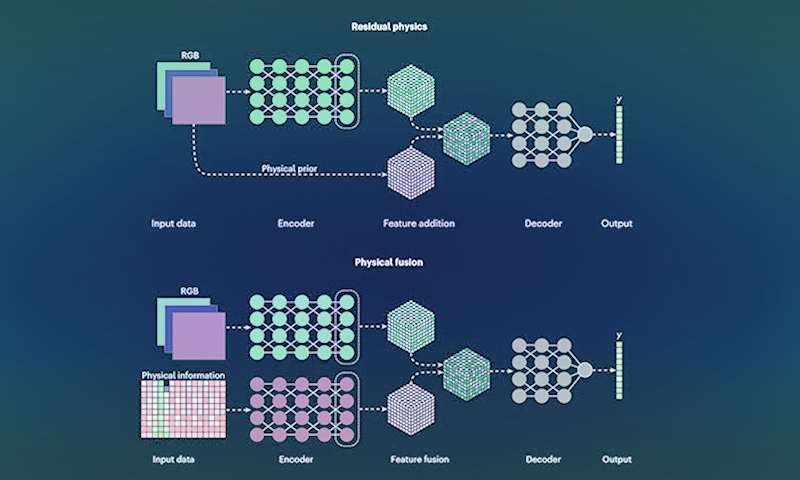
In a revolutionary leap to more intelligent and versatile artificial intelligence (AI), researchers from the UCLA and the Research Laboratory of the United States army have revealed a remarkable approach that merges the fields of physics and megadata. This advanced methodology aims to revolutionize computer vision technologies, raising their capacities to perceive, understand and respond to real -time environments. By Combine awareness -based awareness with data -based techniquesMachines fueled by AI, including autonomous vehicles and precision robots, gain a new level of intelligence and efficiency.
Computer vision serves as a window through which AI perceives and interprets the physical world by decoding complex data and deducting the properties of images. Although these images are intrinsically governed by light and mechanical physics, traditional computer vision techniques are mainly based on automatic learning based on performance optimization. Simultaneously, research-based research has sought to unravel the underlying physical principles behind various challenges of computer vision.
The integration of an understanding of physics in neural networks, imitating the human brain with billions of nodes, was a great challenge. These networks process sets of data from massive images until they acquire understanding of what they “see”. Nevertheless, recent research has identified promising pathways to instill awareness of physics with robust data -based networks.
The study of perceurs of researchers, published in Nature machine intelligenceIntroduces a hybrid methodology that exploits the forces of physics and AI based on data. By combining in -depth knowledge gleaned from data with the real ideas of physics, a new breed of AI emerges – that which has improved capacities and intelligence.
By incorporating physics into AI data sets, researchers label objects with additional information, such as weight and speed of movement, similar to the definition of characters in video games. These increased data allow AI to grasp a deeper understanding of the objects it encounters, allowing better predictions and interactions with its environment.
To permeate cameras with the ability to detect physical properties, researchers offer data execution via network filters. These filters code the physical attributes in images, allowing the AI ​​to perceive and react to the scenes in a closely aligned manner on the laws of physics.
The merger of physics in the AI ​​loss function allows technology to take advantage of the principles of physics while interpreting training data. Consequently, the AI ​​can extract more significant information from observations, leading to better informed decisions and actions.
The success of this double -modality approach has already shown its potential to improve computer vision in a multitude of real world scenarios. For example, machines fueled by AI equipped with this hybrid approach can more precisely follow the movement of objects and generate high -resolution images, even in unnecessary weather conditions.
With continuous progress in this approach to hybrid, AIS based on in -depth learning could even venture on the learning of the fundamental laws of physics independently. This new advancement has immense potential to unlock unforeseen possibilities in various industries, including autonomous vehicles, robotics, health equipment and beyond.
The union of physics and big data in the field of computer vision fueled by AI is a revolutionary stage that brings us closer to human perception and intelligent interactions with the world around us. The search for the UCLA and the Research Laboratory of the United States army opens the way to a new era of hybrid AI, where the combined power of physics and techniques based on data transcends the limits of traditional computer vision. While we are witnessing the transformation of the machines powered by AI, we plan a future where technology is transparently integrated into our physical reality, allowing us to drive in complete safety, to carry out precision tasks and to unlock unequaled progress in human-computer interactions.
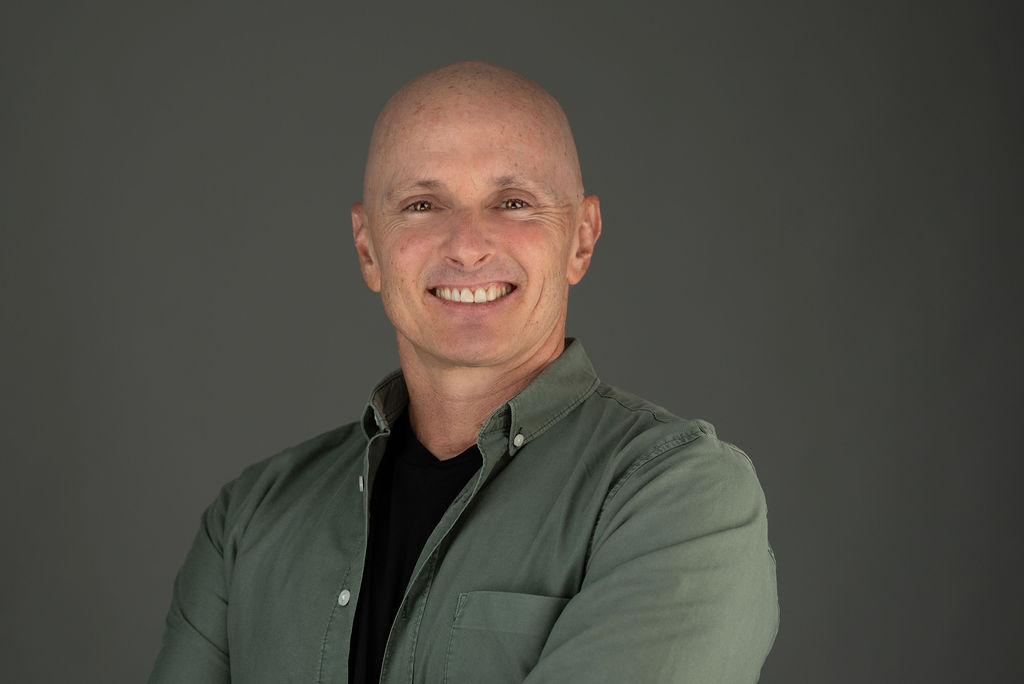