The realization of solid reasoning and in several stages in LMS remains a major challenge, despite notable progress in the performance of general tasks. Such reasoning is crucial for complex fields of problem solving, such as scientific research and strategic planning. Traditionally, improving reasoning skills involves a supervised fine setting (SFT), where models learn by imitating demonstrations of reasoning step by step from more advanced models, such as O1. Although effective, this method strongly depends on the availability of high quality reasoning traces, which are expensive and promote the risk of shallow mimicry on a real logical exploration. RL offers an alternative by allowing models to learn reward signals directly, encouraging the exploration of wider reasoning. However, RL approaches are often heavy with resources and complexes, which raises the question of how to build models compatible with reasoning in a profitable manner.
After the publication of strong models like O1-PREVIEW, several open source efforts such as Still, Sky-T1, Simplerl, Prime and Deepscaler explored effective strategies to reproduce or exceed the reasoning capacities of O1. The techniques include learning light imitation, adjustment of evolutionary instructions and simplified RL methods. Meanwhile, more recent innovations, such as the relative optimization of group policies (GRPO), improve the effectiveness of RL training by eliminating the need for separate value networks, as shown by models as Deepseek-R1. To further reduce training costs, researchers also study low-rank adaptation methods (LORA), which update only a small subset of model parameters, maintaining modularity while preserving reasoning capacity. This approach allows an effective fine adjustment without the requirements for calculating updates with a complete parameter.
Researchers from the University of South California introduce Tina, a family of compact reasoning models that reach solid performance with a minimum cost. Using RL improved by Lora on a 1.5B parameter model, TINA models surpasses or correspond to advanced models to a fraction of calculation expenses. Their best model improves reasoning performance by more than 20% and reached 43.33% of @ 1 passes on AIME24, with a post-training cost of only $ 9. By taking advantage of Lora's efficiency to adapt reasoning formats while preserving basic knowledge, Tina highlights a very accessible and profitable approach, with all the entirely open resources.
Tina is a family of tiny reasoning models built by the Deepseek-R1-Distill-qwen-1.5B model using Lora during learning to strengthen a GRPO style approach. The framework emphasizes minimalism – dull models, small parameters updates and a low material and budget imprint. The TINA models have been formed using public data sets and configurations replicated from models like Still-3, Deepscaler and Open-RS. The training used the OpenR1 code base, a minimum hyperparameter setting and only two NVIDIA L40S GPU, sometimes RTX 6000 ADA GPUS. The training and evaluation costs were low, on average under a budget of $ 100 with experience, making Tina a platform very accessible for research reasoning.
To guarantee fair comparisons, the authors have reassessed the basic reasoning models using a configuration consistent with the Lightval framework and the VLLM engine, thus eliminating the variations introduced by previous studies. Six benchmarks of reasoning, which likes it 24/25, AMC 23, Math 500, GPQA and Minerva, were used. They then evaluated the TINA models – of the LORA basic versions of the basic models – according to the TINA models, outclassed their counterparts in full parameter despite the use of minimum training (19–57% of an era). Other removal studies have revealed that smaller and high -quality data sets, appropriate learning rates, moderate LORA ranks and a meticulous choice of RL algorithm have had a significant impact on performance, confirming the efficiency and robustness of their LARA -based reasoning approach.
In conclusion, Tina, a series of light reasoning models that obtain solid performance using minimum calculation resources. By applying Lora during RL to a basic model of 1.5 parameter B, they reach competitive reasoning capacities with larger advanced models at a post-training cost of only $ 9. TINA models show an improvement of 20% of reasoning and 43.33% precision @ 1 on precision on AIME24. While having an impressive efficiency on performance costs, limits remain, including the smallest model scale, the limited diversity of reasoning tasks and minimal hyperparameter adjustment. All codes, newspapers and model control points are open to promote accessible research and more in -depth exploration.
Discover the Paper And GitHub page. Also, don't forget to follow us Twitter And join our Telegram And Linkedin Group. Don't forget to join our 90K + ML Subdreddit.
Sana Hassan, consulting trainee at Marktechpost and double -degree student at Iit Madras, is passionate about the application of technology and AI to meet the challenges of the real world. With a great interest in solving practical problems, it brings a new perspective to the intersection of AI and real life solutions.
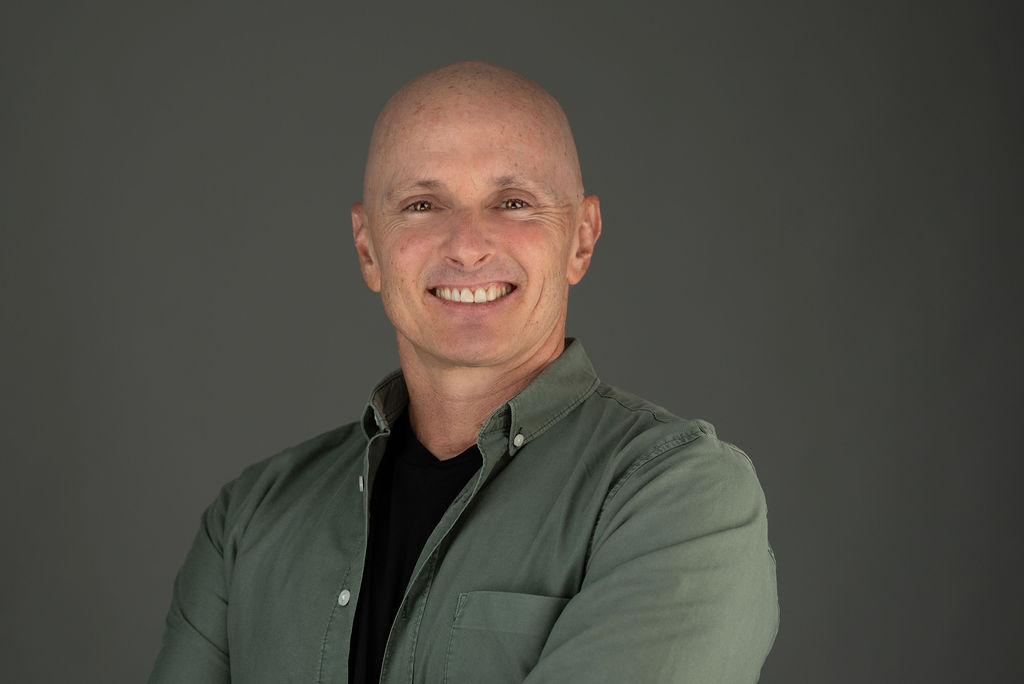