In automatic learningSequence models are designed to process data with a temporal structure, such as language, chronological series or signals. These models follow dependencies through time steps, which allows you to generate coherent outings when learning of the progress of the entries. Neuronal architectures such as recurring neural networks and attention mechanisms manage temporal relations through internal states. The ability of a model to remember and connect the previous inputs to current tasks depends on how it uses its memory mechanisms, which are crucial in determining the effectiveness of the model through real tasks involving sequential data.
One of the persistent challenges of the study of sequence models is to determine how memory is used during calculation. Although the size of the memory of a model – often measured as a state or cache size – is easy to quantify, it does not reveal if memory is used effectively. Two models can have similar memory capacities but very different means to apply this capacity during learning. This difference means that existing assessments do not capture critical nuances in the behavior of the model, leading to ineffectiveness in design and optimization. A more refined metric is necessary to observe the use of memory rather than a simple memory size.
Previous approaches to understand the use of memory in sequence models were based on surface indicators. The visualizations of operators such as attention cards or basic measurements, such as the width of the model and the capacity of cache, have provided an overview. However, these methods are limited because they often only apply to narrow classes of models or do not take into account important architectural characteristics such as causal masking. In addition, techniques such as spectral analysis are hampered by hypotheses that do not hold all models, in particular those with dynamic or varying structures. Consequently, they are unable to guide how models can be optimized or compressed without degrading performance.
Researchers from Liquid AI, the University of Tokyo, Riken and the University of Stanford have introduced an effective state size (ESS) measure to measure the amount of memory of a really used model. ESS is developed using principles of the theory of signal control and processing, and it targets a general class of models which include invariant linear operators and varying entry. These cover a range of structures such as attention variants, convolutional layers and recurrence mechanisms. ESS works by analyzing the rank of sub-lambers within the operator, by specifically focusing on how the inputs contribute to current outputs, providing a measurable means of assessing the use of memory.
The calculation of the SSE is based on the landing in the analysis of the rank of operators' sub-lambers which connect the input segments prior to subsequent outputs. Two variants have been developed: tolerance-ESS, which uses a threshold defined by the user on singular values, and Entropy-ESS, which uses normalized spectral entropy for a more adaptive view. The two methods are designed to manage practical calculation problems and are evolving on multilayer models. The ESS can be calculated by channel and sequence index and aggregated as medium or total ESS for a complete analysis. Researchers point out that ESS is an lower limit of the required memory and can reflect dynamic models in model learning.
Empirical evaluation has confirmed that the SSE is closely correlated with the performance between various tasks. In multi-requiring associative recall tasks (MQAR), won normalized by the number of key values ​​(ESS / KV) has shown a stronger correlation with the accuracy of the model than the theoretical size of state size (TSS / KV). For example, high ESS models have constantly reached higher precision. The study also revealed two modes of failure in the use of the memory of the model: state saturation, where the ESs are almost equal to TSS, and the collapse of the State, where the ESS remains underused. In addition, the SSE has been successfully applied to the compression of the model via distillation. A higher ESS in teacher models led to greater loss when compressing smaller models, showing the usefulness of ESS to predict compressibility. He also followed the way in which the use of the memory modulated by the end of the sequence tokens in large -language models like Falcon Mamba 7B.
The study describes a precise and effective approach to resolve the difference between the theoretical size of memory and the real use of memory in sequence models. Thanks to the development of the SSE, the researchers offer a robust metric that brings the clarity of the evaluation and optimization of the model. It opens the way to the design of more effective sequence models and makes it possible to use ESS in the regularization, initialization and compression strategies of models based on clear and quantifiable memory behavior.
Discover the Paper. All the merit of this research goes to researchers in this project. Also, don't hesitate to follow us Twitter And don't forget to join our 90K + ML Subdreddit.
Here is a brief overview of what we build on Marktechpost:
Nikhil is an intern consultant at Marktechpost. It pursues a double degree integrated into materials at the Indian Kharagpur Institute of Technology. Nikhil is an IA / ML enthusiast who is still looking for applications in fields like biomaterials and biomedical sciences. With a strong experience in material science, he explores new progress and creates opportunities to contribute.
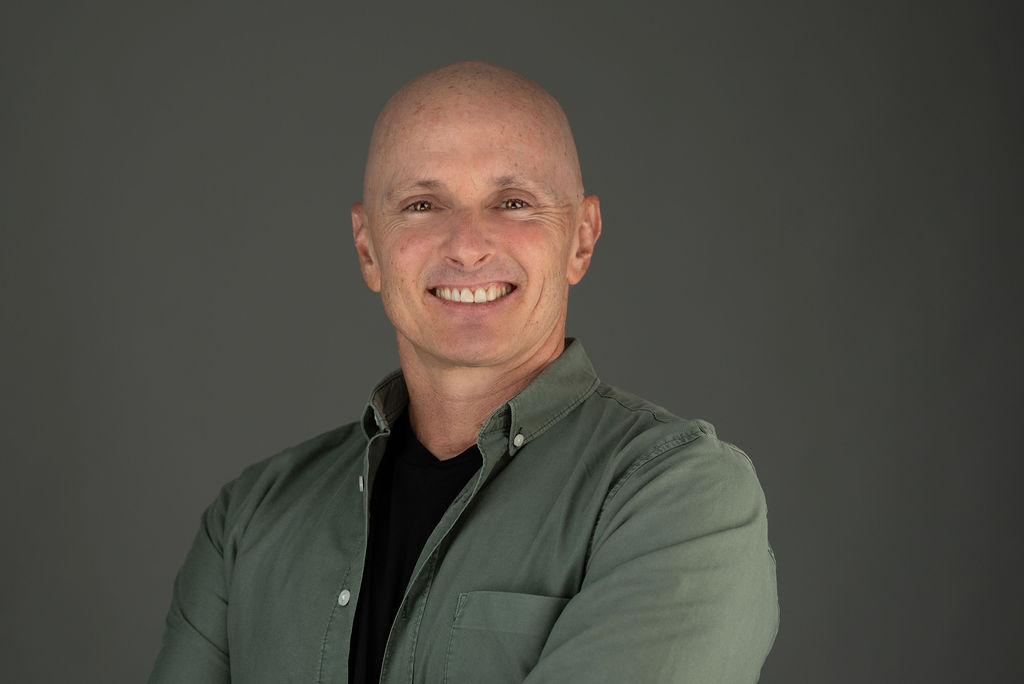