OPENAI launched an End Reinforcement Adjustment (RFT) on its O4-Mini reasoning model, introducing a new powerful technique to adapt the foundation models to specialized tasks. Built on the principles of learning to strengthen, RFT allows organizations to define personalized objectives and to reward functions, allowing fine grain control on how models improve – further beyond the standard supervised fine adjustment offers.
Basically, RFT is designed to help developers bring models to the ideal behavior for real world applications closer to them by teaching them not only what to go out, but why this outing is preferred in a particular field.
What is strengthening strengthening?
The end -up adjustment applies the principles of learning to strengthen the tongue model adjustment model. Rather than counting only on labeled examples, developers provide a grader—A function that assesses and marks the outputs of the model according to the personalized criteria. The model is then formed to optimize against this reward signal, gradually learning to generate responses that line up with desired behavior.
This approach is particularly precious for nuanced or subjective tasks where the truth on the ground is difficult to define. For example, you may not have labeled data for “the best way to formulate a medical explanation”, but you can write a program that assesses clarity, accuracy and completeness – and let the model learn accordingly.
Why O4-Mini?
O4-Mini d'Openai is a compact reasoning model published in April 2025, optimized for text and image entries. It is part of the new generation of Openai of compatible multitasking models and is particularly strong in structured reasoning and chain of thought prompts.
By allowing RFT on O4-Mini, Openai gives developers access to a light but capable base which can be accurately adjusted for specific reasoning tasks, while remaining in effective and sufficiently rapid calculation for real-time applications.
Applied use cases: what developers build with RFT
Several first adopters demonstrated the practical RFT potential on O4-Mini:
- In accordance AI Built a personalized tax analysis model which improved the accuracy of 39% compared to the basic line, using a level -based leveling level to apply the logic of conformity.
- Ambience Healthcare Used RFT to improve the accuracy of medical coding, increasing the performance of the CIM-10 of 12 points on the labels written by doctors.
- HarveyA legal AI startup, refined a model to extract quotes from legal documents with an improvement of 20% of F1, corresponding to GPT-4O on performance to reduced latency.
- Run Trained the model to generate valid striped api extracts, achieving a 12% gain using AST validation and the syntax -based classification.
- MiloA planning assistant, an improved exit quality on the complex calendar invites 25 points.
- Security has increased the accuracy of the moderation of production content from 86% to 90% F1 by applying the compliance of the granular policy through personalized classification functions.
These examples underline the RFT force in the alignment of models with the specific requirements for use cases – that these imply legal reasoning, a medical understanding, a synthesis of the code or the application of policies.
How to use RFT on O4-Mini
The beginning of reinstallation of reinstallation implies four key components:
- Design a classification function: The developers define a Python function that assesses the outputs of the model. This function returns a score from 0 to 1 and can code preferences specific to the task, such as accuracy, format or tone.
- Prepare a data set: A high quality rapid data is essential. OPENAI recommends using various and difficult examples that reflect the target task.
- Launch a training work: Via the API or OpenAi Fineding dashboard, users can launch RFT executions with adjustable configurations and performance monitoring.
- Assess and iterate: The developers monitor the progress of the award, assess the control points and refine the rating logic to maximize performance over time.
Complete documentation and examples are available via OPENAI RFT guide.
Access and price
RFT is currently available for verified organizations. The training costs are billed at $ 100 / hour for an active training time. If an OPENAI hosted model is used to execute the leveler (for example, GPT-4O), the use of tokens for these calls is loaded separately at standard inference rates.
As an incitement, Openai offers a 50% training costs discount for organizations that agree to share their data sets for research and improvement of models.
A technical leap for personalization of the model
The end -up adjustment represents a change in the way we adapt the foundation models to specific needs. Rather than simply reproducing the labeled outputs, RFT allows models to internalize the feedback loops which reflect the objectives and constraints of the real world's applications. For organizations working on complex work flows where precision and alignment are important, this new capacity opens a critical path to a reliable and effective AI deployment.
With RFT now available on the O4-Mini reasoning model, Openai is equivalent to tool developers not only to refine language, but to refine reasoning.
Discover the Detailed documentation here. Also, don't forget to follow us Twitter.
Here is a brief overview of what we build on Marktechpost:
Nikhil is an intern consultant at Marktechpost. It pursues a double degree integrated into materials at the Indian Kharagpur Institute of Technology. Nikhil is an IA / ML enthusiast who is still looking for applications in fields like biomaterials and biomedical sciences. With a strong experience in material science, he explores new progress and creates opportunities to contribute.
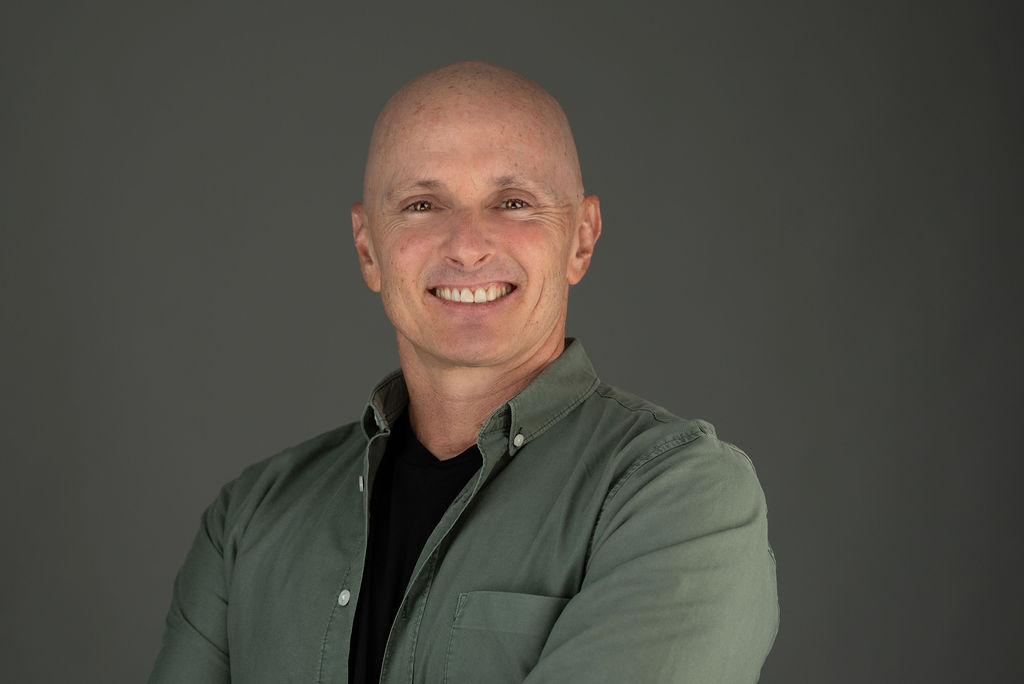