The LLMs have shown progress in reasoning capacities thanks to the learning of strengthening with verifiable rewards (RLVR), which is based on results based on results rather than imitating intermediate reasoning stages. The current RLVR work is faced with critical scope challenges because they are strongly dependent on collections of questions and answers organized manually for training. As the reasoning models advance, the construction of large-scale and high-quality datasets becomes more and more unbearable, similar to the bottlenecks identified in LLM pre-training. In addition, the exclusive dependence of tasks designed by humans can limit the capacity of AI systems to autonomous learning and development, especially since they evolve beyond human intellectual capacities.
Researchers have explored various approaches to improve LLM reasoning capacities. Star was the pioneer of the self-drum using the sampling of experts and sampling of rejection of the responses verified by the results to improve COT reasoning. The O1 model has deployed this large -scale concept, obtaining advanced results, and R1 later became the first open weight model to match or exceed O1 performance by introducing the “zero” setting where RL is applied directly to the basic LLM. In addition, auto-play paradigms have evolved from the first Schmidhuber configurations to two agents to more complex implementations like Alphago and Alphazero. Recent methods such as spin, self-reversed language models, SPC and spag have applied auto-play to language models for alignment and reasoning.
Researchers from the University of Tsinghua, the Beijing Institute for General Artificial Intelligence and the Pennsylvania State University have proposed an RLVR paradigm called Absolute Zero to allow a unique model to generate and resolve tasks in an autonomous way that maximizes its own learning progress without relying on external data. According to this method, the researchers have introduced the absolute zero reasoning (AZR) which evolves self-evolving its training program and its reasoning capacity via a code executor which validates the proposed code reasoning tasks and checks the responses, providing a unified source of verifiable reward to guide open but founded learning. AZR can be implemented effectively on different model scales and remains compatible with various models of models, which suggests a large applicability.
LLMS provide an ideal framework for the implementation of AZR in multitasking learning contexts. During each online deployment iteration in the objective equation of the absolute zero parameter, AZR offers new reasoning tasks based on the type of task and the examples of past self-generation, with an explicit incentive to generate various tasks, then tries to resolve them, receiving comments based on its model responses. AZR uses a code executor as a flexible interface and a verifiable environment, allowing automatic construction, execution and validation of code reasoning tasks. Finally, the AZR algorithm includes the initialization of the buffer, the inputs of the task proposal and the stamp management, the valid construction of tasks, the validation of the solution and the calculation of the estimator advantage in relation to the task relating to the ++ task.
The absolutely zero-coder-7B has achieved advanced performance in the overall average 7B and coding the average categories, exceeding the best previous models of 1.8 absolute percentage points although they are entirely out of distribution for reasoning and code reasoning references. He surpasses the models formed with human data organized by coding experts by 0.3 point of absolute percentage while never accepting such data itself. The scaling analysis reveals that AZR offers larger gains on larger models, 7B and 14B models continue to improve beyond 200 stages of training while 3B model trays. The performance gains excluding distribution increase with the size of the model: +5.7, +10.2 and +13.2 for 3b, 7b and 14b, respectively.
In conclusion, the researchers introduced the absolute zero paradigm to approach the limitations of data in existing RLVR frames. According to this method, the researchers present AZR, which forms models to propose and resolve reasoning tasks linked to the code based on a code executor. However, there is a limitation concerning security management in self-improvement systems. The team observed several cases of reasoning on the COT in strengthening the security of the LLAMA-3.1-8B model, called “UH-OH moments”. The results indicate that if the absolute zero paradigm reduces human intervention needs in the conservation of tasks, continuous monitoring remains necessary to respond to persistent security problems, highlighting a critical orientation for future research.
Discover the Paper,, Model on the embraced face And GitHub page. Also, don't forget to follow us Twitter.
Here is a brief overview of what we build on Marktechpost:
Sajjad Ansari is a last year's first year of the Kharagpur Iit. As a technology enthusiast, he plunges into AI's practical applications by emphasizing the understanding of the impact of AI technologies and their real implications. It aims to articulate complex AI concepts in a clear and accessible way.
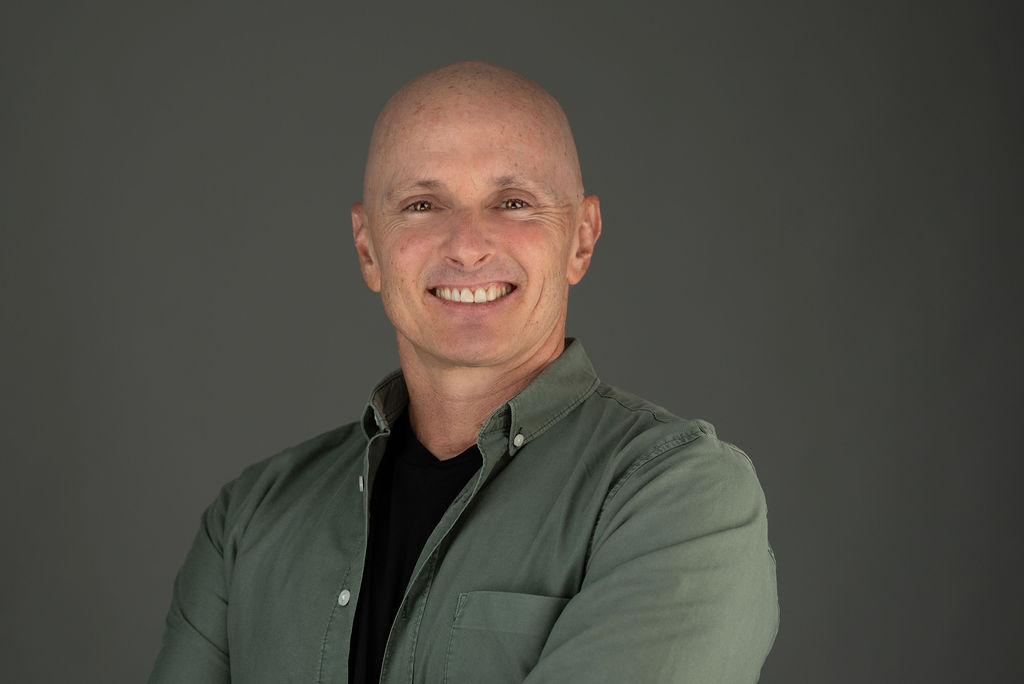