Recent progress in significant reasoning language models (LRLMS), such as Deepseek-R1 and GPT-O1, have considerably improved complex problem solving capacities by extending the duration of COT generation during inference. These models benefit from laws on the test of testing time, allowing richer and more diverse reasoning paths. However, the generation of too long cot sequences leads to an ineffectiveness of calculation and an increased latency, which makes the deployment of real real systems. In addition, excessive reasoning often introduces redundant or non -relevant steps, which can ensure that the models go out of correct answers, ultimately reducing precision. This problem of thinking too much stems from traditional approaches to fine adjustment and learning by traditional strengthening which do not favor dynamic control over the length of reasoning. Research has shown that in many cases, the reasoning could be interrupted earlier, that the authors call points of “pearl reasoning”, without sacrificing accuracy. Identification and stopping at these critical points could considerably improve efficiency while maintaining the performance of the model.
Existing approaches to improve the efficiency of inference is generally distributed in three categories: post-training methods, based on a base and based on output. Post-training techniques involve recycling models with examples of cot of variable length or length rewards, but they are often intensive in calculation and risks. The methods based on prompts adjust the length of the COT by modifying the input prompts according to the difficulty of the task, by achieving more concise reasoning without sacrificing a lot of precision. The methods based on output generally focus on sampling techniques, such as early stop when several outputs converge on the same answer. However, with more recent models like R1, dependence on sampling of N has decreased. Recent work has explored early release strategies, but often require distinct verification models or are only effective in limited contexts. On the other hand, the discussed approach aims to allow models to recognize optimal stop points during their reasoning process, providing a more transparent and generalizable solution.
Researchers from the Institute of Information Engineering, the University of the Chinese Academy of Sciences and Technologies of Huawei proposed deer, a simple and without training method to allow the LRMM to leave dynamically early during reasoning. The deer monitors key transition points, such as the generation of “waiting” token, and invites the model to produce test responses at these moments. If the model shows great confidence, the reasoning is interrupted; Otherwise, it continues. This approach is transparently integrated into existing models, such as Deepseek, and reduces the length of the COT by 31 to 43%, while improving the precision of 1.7 to 5.7% between references, including Math-500, likes 2025 and GPQA Diamond.
The deer method (early dynamic output of reasoning) allows models of important reasoning language to leave the reasoning early by assessing their confidence in test responses to key transition points. He uses three modules: a reasoning transition monitor to detect “reflection switch” signals, an answer to cause a trial conclusion and a confidence assessor to assess whether the reasoning is sufficient. If confidence exceeds a threshold, reasoning stops; Otherwise, it continues. To reduce the latency of the generation of test responses, the deer also uses the decoding parallel to the branch with dynamic management of the cache, thus improving efficiency without sacrificing precision, in particular for tasks such as code generation.
The experiments evaluated the models on four major marks of reasoning: MATH-500, AMC 2025, likes 2025 and GPQA Diamond, as well as the references of Humananeval and Bigcodebench programming. The tests were carried out using Deepseek-R1-Distill-Qwen models of different sizes (1.5b to 32b parameters) under a zero thought chain configuration. The deer has considerably improved performance by reducing the length of the reasoning by 31 to 43% while increasing the accuracy from 1.7 to 5.7% compared to the standard COT. A detailed analysis revealed that deer has corrected more answers through early outings, especially for smaller models and simpler tasks. On programming references, deer have also reduced the duration of the reasoning by more than 60% with a minimum or no loss of precision, demonstrating its robustness on various tasks.
In conclusion, the study validates the idea of using the first outings during the generation of COT by pilot studies. Based on these results, it introduces a dynamic output method without training without training which allows models to stop reasoning once enough information is collected. Tested on various models of models and six major reasoning benchmarks, the method reaches better precision with fewer tokens, effectively balancing efficiency and performance. Unlike traditional approaches that are based on a long cot for complex tasks, this method dynamically monitors confidence to determine when to stop reasoning, thus avoiding unnecessary steps. Experiments show significant reductions in the length of reasoning while increasing global accuracy.
Discover the Paper. Also, don't forget to follow us Twitter And join our Telegram And Linkedin Group. Don't forget to join our 90K + ML Subdreddit.
Sana Hassan, consulting trainee at Marktechpost and double -degree student at Iit Madras, is passionate about the application of technology and AI to meet the challenges of the real world. With a great interest in solving practical problems, it brings a new perspective to the intersection of AI and real life solutions.
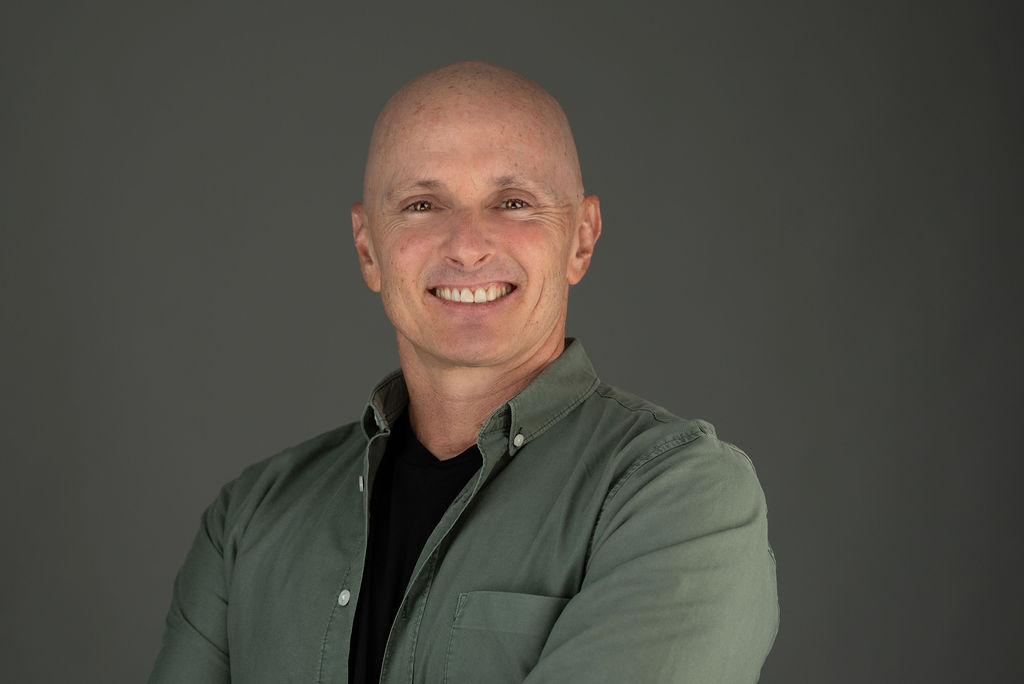