AI models have made remarkable progress in the generation of speech, music and other forms of audio content, widening possibilities through communication, entertainment and human-computer interaction. The ability to create a human audio through deep generative models is no longer a futuristic ambition but a tangible reality that has an impact on industries today. However, as these models become more sophisticated, the need for rigorous, scalable and objective evaluation systems becomes critical. The assessment of the quality of the generated audio is complex because it implies not only to measure the precision of the signal, but also to assess perceptual aspects such as natural, emotion, identity of speakers and musical creativity. Traditional evaluation practices, such as human subjective assessments, are long, expensive and subject to psychological biases, making automated audio evaluation methods a need to advance research and applications.
A persistent challenge in the assessment of automated audio lies in the diversity and inconsistency of existing methods. Human evaluations, although they are a ordeal, suffer from prejudices such as the equalization effects of the distribution area and require significant knowledge of labor and experts, in particular in nuanced fields such as the synthesis of singing or emotional expression. The automatic measures have filled this gap, but they vary considerably depending on the application scenario, such as the improvement of speech, the synthesis of speech or the generation of music. In addition, there is no set of universally adopted metrics or a standardized framework, leading to dispersed efforts and incomparable results on different systems. Without unified evaluation practices, it becomes more and more difficult to compare the performance of audio generation models and to follow real progress in the field.
Existing tools and methods cover each part of the problem. Tool boxes like ESPNET and evaluation modules offer sheets, but focus strongly on speaking treatment, offering limited coverage for music or mixed audio tasks. AudiOLDM-Eval, stable-audio-metric and Sony Audio-metrics try wider audio assessments but always suffer from a fragmented metric support and inflexible configurations. Measures such as the average opinion score (MOS), the PESQ (perceptual evaluation of the quality of the word), the SI-SNR (signal / noise ratio on a scale) and the Audio Fréchet (FAD) are widely used; However, most of the tools implement only a handful of these measures. In addition, dependence on external references, whether corresponding or non -corresponding audio, text transcriptions or visual indices, varies considerably between the tools. The centralization and normalization of these evaluations in a flexible and scalable toolbox have so far remained an unmet need.
Researchers from Carnegie Mellon University, Microsoft, the University of Indiana, the Nanyang Technological University, the University of Rochester, the University of Renmin of China, the University of Shanghai Jiaotong and the AI of Sony VersaA new evaluation toolbox. Versa stands out by offering a modular toolbox based on Python which incorporates 65 evaluation measures, leading to 729 configurable metric variants. It supports the evaluation of speech, audio and music in a single framework, a functionality that no previous toolbox has obtained overall. Versa also emphasizes flexible configuration and strict dependence control, allowing easy adaptation to different evaluation needs without incurring software conflicts. Released publicly via Github, Versa aims to become a fundamental tool to compare sound generation tasks, thus making a significant contribution to research and engineering communities.
The Versa system is organized around two basic scripts: “scorers.py” and “aggregate_result.py”. The “scorers.py” manages the real calculation of the measures, while “aggregate_result.py” consolidates the metric results in complete evaluation reports. The input and output interfaces are designed to support a range of formats, including PCM, FLAC, MP3 and Kaldi-Gark, welcoming various file organizations for WAV.SCP mappings with simple directories. The metrics are checked via unified YAML style configuration files, allowing users to select measurements in a main list (general.yaml) or to create specialized configurations for individual measurements (for example, MCD_F0.YAML for the evaluation of MEL CEPSTRAL distortion). To further simplify conviviality, Versa ensures minimum default dependencies while providing optional installation scripts for measures that require additional packages. Local forks of external evaluation libraries are incorporated, guaranteeing the strictly locking flexibility, improving both the conviviality and the robustness of the system.
When he compared existing solutions, Versa surpasses them considerably. It supports 22 independent measures which do not require reference audio, 25 dependent measures based on the corresponding references, 11 measures which are based on non -corresponding references and five distributional measures to assess the generative models. For example, independent measures such as SI-SNR and VAD (detection of vocal activity) are taken care of, alongside dependent measures such as PESQ and STOI (short-term objective intelligibility). The toolbox covers 54 measures applicable to speech tasks, 22 at the general audio and 22 to the music generation, offering unprecedented flexibility. In particular, Versa supports the evaluation using external resources, such as textual legends and visual indices, which makes it suitable for multimodal generative evaluation scenarios. Compared to other tool boxes, such as audiocrat (which only supports six metrics) or the amphion (15 measurements), Versa offers unrivaled scale and depth.
Research shows that Versa allows a coherent comparative analysis by minimizing subjective variability, improving comparability by providing a unified metric set and improving research efficiency by consolidating various evaluation methods in a single platform. By offering more than 700 metric variants simply by configuration adjustments, researchers no longer have to reconstruct different evaluation methods from several fragmented tools. This consistency in evaluation promotes reproducibility and fair comparisons, which are both essential to follow the progress of generative sound technologies.
Several key dishes of research on Versa include:
- Versa provides 65 measures and 729 metric variations to assess speech, audio and music.
- It supports various file formats, including PCM, FLAC, MP3 and Kaldi-Bar.
- The toolbox covers 54 measures applicable to speech, 22 at audio and 22 with music generation tasks.
- Two basic scripts, “scorers.py” and “aggregate_result.py”, simplify the process of evaluation and generation of reports.
- Versa offers strict but flexible dependence control, minimizing installation conflicts.
- It supports the evaluation using corresponding and non -corresponding audio references, text transcriptions and visual indices.
- Compared to 16 measures in ESPNET and 15 in amphion, the 65 metrics of Versa represent major progress.
- Released publicly, it aims to become a universal standard to assess the sound generation.
- Flexibility to modify configuration files allows users to generate up to 729 separate evaluation configurations.
- The toolbox addresses biases and ineffectiveness in subjective human assessments thanks to reliable automated assessments.
Discover the Paper, Demo on the embraced face And GitHub page. Also, don't forget to follow us Twitter And join our Telegram And Linkedin Group. Don't forget to join our 90K + ML Subdreddit.
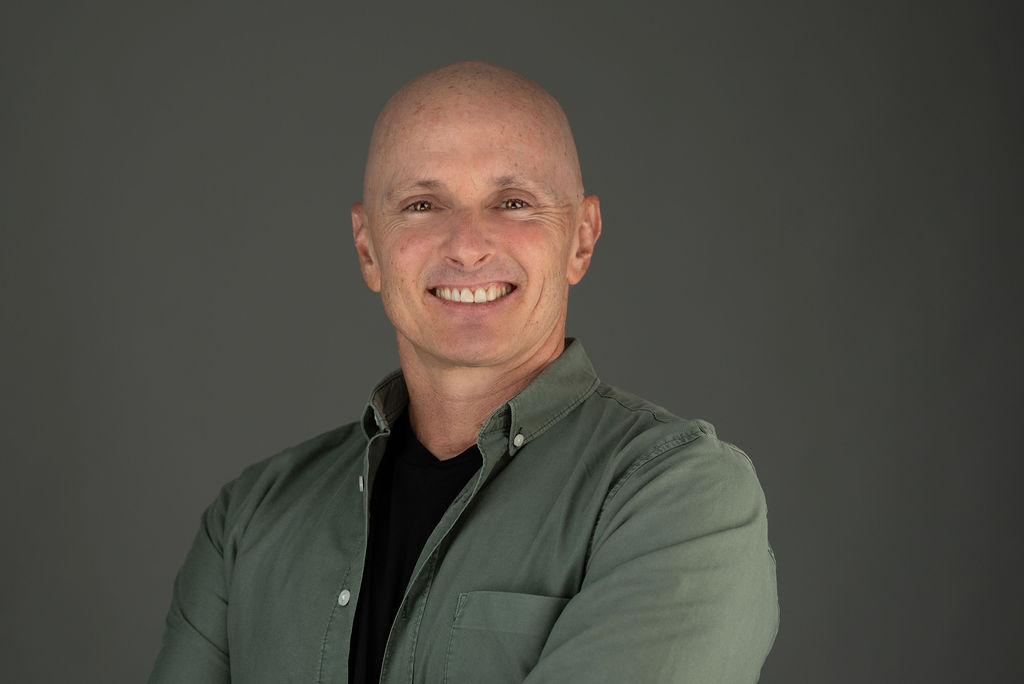