Due to the ambiguity inherent in medical images such as X -rays, radiologists often use words like “can” or “probably” when describing the presence of a certain pathology, such as pneumonia.
But the words that radiologists use to express their level of confidence precisely reflect the frequency at which a particular pathology occurs in patients? A new study shows that when radiologists express the confidence of a certain pathology using a sentence as “very likely”, they tend to be too confident, and vice versa when they express less confidence by using a word as “perhaps”.
Using clinical data, a multidisciplinary team of MIT researchers in collaboration with researchers and clinicians affiliated with the Harvard Medical School has created a framework to quantify how reliable radiologists are when they express a certainty using natural language terms.
They used this approach to provide clear suggestions that help radiologists to choose certainty sentences that would improve the reliability of their clinical reports. They have also shown that the same technique can effectively measure and improve the calibration of large languages models by better aligning words than the models used to express their confidence with the precision of their predictions.
By helping radiologists more precisely describe the probability of certain pathologies in medical images, this new framework could improve the reliability of critical clinical information.
“The words that radiologists use are important. They affect how doctors intervene, in terms of decision -making for the patient. If these practitioners can be more reliable in their reports, patients will be the ultimate beneficiaries, ”explains Peiqi Wang, a student graduated from MIT and principal author of a Document on this research.
He is joined on the newspaper by the main author Polina Golland, professor of electrical engineering and computer science (EECS), a teacher of electrical engineering (EECS), a principal researcher of MIT in computer science and artificial intelligence (CSAIL), and the head of the medical vision group; as well as Barbara D. Lam, clinical stock market at the Beth Israel Deaconess Medical Center; Yingcheng Liu, in Mit Graduate Student; Amenneh Asgari-Targhi, researcher at the Massachusetts General Brigham (MGB); Rameswar Panda, member of research staff at the Mit-ibm Watson Ai Lab; William M. Wells, professor of radiology at the MGB and researcher at CSAIL; And Tina Kapur, assistant professor of radiology at the MGB. Research will be presented at the international conference on representations of learning.
Decode uncertainty in words
A radiologist drafting a report on a pulmonary radiography could say that the image shows “possible” pneumonia, which is an infection that ignites the air bags in the lungs. In this case, a doctor could order a follow -up scanner to confirm the diagnosis.
However, if the radiologist writes that radiography shows “likely” pneumonia, the doctor could start treatment immediately, as by prescribing antibiotics, while ordering additional tests to assess seriousness.
Trying to measure the calibration or reliability of ambiguous terms in natural language as “perhaps” and “probably” presents many challenges, says Wang.
The existing calibration methods generally rest on the trust score provided by an AI model, which represents the estimated probability of the model that its prediction is correct.
For example, a meteorological application could predict 83% of the rain. This model is well calibrated if, in any case where it predicts 83% of the rain, it rains about 83% of the time.
“But humans use natural language, and if we map these sentences in one number, it is not an exact description of the real world. If a person says that an event is” likely “, they do not necessarily think of the exact probability, like 75%,” said Wang.
Rather than trying to map certainty sentences in a single percentage, the researchers' approach treats them as probability distributions. A distribution describes the range of possible values and their probabilities – think of the classic bell curve in statistics.
“This captures more nuances of what every word means,” adds Wang.
Evaluation and improvement of calibration
The researchers have exploited previous work that studied radiologists to obtain probability distributions that correspond to each sentence of diagnostic certainty, ranging from “most likely” to “coherent with”.
For example, as more radiologists think that the expression “consistent with” means that a pathology is present in a medical image, its probability distribution climbs strongly to a high peak, with most of the values grouped around the beach from 90 to 100%.
On the other hand, the expression “can represent” transmits greater uncertainty, leading to a larger distribution in the form of a bell centered on 50%.
Typical methods assess calibration by comparing to what extent the planned probability scores of a model align with the actual number of positive results.
The researchers' approach follows the same general framework but extends it to take into account the fact that certainty sentences represent probability distributions rather than probabilities.
To improve calibration, researchers have formulated and solved an optimization problem that adjusts the frequency to which certain sentences are used to better align trust with reality.
They derived a calibration card which suggests terms of certainty that a radiologist should use to make the relationships more precise for a specific pathology.
“Perhaps, for this set of data, if each time the radiologist said that pneumonia was” present “, they changed the sentence into” probably present “instead, so they would become better calibrated,” Wang explains.
When the researchers used their framework to assess clinical reports, they found that radiologists were generally under-confident during the diagnosis of common conditions such as atelectasis, but too confident with more ambiguous conditions such as infection.
In addition, researchers have evaluated the reliability of language models using their method, offering a more nuanced representation of confidence than the classic methods that are based on trust scores.
“Often these models use sentences like” certainly “. But because they are so confident in their answers, that does not encourage people to verify the accuracy of the declarations themselves, ”adds Wang.
In the future, researchers plan to continue to collaborate with clinicians in the hope of improving diagnostics and treatment. They work to extend their study to include data from abdominal computed tomography.
In addition, they are interested in studying how much radiologists are suggestions to improve calibration and if they can mentally adjust their use of certainty sentences.
“The expression of diagnostic certainty is a crucial aspect of the radiology report, because it influences significant management decisions. This study adopts a new approach to analyze and calibrate How Radiologists express the diagnostic certainty in XORATE X -ray reports, offering feedback on the use of the terms and associated results, “explains ATUL B. Shinagare, associate professor of radiology at the Harvard Medical School, which did not involve this work. “This approach has the potential to improve the precision and communication of radiologists, which will help improve patient care.”
The work was funded, in part, by a Takeda scholarship, the Mit-Ibm Watson AI laboratory, the MIT CSAIL WISTROM program and the MIT Jameel clinic.
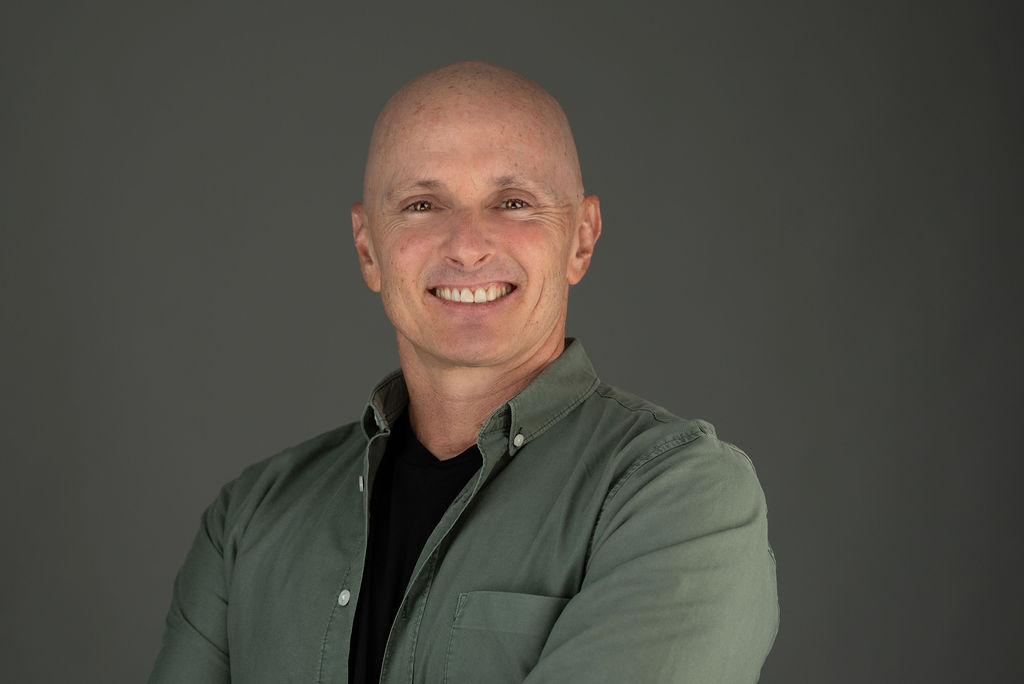