The recent progress of generative models, in particular diffusion models and rectified flows, have revolutionized the creation of visual content with improved output quality and versatility. The integration of human feedback during training is essential to align results with human preferences and aesthetic standards. Current approaches as well as the methods of reflection depend on differentiaiable reward models which introduce the VRAM ineffectiveness for the video generation. DPO variants only get marginal visual improvements. In addition, RL-based methods are faced with challenges, including conflicts between sampling based on the ODEs of rectified flow models and Markov's decision-making formulations, instability during the scale beyond small data sets, and a lack of validation for video generation tasks.
LLMS alignment uses learning to strengthen human feedback (RLHF), which forms reward functions according to comparison data to capture human preferences. Policy gradient methods have proven to be effective but are intensive in calculation and require in -depth adjustment, while direct optimization of policies (DPO) offers profitability but offers lower performance. Deepseek-R1 has recently shown that large-scale RL with specialized reward functions can guide LLM to self-emerging thinking processes. Current approaches include DPO style methods, direct retro-propagation with reward signals as reflected and methods based on policy gradient such as DPOK and DDPO. Production models mainly use the DPO and reflects it due to the instability of policy gradient methods in large -scale applications.
Researchers from Bytedance Seed and the University of Hong Kong proposed Dancegrpo, a unified framework adapting the optimization of policies relating to visual generation paradigms. This solution works transparently through the diffusion models and rectified flows, managing image text tasks, video texts and video image. The framework fits into four foundation models (stable broadcasting, Hunyuanvideo, Flux, Skyreels-I2V) and five reward models covering the image / video aesthetics, text image alignment, the quality of the video movement and binary reward assessments. Dancegrpo surpasses the basic bases up to 181% on key references, including HPS-V2.1, Clip Score, Videoalign and Geneval.
Architecture uses five specialized reward models to optimize the quality of the visual generation:
- Image Aesthetics Quantifies visual appeal using refined models on human nominal data.
- Text image alignment Use Clip to maximize cross-model consistency.
- Video aesthetics quality Extends evaluation to temporal domains using vision language models (VLMS).
- Video movement quality Assesses the realism of the movement through the VLM analysis of physics.
- Binary threshold reward Use a discretization mechanism where the values exceeding a threshold receive 1, others 0, specifically designed to assess the capacity of generative models to learn stiffened steep rewards under optimization based on the threshold.
Dancegrpo shows significant improvements in reward measures for stable broadcast V1.4 with an increase in HPS score from 0.239 to 0.365, and clip from 0.363 to 0.395. Pick-A-PIC and Geneval evaluations confirm the effectiveness of the method, the dancerpo surprising all competing approaches. For Hunyuanvideo-T2i, optimization using the HPS-V2.1 model increases the average reward score from 0.23 to 0.33, showing increased alignment with human aesthetic preferences. With Hunyuanvideo, despite the exclusion of the alignment of the video of the text due to instability, the methodology reaches relative improvements of 56% and 181% in measures of visual quality and movement, respectively. Dancegrpo uses metric of the movement quality of the video reward model, achieving a relative substantial improvement of 91% of this dimension.
In this article, researchers introduced Dancegrpo, a unified framework to improve diffusion models and rectified flows through image text tasks, video text and video image. It addresses the critical limits of previous methods to fill the gap between language and visual methods, by achieving higher performance thanks to effective alignment with human preferences and robust scaling with complex and multi-field parameters. Experiences demonstrate substantial improvements in visual fidelity, the quality of the movement and the image alignment of the text. Future work will explore the extension of GRPO to the multimodal generation, unifying paradigms of optimization through generative AI.
Discover the Paper And Project page. All the merit of this research goes to researchers in this project. Also, don't hesitate to follow us Twitter And don't forget to join our 90K + ML Subdreddit.
Sajjad Ansari is a last year's first year of the Kharagpur Iit. As a technology enthusiast, he plunges into AI's practical applications by emphasizing the understanding of the impact of AI technologies and their real implications. It aims to articulate complex AI concepts in a clear and accessible way.
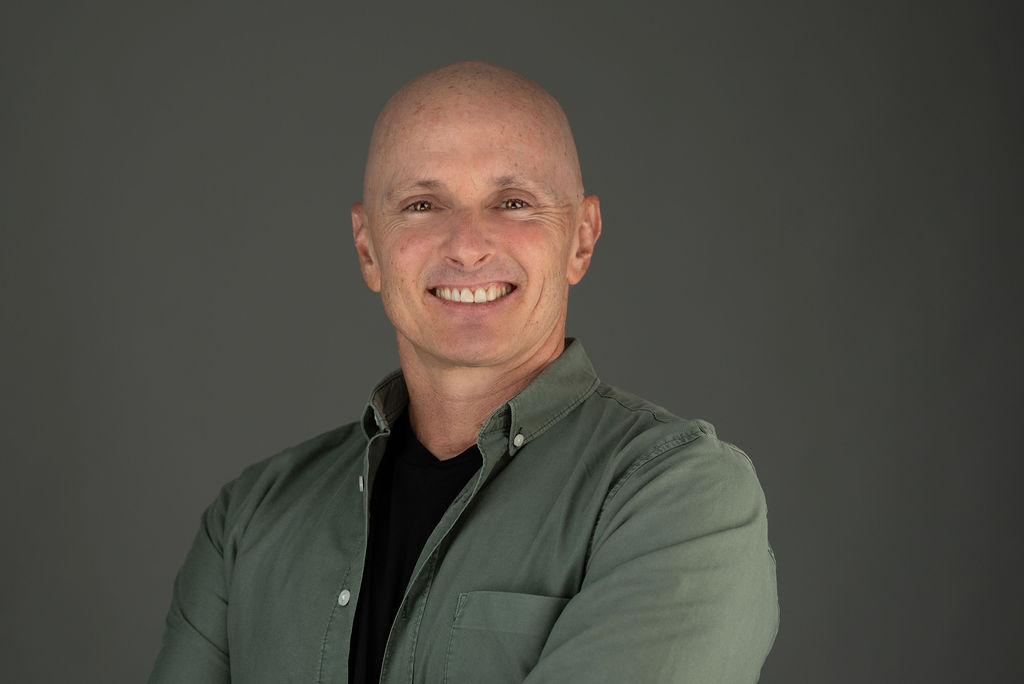