In 2025, AI continues to reshape the way startups build, operate and compete. Google AUT FUTURE: Prospects for startups The report has a complete roadmapBased on the information of infrastructure leaders, startup founders and venture capital partners. The message is pragmatic: AI becomes more accessible, but the reflected application and long -term orientation are more important than speed alone.
The infrastructure is evolving, but startups can abstraction of complexity
AMIN VAHDAT from Google Cloud highlights how the progress of calculation equipment – specialized interconnections, 3D stacked memory and liquid cooling – allow the next generation of IA workloads. These changes in systems are designed to support multimodal long -context models like Gemini 2.0, which offer startups access to increasingly competent tools without the burden of building infrastructure from zero.
This development indirectly benefits startups. Most will not need to manage the equipment, but they should understand how to get upCLOTHE What is available: API based on the cloud with generation (RAG) (RAG) (recovery), and real -time streaming interfaces.
Focus on usefulness, not just novelty
Many contributors point out that the real value of AI is not listed in abstraction but in tangible results. Arvind Jain (Glean) advises founders to approach AI as a way to unlock new product capacities, rather than simply optimizing cost savings. The objective is not to hunt media threshing around agents or automation – it is a question of creating tools that allow users to do something that they could not before.
Startups are also encouraged to be deliberate in the way they design the experiences fueled by AI. Chamath Palihapitiya stresses that the future of the software lies in doing more with less production workflows, and not the multiplication of features. Crystal Huang (GV) stresses that if a product is easy to install, it is just as easy to uninstall. Adherence will come from deep integration into user workflows.
Agent systems: practice on idealism
AI agents remain a promising but developing area. Managers like Harrison Chase (Langchain) and Dylan Fox (Assembly) note that success in this space depends on the resolution of fundamental conviviality problems – politics, persistence of context and hallucination.
Instead of targeting fully autonomous systems, consensus consists in building agents specific to the field with human surveillance and a clear evaluation pipeline. The models are only part of the equation. The definition of success, monitoring the behavior of agents and refining with feedback are the main parts of the development process.
The considerations of the business model count as much as the technology
Startups are advised to move away from the monolithic thought of products and to modular design. Jennifer Li (A16Z) and Jerry Chen (Greylock) both point out that the way an AI product is packaged and sold – based on use, based on value or by seat – can be as strategic as the underlying architecture.
In parallel, the proprietary data remains a basic differentializer. Companies that can generate or access unique data sources will be better positioned to create defensible models and user experiences. Harrison Chase de Langchain encourages teams to prioritize internal evaluation tools from the start, not only to measure performance, but to guide development choices.
AI is a set of tools – not a default company
Several voices warn against access to confusion of the model with lasting differentiation. David Friedberg underlines that the packaging Great language model (LLM) is not a ditch. Instead, the founders should focus on creating what he calls “software factories” – systems that ingest work solutions on business logic and exit, permanently improved by iteration and feedback loops.
Startups are also advised to anchor their strategy in real problems. Whether the application is internal productivity, customer support or automation specific to the field, the strongest use cases tend to come from industries with complex and repetitive tasks and under-optimized workflows.
The AI value chain moves to the application layer
While models and infrastructure continue to commodity, the application layer becomes the place of value creation. APOORV AGRAWAL (Altimeter Capital) considers this as a pivotal change – from the development of the fundamental model and towards AI -Native applications. The recommendation is clear: do not build a model for itself; Create tools that solve something that end users live every day.
There is also a call for intentionality in design. Matthieu Rouif (photoroom) suggests designing experiences that delete friction rather than adding another invitation. The AI must blend into the product, not resume the user interface.
Conclusion
The Google report avoids daring predictions and rather offers anchored advice: startups that carefully integrate AI into specific workflows, align their commercial models with the value delivered and invest in the evaluation of the results will be well positioned for the coming years.
The AI will probably continue to evolve faster than infrastructure, regulations or markets. But by anchoring the development of utility and long -term value, startups can make AI a lasting advantage – not just functionality.
Download the Guide here. Also, don't forget to follow us Twitter And join our Telegram And Linkedin Group. Don't forget to join our 90K + ML Subdreddit.
Asif Razzaq is the CEO of Marktechpost Media Inc .. as a visionary entrepreneur and engineer, AIF undertakes to exploit the potential of artificial intelligence for social good. His most recent company is the launch of an artificial intelligence media platform, Marktechpost, which stands out from its in-depth coverage of automatic learning and in-depth learning news which are both technically solid and easily understandable by a large audience. The platform has more than 2 million monthly views, illustrating its popularity with the public.
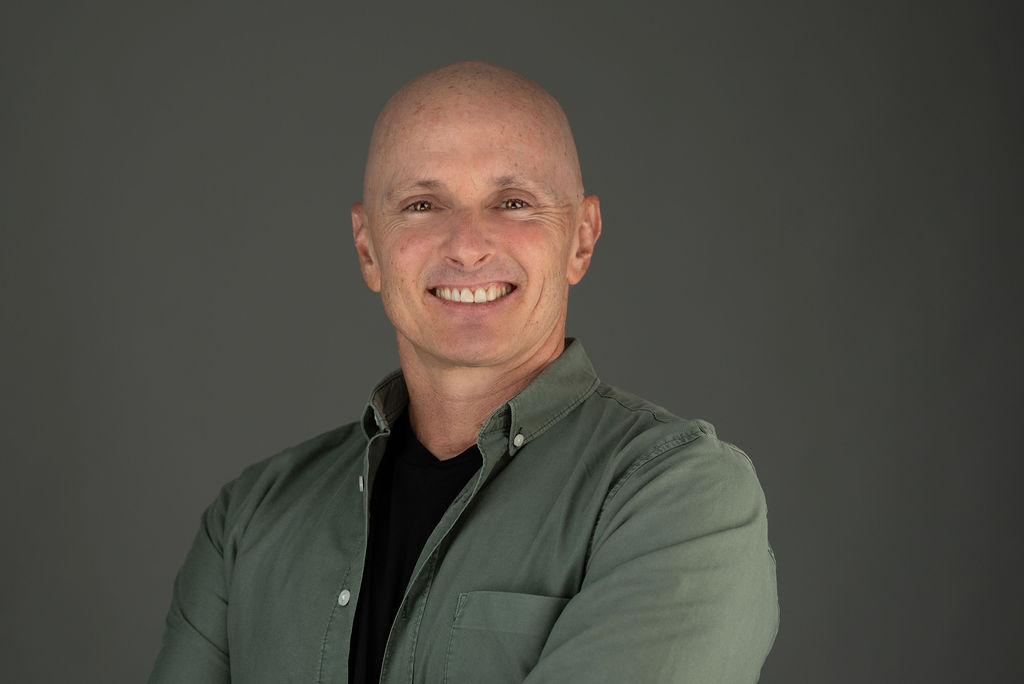