way.
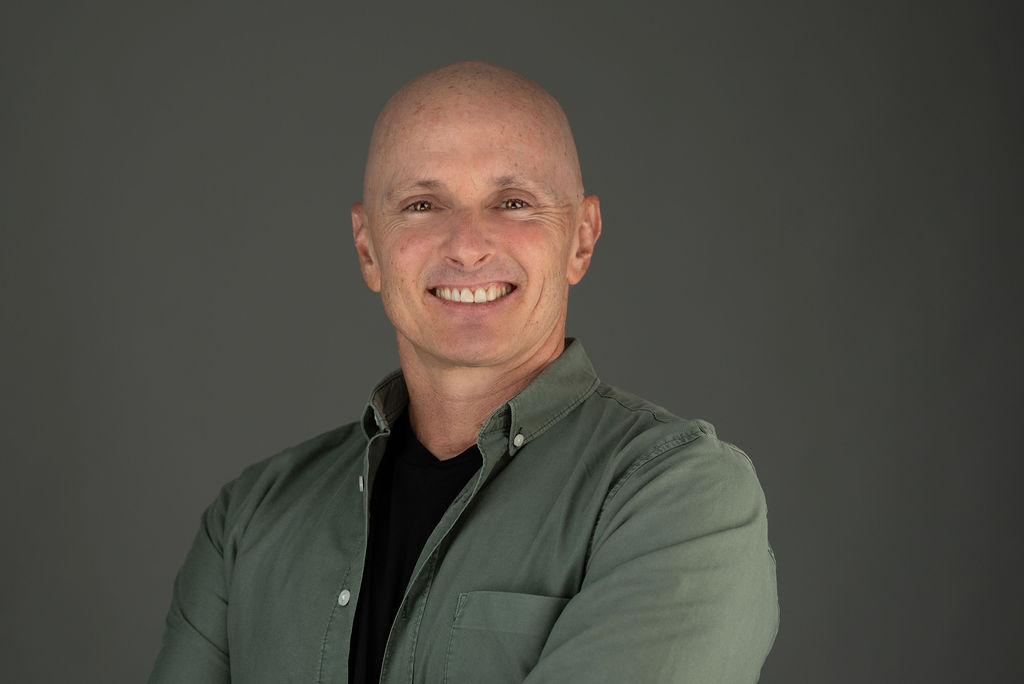