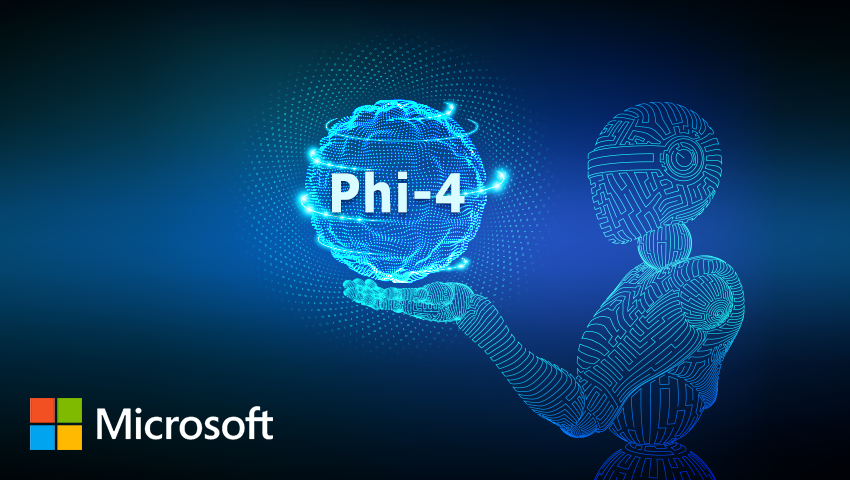
The Phi-4 family is Microsoft's latest advancement in small languages models (SLMS), designed to excel in complex reasoning tasks while maintaining efficiency. The Phi-4 series includes three key models: the seizure of Phi-4, the season of the season of Phi-4 and the seasonal race. The newly published models are built with a clear focus: offer advanced reasoning performance without the infrastructure requests for billions of billions of dollars. They establish an optimal balance between size and performance using advanced techniques such as distillation, strengthening learning and carefully organized data.
During the season of Phi-4 is a 14 billion parameter model with a 32K token context window, formed using high-quality web data and OPENAI O3-Mini prompt. It excels in tasks requiring detailed reasoning and in several steps such as mathematics, coding and solving algorithmic problems.
Phi-4-Seasoning-Plus Bases on this with additional fine adjustment using 1.5 times more tokens and strengthening learning, offering even higher precision and performance of inference time.
In the process of realization of phiWith only 3.8 billion parameters, was formed of a million synthetic mathematics problems generated by Deepseek R1. It targets use cases such as educational tools and mobile applications, proving to be able to solve problems step by step in resource -related environments.
What distinguishes Phi-4 is not only efficiency, but pure capacity. On landmarks like Humaneval + and Math-500:
- Phi-4-Seasoning-Plus surpasses Deepseek-R1 (parameters 671b) on certain tasks, demonstrating that smarter training can beat brute force.
- He also competes with O3-Mini of Openai and exceeds Deepseek-R1-Distill-Lalma-70b on complex reasoning and planning tasks.
- The seasoning of Phi-4-Mini works in a competitive way with much larger models and is even exceeding some in references specific to mathematics.
True to the IA manager responsible for Microsoft, all Phi-4 models are formed with solid safety protocols. Post-training implies a supervised fine adjustment (SFT), direct optimization of preferences (DPO) and learning to strengthen human feedback (RLHF). Microsoft uses public data sets focused on security, aid and equity – ensure large conviviality while minimizing the risks.
The three models are available for free via Face And Foundry Azure Aiallowing researchers, startups and educators to integrate high performance reasoning in their own applications.
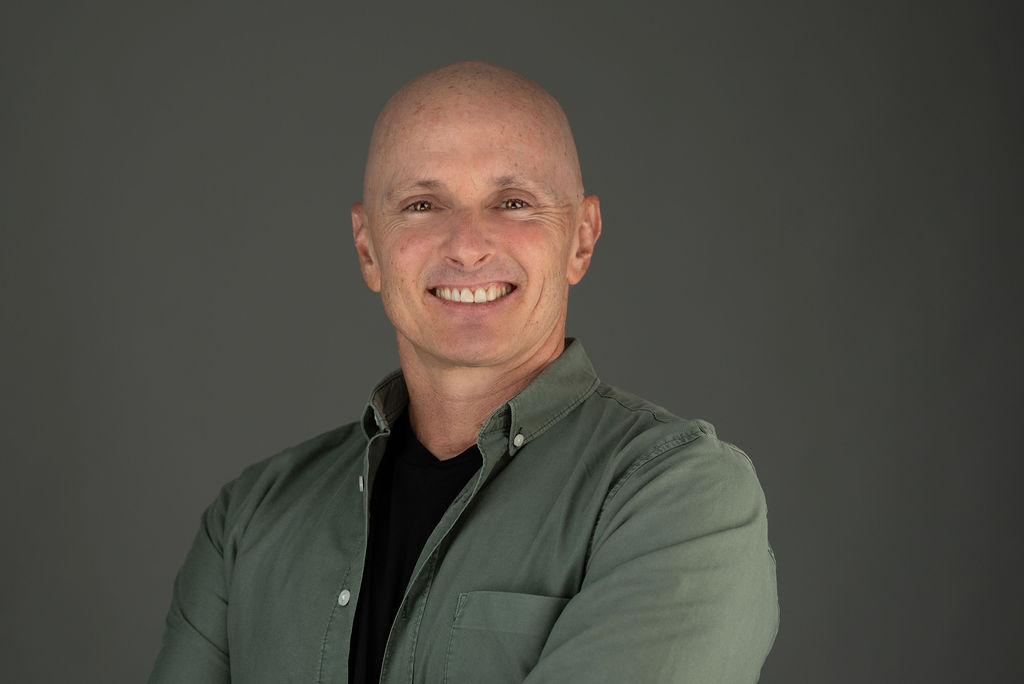