Audio text generation has become a transformative approach to synthesize sound directly from textual prompts, offering practical use in musical production, games and virtual experiences. Under the hood, these models generally use Gaussian flow techniques such as diffusion or rectified flows. These methods model the incremental stages which pass from random noise to structured audio. Although very effective in producing high -quality sound landscapes, slow inference speeds have set a barrier to real -time interactivity. It is particularly limited when creative users expect an instrument -type reactivity of these tools.
Latence is the main problem with these systems. Current audio text models can take several seconds or even a few minutes to generate a few seconds of audio. The basic strangulation neck lies in their inference architecture based on the steps, requiring between 50 and 100 iterations per output. Previous acceleration strategies focus on distillation methods where smaller models are formed under the supervision of larger teachers models to reproduce inferences in several stages in less stages. However, these distillation methods are costly in calculation. They require large -scale storage for intermediate training results or require simultaneous operation of several memory models, which hinders their adoption, especially on mobile or on -board devices. In addition, such methods often sacrifice the diversity of results and introduce over-saturation artifacts.
While some contradictory post-training methods have been tempted to bypass the cost of distillation, their success was limited. Most of the existing implementations are based on partial distillation for initialization or do not go well to complex audio synthesis. In addition, audio applications have seen less contradictory solutions. Tools like Presto integrate contradictory objectives but still depend on teachers' models and training based on CFG for rapid membership, which restricts their generative diversity.
UC San Diego researchers, AI stability and arm have been introduced Post-contract (Arc) opponent after training. This approach avoids the need for teacher models, distillation or advice without classifier. Instead, the arc improves an existing rectified rectified flow generator by integrating two new training objectives: a relativistic opponent loss and a contrastive discriminator loss. They help the generator to produce high fidelity audio in fewer steps while maintaining a strong alignment with text prompts. When associated with the stable open audio framework (SAO), the result was a system capable of generating 12 seconds of stereo audio of 44.1 kHz in just 75 milliseconds on a H100 GPU and approximately 7 seconds on mobile devices.
With the arc methodology, they introduced Open stable audio smallA compact and efficient version of SAO adapted to the environment -related environments. This model contains 497 million parameters and uses an architecture built on a latent diffusion transformer. It consists of three main components: a waveform compression self -dencoder, a t5 -based text integration system for semantic conditioning and a said (diffusion transformer) which works in the latent space of the auto -coder. The Stable Open Small audio can generate stereo audio up to 11 seconds to 44.1 kHz. It is designed to be deployed using the “Stable-Audio-Tools” library and supports the ping-pong sampling, allowing effective generation in a few steps. The model has shown exceptional inference efficiency, reaching generation speeds of less than 7 seconds on a VIVO X200 Pro phone after applying the dynamic int8 dynamic quantification, which also reduced the use of 6.5 GB RAM to 3.6 GB. This makes it particularly viable for creative applications on devices such as mobile audio tools and integrated systems.
The arc training approach consists in replacing the loss of traditional L2 with a contradictory formulation where samples generated and real, associated with identical prompts, are assessed by a discriminator formed to distinguish them. A contrastive objective teaches the discriminator to classify pairs of audio text precise than those incompatible to improve rapid relevance. These paired objectives eliminate the need for CFG while obtaining better rapid grip. In addition, ARC adopts the ping-pong sampling to refine audio production by alternating delating and redesign cycles, reducing the inference stages without compromising quality.
Arc performance has been widely assessed. In objective tests, he obtained an 84.43 FDOPENL3 score, a KLPADST score of 2.24 and a CLAP score of 0.27, indicating balanced quality and semantic precision. Diversity was particularly strong, with a score of conditional diversity (CCD) of 0.41. The real -time factor reached 156.42, reflecting the exceptional generation speed, while the use of GPU memory remained at 4.06 GB practical. Subjectively, ARC obtained a score of 4.4 for diversity, 4.2 for quality and 4.2 for rapid membership in human assessments involving 14 participants. Unlike the models based on distillation like Presto, which obtained a higher note on quality but fell to 2.7 on diversity, Arc presented a more balanced and practical solution.

Several key points to remember from AI stability research on the opponent and contrast (ARC) and stable open Small audio include:
- The arc after training avoids distillation and CFG, based on contastive and contrasting losses.
- The arc generates 12s of the 44.1 kHz stereo audio in 75 ms on H100 and 7 on mobile processors.
- He obtained a conditional diversity score of 0.41 CLAP, the highest among the tested models.
- Subjective scores: 4.4 (diversity), 4.2 (quality) and 4.2 (rapid grip).
- Ping-pong sampling allows inference in a few steps while refining the exit quality.
- Stable Audio Open Small offers 497m settings, supports generation in 8 steps and is compatible with mobile deployments.
- On Vivo X200 Pro, the inference latency increased from 15.3s to 6.6 s with half of the memory.
- Arc and Sao Small provide real -time solutions for music, games and creative tools.
In conclusion, the combination of the CRA after the training and the small open audio extent eliminates dependence on distillation with high intensity of resources and to advice without classifier, allowing researchers to provide a rationalized opponent who accelerates inference without compromising the quality of the exit or rapid membership. The arc allows a rapid, diversified and semantically rich audio synthesis in high -performance and mobile environments. With an open stable audio optimized for a light deployment, this search sets the basics of reactive generative audio tools in daily creative workflows, from professional sound design to real -time applications on EDGE devices.
Discover the Paper, GitHub page And Model on the embraced face. All the merit of this research goes to researchers in this project. Also, don't hesitate to follow us Twitter And don't forget to join our 90K + ML Subdreddit.
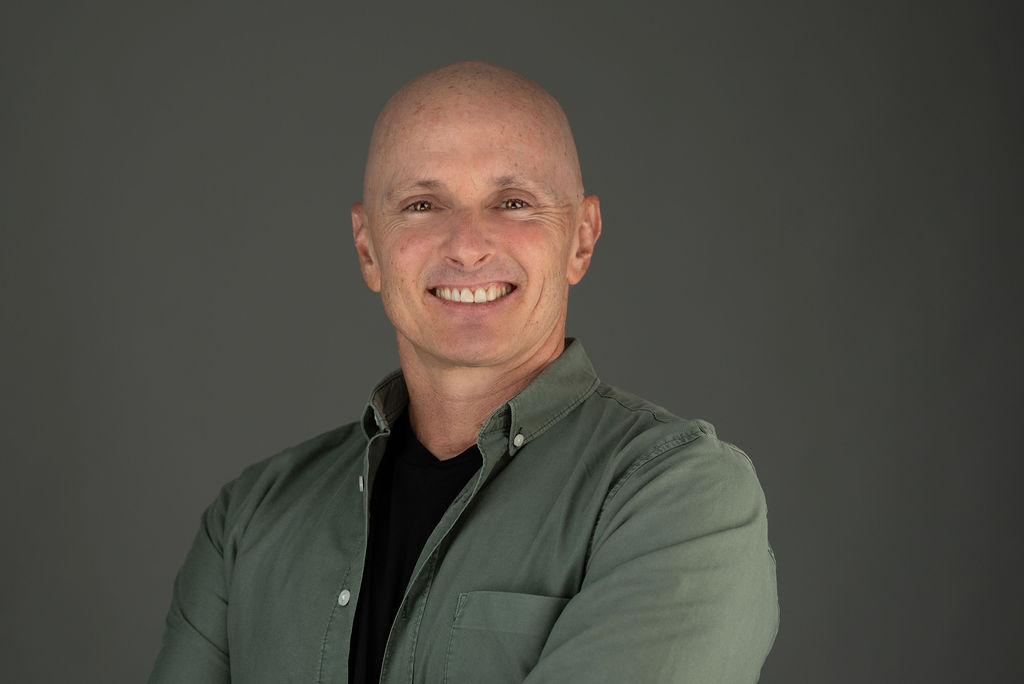