The recent progress of multimodal AI has highlighted a persistent challenge: obtaining solid specialized reasoning capacities while preserving generalization through various tasks. “Slow thoughts” models such as Openai-O1 and Gemini-Thinking have made progress in deliberate analytical reasoning, but often present compromise performance on general visual understanding tasks, with increased trends with visual hallucinations. As the field progresses towards the construction of AI systems for general use, the reconciliation of this compromise remains an essential research problem.
Skywork ai presents Skywork R1V2
Skywork AI published Skywork R1V2, a new generation multimodal reasoning model designed to systematically resolve the compromise of the generalization of reasoning. Drawing on the basics of Skywork R1V, R1V2 presents a learning framework by hybrid strengthening, combining reward model advice with structured signals based on rules. The model bypassing conventional dependence on the distillation of teachers-student by learning directly from multimodal interactions, offering an open and reproducible progression through its release on the embraced face.
Technical approach and innovations
Skywork R1V2 incorporates the optimization of group relative policies (GRPO) alongside a selective sample stamp (SSB) to improve the stability and efficiency of the training. GRPO allows a relative assessment of candidates' responses within the same request group, but convergence problems can reduce effective learning signals. The SSB mechanism addresses this by maintaining an informative samples cover, ensuring continuous access to high value gradients.
In addition, the model adopts an optimization strategy of mixed preferences (MPO), integrating preferences based on the reward model with constraints based on rules. This hybrid optimization allows SkyWork R1V2 to strengthen the quality of the reasoning step by step while maintaining consistency in general perception tasks. A modular training approach, using light adapters between a vision of Vit-6b Inter-6b frozen and a pre-trained language model, preserves the reasoning capacities of the language model while effectively optimizing the cross-modal alignment.
Empirical results and analyzes
Skywork R1V2 demonstrates robust performance on a range of multimodal reasons and references. On text reasoning tasks, the model reaches 78.9% on the AIM2024, 63.6% on Livecodebench, 73.2% on LiveBench, 82.9% on Ifeval and 66.3% on BFCL. These results represent significant improvements compared to Skywork R1V1 and are competitive with significantly larger models, such as Deepseek R1 (671b settings).
In multimodal evaluation, R1V2 reached 73.6% on MMMU, 74.0% on Mathvista, 62.6% on Olympiadbench, 49.0% on Mathvision and 52.0% on MMMU-Pro. The model constantly surpasses the open-end-sized or larger-size base lines, including QWEN2.5-VL-72B and QVQ-PREVIEW-72B, in particular the excellent in tasks that require a resolution of structured problems between visual and textual entries.
Compared to proprietary models, R1V2 demonstrates narrowed performance gaps. It exceeds Claude 3.5 Sonnet and Gemini 2 Flash on critical multimodal references such as MMMU and Mathvista. Above all, hallucination rates were considerably reduced to 8.7% thanks to calibrated strengthening strategies, maintaining factual integrity alongside complex reasoning.
Qualitative evaluations also illustrate the systematic approach to resolution of R1V2 problems, the model demonstrating methodical decomposition and verification behaviors in complex scientific and mathematical tasks, strengthening its alignment with reflective cognitive models.
Conclusion
Skywork R1V2 advances the state of multimodal reasoning through a carefully designed hybrid strengthening learning framework. By approaching the problem of the advantages of the leak with the selective sample stamp and balancing optimization signals thanks to optimization of mixed preferences, the model performs notable improvements in specialized reasoning tasks and general multimodal understanding.
With reference head performance such as 62.6% on Olympiadbench and 73.6% on MMMU, Skywork R1V2 establishes a solid base line in open source. Its design principles and training methodology offer a pragmatic approach to develop robust and effective multimodal AI systems. Future orientations for Skywork IA include improving general visual comprehension capacities while preserving the sophisticated reasoning foundations laid by R1V2.
Discover the Paper And Model on Huggingface. Also, don't forget to follow us Twitter And join our Telegram And Linkedin Group. Don't forget to join our 90K + ML Subdreddit.
Sana Hassan, consulting trainee at Marktechpost and double -degree student at Iit Madras, is passionate about the application of technology and AI to meet the challenges of the real world. With a great interest in solving practical problems, it brings a new perspective to the intersection of AI and real life solutions.
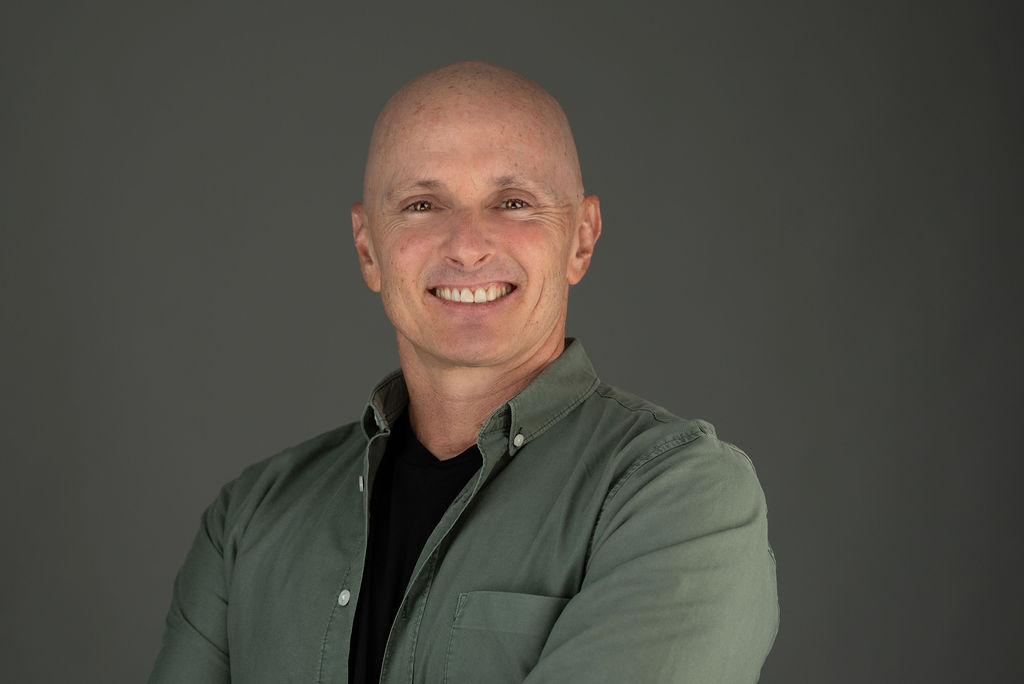