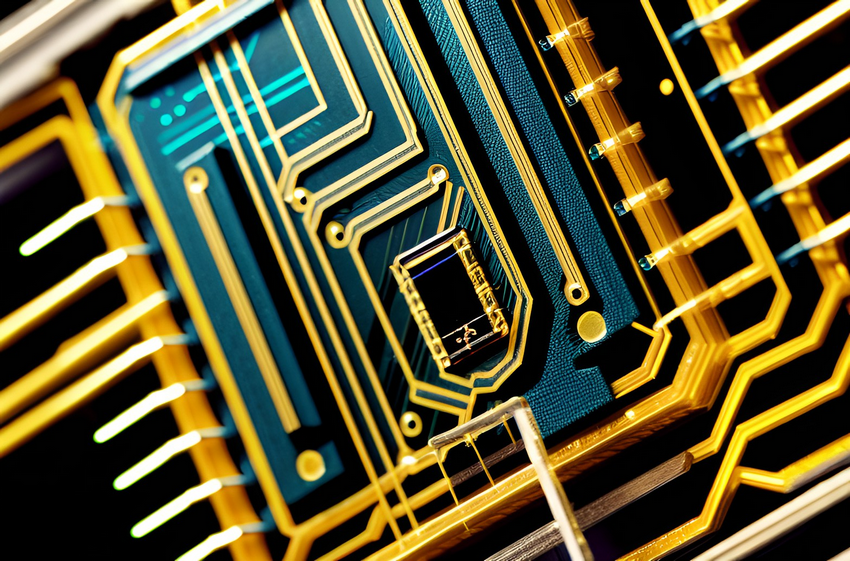
In recent decades, the performance of automatic learning models on various real world problems have improved considerably. However, the formation of these models, as well as their implementation, always requires a huge amount of energy and computer power.
Consequently, engineers around the world are trying to develop alternative material solutions that will more effectively perform artificial intelligence models. This can contribute to their widespread use and increase their sustainability. Some of these solutions are based on Memristors, memory devices that can store information without power consumption.
Memristors are electronic components based on nanodins which limit or regulate the flow of electric current in a circuit, and also register in advance the amount of energy that has crossed it. Because they carry out both the calculation and storage of information, these devices can better perform information processing tasks similar to the human brain.
Researchers from Paris-Saclay-CNRS University, Grenoble-Alpes-Céa-Leti, Hawai.tech, Sorbonne University and Aix-Marseille University-CNRS have developed a prototype of Bayesian machine (that is to say a device based on the theorem of Bayes) using memorists. The system they proposed, which was presented in an article published in Electronic natureIt turned out to be much more energy efficient than the material solutions currently used.
Image of a complete Bayesian machine with a optical microscope
According to Damien Querlioz, one of the researchers who has carried out the study: “Artificial intelligence makes major progress today, but faces a challenge: its considerable energy consumption. The closest to calculation functions are integrated, and we wanted to reproduce this strategy”.
“Until recently, Memristors was emerging technology, and we could not carry out complete systems with them,” said Querlioz. “Now, our team has built a Bayesian machine, a small artificial intelligence with Memristors. The prototype includes 2048 Hafnium oxide memristors and 30080 silicon transistors (Mosfet)”.
A microscopic optical zoomed optical image of the Bayesian machine on one of its 16 Memristor networks. Photo: Damien Querlioz (CNRS / University Paris-Saclay)
The architecture of the machine is designed by writing the law of Bayes in such a way that its implementation is natural according to the principles of distributed memory and stochastic computers, allowing the circuit to operate exclusively by using local memory and a minimal data movement. Researchers use a complementary hybrid process of metal-semi-semi-consumer / Memristor and prove that the largest design of the machine is more energy efficient in the practical task of gestures than the standard implementation of Bayesian inference on a microcontroller. It can recognize certain human gestures using thousands of times less energy than a traditional hardware solution based on a microcontroller. In addition, this Bayesian machine provides an instant on / off and resists unique failures.
“Most research on automatic learning based on Memristor aim to implement learning in depth,” said Querlioz. “This is, of course, an extremely important objective, because in -depth learning is such a success today. However, in -depth learning has certain limits: its results are not explainable, and it does not work well when little data is available. Here, we have chosen to implement Bayesian reasoning, an alternative AI approach which does not provide well the applications of Big Data where the in -depth learning works so well, but in small situations. does not provide fully explained results “.
In the future, the Bayesian machine based on Memristor created by this group of researchers could help improve the energy efficiency of AI models, as well as to potentially inspire the development of other similar solutions. This can be particularly useful for critical safety applications such as medical sensors and industrial security surveillance circuits.
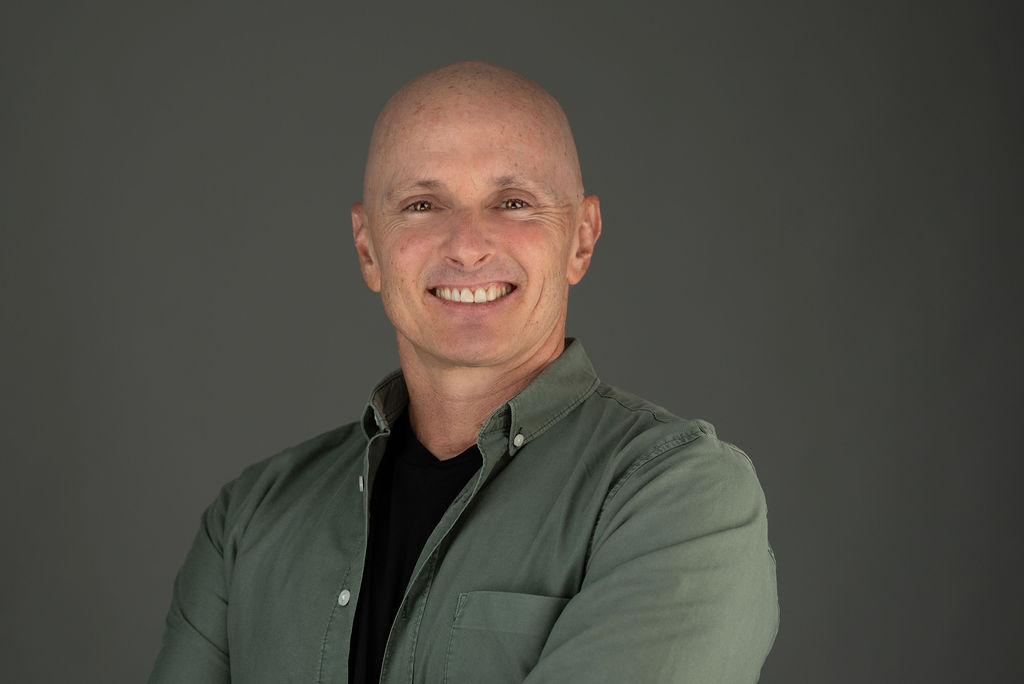