Multimodal mathematical reasoning allows machines to solve problems involving textual information and visual components such as diagrams and figures. This requires combining understanding of language and visual interpretation to give meaning to complex mathematical contexts. These capacities are vital in education, automated tutoring and documents analysis, where problems are often presented with a mixture of text and images.
A major obstacle in this area is the precise and precise lack of alignment between mathematical images and their textual or symbolic representations. Most of the data sets used to form large multimodal models are derived from image legends in natural parameters, which often lack elements essential to mathematical precision. This creates problems for models that are based on these data sources, which makes them unreliable when dealing with geometry, figures or technical diagrams. The performance of a model in mathematical reasoning depends strongly on its ability to interpret and correctly connect these visual details with expressions or mathematical instructions.
In the past, certain approaches have tried to solve this problem by improving visual encoders or using manually manufactured data sets. However, these methods tend to produce a small diversity of images, based on the generation coded by hand or based on models, which limits their applicability. Certain efforts, such as Math-Lava and Mavis, have developed sets of synthetic data and used predefined models or categories. However, they could not dynamically create a wide variety of mathematics visuals. This deficit restricts the learning scope of models and leaves them with more complex or less structured mathematical problems.
Researchers from the Multimedia Laboratory of the Chinese University of Hong Kong and CPII under Innov has introduced a new approach called Mathcoder-VL. This method combines a code vision model named Figcodifier and a synthetic data engine. They have built the Imgcode-8.6m data set using a loop model strategy, which has enabled them to create the largest image code data set to date. In addition, they have developed MM-Mathinstruct-3m, a set of multimodal instructions enriched with newly synthesized images. The Mathcoder-VL model is formed in two stages: mid-training training on IMGCODE-8.6 m to improve the alignment of the visual text and the fine adjustment to MM-MATINTRACT-3M to strengthen reasoning capacities.
The figcodifying cocodifier model works by translating mathematical figures into code which can recreate these figures exactly. This appearance of the image code ensures strict alignment and precision, unlike the sets of data based on legends. The process begins with pairs of image code 119K from Datikz and develops via iterative training using images collected from manuals, K12 datasets and ARXIV articles. The final data set includes 8.6 million code image pairs and covers various mathematical subjects. Figcodifify also supports the rendering based on Python, which adds variety to the generation of images. The system filters low quality data by checking the validity of the code and deleting redundant or useless visuals, resulting in high quality Tikz pairs of 4.3 m and 4.3 m.
Performance assessments show that Mathcoder-VL surpasses several open source models. Version 8B reached a precision of 73.6% on the Mathvista geometry problem solutions, exceeding GPT-4O and Claude 3.5 of 8.9% and 9.2% respectively. He also scored 26.1% on mathematical vision and 46.5% on Mathverse. In the landmarks in Chinese, he reached 51.2% on Gaokao-MM. On the reference of We-Math, he solved two-step problems at 58.6%, surpassing 58.1% of GPT-4O. Its performance on three-step problems reached 52.1%, again exceeding 43.6% of GPT-4O. Compared to his basic model intervl2-8b, he showed 6.1% gains on mathematical vision and 11.6% on Mathvista.
This work clearly defines the problem of insufficient visual-text alignment in multimodal mathematical reasoning and provides an evolutionary and innovative solution. The introduction of synthetic figcodifier and data data allows models to learn precise and various visuals associated with an exact code, considerably increasing their reasoning capacities. Mathcoder-VL represents practical progress in this area, demonstrating how high-quality model design and data can overcome the long-standing limits of mathematical AI.
Discover the Paper And GitHub page. All the merit of this research goes to researchers in this project. Also, don't hesitate to follow us Twitter And don't forget to join our 95K + ML Subdreddit and subscribe to Our newsletter.
Nikhil is an intern consultant at Marktechpost. It pursues a double degree integrated into materials at the Indian Kharagpur Institute of Technology. Nikhil is an IA / ML enthusiast who is still looking for applications in fields like biomaterials and biomedical sciences. With a strong experience in material science, he explores new progress and creates opportunities to contribute.
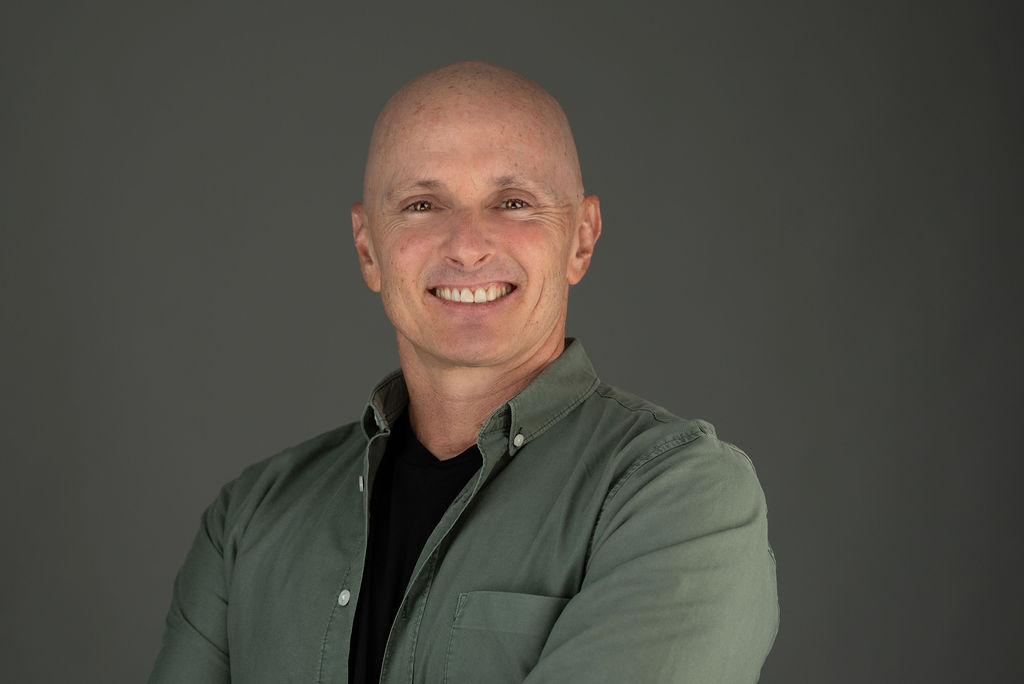