Linguistic treatment in corporate environments is confronted with critical challenges, as commercial work flows depend more and more on the summary of information from various sources, in particular internal documentation, code standards, research reports and real -time data flows. While recent progress in large -language models have provided impressive capacities, these progress is accompanied by significant drawbacks: the rowing of costs by demand, the constant material upgrading requirements and the increased risks of data confidentiality.
The continuation of the architectures of ever larger models has shown a decrease in yields, the acceleration of energy requirements potentially binding the future development of AI. Modern companies now require balanced solutions that offer a complete understanding of long -term contexts while maintaining effective treatment, low -cost service capacity and robust confidentiality guarantees – a combination that Small language models are unique to provide despite complex and high volume inference requests characteristics of today's commercial applications.
Traditional approaches to extend the capacities of the linguistic model beyond their inherent context limitations have relied on several bypass methods. Generation with recovery (CLOTH) Systems extract relevant information from external knowledge bases to complete the inputs of the model. External tool calls allow models to access specialized functions outside their settings. The memory mechanisms artificially persist the information through the conversation turns. Although functional, these techniques represent fragile “sewing” solutions which add potential complexity and failure points to treatment pipelines.
Context window extensions in larger models have tried to deal with these limits but introduced significant general costs. Each method fundamentally recognizes the same critical need: real long-context processing skills that allow models to manage whole documents, sustained conversations, code standards and research reports in a single pass before rather than fragmented treatment. These STOPGAP approaches highlight the reason why the extensive context is essential – it eliminates architectural complexity while maintaining the consistency of information throughout the processing.
Salesforce AI research has developed Xgen-SmallA compact -based compact language model for effective long -context treatment. This solution combines the conservation of data focused on the field, techniques of evolution of pre-training, length of length, fine adjustment of instructions and learning to strengthen to offer high performance corporate AI capacities with low predictable costs, the fight against the critical balance that companies need between capacities and operational efficiency.
Xgen-Small's architecture uses a “small but long” strategy which fundamentally reverses the traditional scaling paradigm. Rather than increasing the number of parameters, this approach deliberately reduces the size of the model while precisely refining data distributions to the areas and training protocols linked to the company. This architectural philosophy requires complete expertise on several stages of development and components working together via an integrated pipeline.
The frame begins with a meticulous conservation of the raw data followed by an evolving pre-training optimized for effective treatment. Sophisticated length length mechanisms allow the compact model to manage extensive contexts while targeted post-training and reinforcement techniques improve the performance of specific tasks. This architecture offers strategic advantages for commercial applications by providing profitability, robust confidentiality guarantees and long -term understanding without the requirements for larger model resources, creating a lasting way to deploy a large -scale business AI with predictable operational characteristics.
Xgen-Small's development pipeline incorporates several stages in a rationalized workflow. Starting with a corpus of several vority, the process applies filtering and rigorous quality controls before large-scale TPU pre-training with optimized learning hours. The targeted techniques of the extension length extend the context of context, while the capacity of the reinforcement model based on the specific training and reward.
The conservation of data for Xgen-Small began with the harvest of a large corpus than the eight bugs of training tokens. The pipeline applied rapid heuristic filters to eliminate spam, followed by a quality assessment in two stages using classifier sets. Exact chopping and fuzzy fingerprints eliminated the quasi-duplics, While carefully balancing general data with specialized content for code, mathematics and optimized performance in natural language. Vast ablation studies have refined this conservation approach to maximize factual precision and global utility.
The pre-training of Xgen-Small uses V5P TPU pods with the JAXFORMER V8 library, the implementation of the FSDP, the attention parallel to the sequence and the splash grains for maximum efficiency. The calendar of multiphasical learning rates optimizes training dynamics. At the same time, a mixture of carefully balanced data combines code corpus, examples of natural language, mathematical texts and high quality filtered content to grasp both diversity and expertise in the domain.
Xgen-Small demonstrates competitive performance against basic lines in its size class. The strategic mixture of various types of data – including low entropy code, high entropy natural language, mathematical content and high -quality subset filtered to the classifier – give exceptional results through evaluation measures while maintaining the compact and effective model of the model. This approach successfully balances the effectiveness of treatment with robust performance capacities required for business applications.
Performance assessments demonstrate the exceptional long-term context capacities of Xgen-Small, the 9B model achieving cutting-edge results on the rule reference and the 4B model securing second place in its class. Unlike competitors whose performances deteriorate considerably at extensive context lengths, Xgen maintains coherent performance from 4K to 128k tokens. This stability comes from a sophisticated length extension strategy using a two -step extension (32K then 128K), excessive training at 256k and a sequence parallelism to effectively manage memory constraints, offering reliable performance in the entire context spectrum.
Post-training transforms Xgen-Small basic models into models of complete instructions through a two-step process. First, the supervised fine adjustment uses a high -quality diverse diversified instructions set covering mathematical areas, coding, security and general use to establish behavior and basic alignment. Subsequently, learning by large -scale strengthening refines the policy of the model, in particular the improvement of reasoning capacities. This approach offers exceptional performance in complex reasoning areas such as mathematics, coding and STEM applications while retaining coherent instructions monitoring between general tasks.
The development of Xgen-Small shows that the constraint of the size of the model deliberately while extending the context of context creates optimal solutions for business AI applications. This “small but long” approach considerably reduces inference costs and material requirements while allowing transparent treatment of large internal sources of knowledge without external recovery dependencies. Thanks to an integrated meticulous data conservation pipeline, evolutionary pre-training, targeted length tension and reinforcement learning, these compact models correspond or exceed the performance of larger counterparts. This architecture provides companies with a predictable, sustainable, profitable, preserving confidentiality for the deployment of AI on a business scale.
Discover the Model on the embraced face And Technical details. Also, don't forget to follow us Twitter.
Here is a brief overview of what we build on Marktechpost:
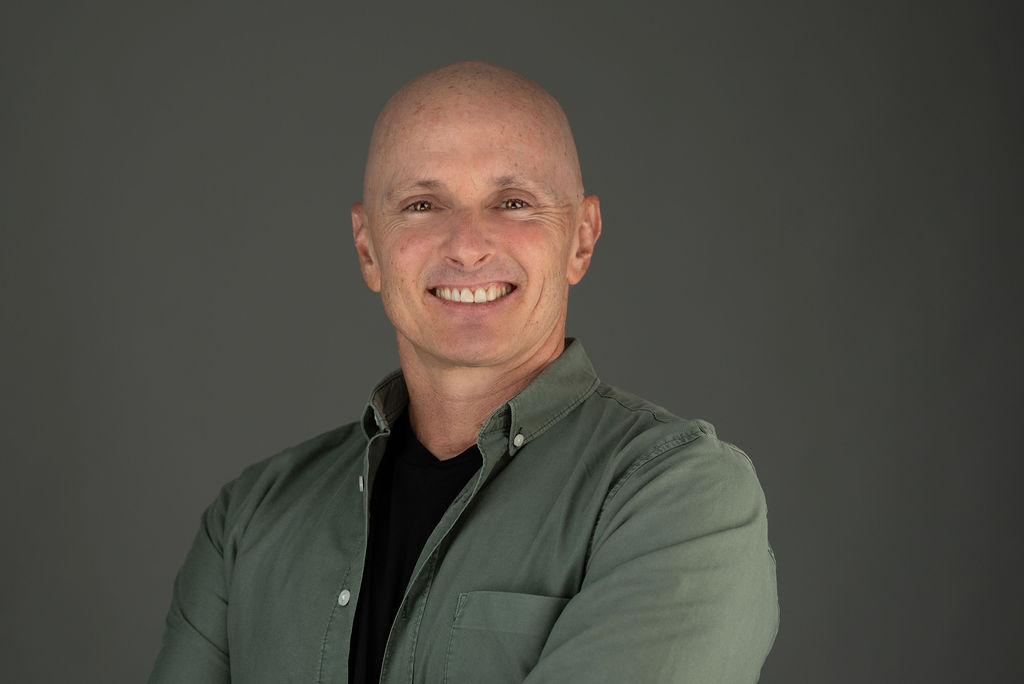