With an increasing demand for AI systems that can manage tasks involving logic in several stages, mathematical evidence and software development, researchers have turned their attention to improving the models of models. This capacity, formerly considered exclusive to human intelligence, is now actively pursued in smaller models to make them more effective and largely deployable. While the reasoning -based tasks continue to develop in relevance, encompassing the school resolution of problems, automated theorem, the design of algorithms and the debugging of complex software, linguistic models should become more than conversational agents for general use. They are encouraged to become problem -specific problems with the fields that can help professionals and researchers.
A challenge in the construction of reasoning -oriented models is to obtain solid and simultaneous mathematics and programming performance while maintaining a relatively small model size. The most competitive results in these areas are obtained by models with around 32 billion or more parameters. These large models are often used because the smallest finds it difficult to generalize and optimize rewards in strengthening learning tasks, in particular with regard to the problem -based problem solving. A sparse reward feedback, high -quality limited data and low basic model architecture make the development of compact but powerful models difficult. In addition, the data used to train these models is not always organized with reasoning in mind, often causing ineffectiveness of training and limited gains in problem solving capacities.
To meet the challenges of reasoning, several models, including OPNAI's O series, Deepseek R1 and Claude 3.7, were introduced, taking advantage of the counts of massive parameters and complex reinforcement learning strategies. These models use techniques such as step -by -step planning and backwards to improve reasoning, especially in algorithmic thinking and mathematics tasks. However, they strongly depend on post-training steps and underestimate the importance of high-quality pre-training data. Many are also based on reward systems based on fixed models which are inclined to reward hacking. Code generation references often reveal that these models work inconsistently in difficult tasks due to shallow pre-training foundations and modeling the ineffective reward signal during the fine adjustment.
A Xiaomi research team introduced the Mimo-7b Family of linguistic models with a targeted approach to overcome these obstacles. Innovation lies in the treatment of pre-training and post-training as a phases that are just as critical to develop reasoning capacities. The basic model, Mimo-7b-Base, was formed from zero using a data set comprising 25 billions of tokens. This data set was built with a three -step mixing strategy which has gradually increased the share of mathematical and programming content. An additional prediction of prediction (MTP) of several token was introduced during pre-training to improve both performance and speed of inference. For post-training, the team has developed an organized data set of 130,000 mathematics and verifiable programming problems, each tagged with difficulty scores. The learning of strengthening was then applied using a reward focused on difficulty, allowing more nuanced and effective feedback during training. This resulted in two major variants: Mimo-7b-RL and Mimo-7b-Rl-Zero.
The pre-training methodology started with the extraction of reasoning content from web pages, academic articles and books using a personalized HTML extraction tool designed to preserve mathematical equations and code extracts. Unlike generic pipelines, this extractor has retained essential structural elements for problem solving areas. The team then improved PDF analysis tools to precisely interpret the scientific and programmed content. To avoid duplication of data, the overall deduction was applied using techniques based on URL and Minhash. The training corpus was filtered using Small language models Adjusted to mark the quality of the content, replacing obsolete heuristic filters which often deleted examples of precious reasoning. High quality synthetic reasoning data has also been generated from advanced and added models to the final training stage. This three -step approach led to a mixture of final training comprising 70% of mathematical data and code in step two and 10% of the synthetic content in the third step. The maximum context length was extended from 8 192 to 32,768 tokens, ensuring that the model could manage long -term reasoning problems.
In the strengthening learning phase, the search team designed a transparent deployment engine to accelerate training and validation. This infrastructure has incorporated the asynchronous reward calculation and early termination mechanisms to reduce the inactivity time of the GPU, which leads to training 2.29 times faster and 1.96 times faster validation. The model of the model was optimized using a fine grain awards derived from the difficulty of test cases, solving the problem of sparse reward in the programming of landmarks. Data reorganization techniques have been introduced to maintain training stability and increase the deployment sampling efficiency. These strategies have collectively allowed Mimo-7B variants to learn effectively, even from cold implementation states where no pre-regulated initialization is available.
The performance assessment revealed that the Mimo-7B base has obtained a 75.2 score on the hard task (BBH), exceeding other 7B open source models. He also worked well on Supergpqa, which includes reasons of reasoning in graduates. The Mimo-7b-RL post-formmed marked 55.4 on the reference likes 2025, exceeding the O1-Mini of Openai of 4.7 points. On code generation tasks, he outperformed much larger models like Deepseek-R1-Zero-32B and QWEN2.5-32B-RL-ZERO on LiveCodebench V5 and V6. These results show that a properly optimized 7B model can compete or even surpass the models with more than four times the number of parameters.
The MIMO-7B project serves as a concrete demonstration of how pre-training, data quality and strengthening learning infrastructure contribute to the final reasoning capacity of a language model. By rethinking the data extraction pipeline to the reward calculation, the Xiaomi research team obtained compact but powerful models adapted to actual applications in mathematics, coding and logic. Their approach highlights the unexploited potential of small models and calls into question the hypothesis that size alone determines intelligence or versatility.
The main dishes to remember from research on Mimo-7b:
- Mimo-7B was formed on a massive data set of 25 billions of tokens, targeting reasoning tasks thanks to the use of structured data mixtures.
- 130,000 mathematics and code problems were used in RL training, each annotated with difficulty scores to allow effective formatting of rewards.
- Mathematics and coding content increased in three stages to 70%, followed by 10% of synthetic problem solving data.
- A transparent deployment engine increased the RL training speed by 2.29 times and the validation of 1.96 times.
- Mimo-7B-RL reached 55.4 on AIM 2025, surpassing the O1-Mini OPNAI of 4.7 points.
- Mimo-7B models are accessible to the public and include all control points: base, SFT and RL variants.
- The success of the model shows that small well -designed models can compete or exceed the performance of 32B models in reasoning tasks.
Discover the Paper And GitHub page. Also, don't forget to follow us Twitter And join our Telegram And Linkedin Group. Don't forget to join our 90K + ML Subdreddit.
Nikhil is an intern consultant at Marktechpost. It pursues a double degree integrated into materials at the Indian Kharagpur Institute of Technology. Nikhil is an IA / ML enthusiast who is still looking for applications in fields like biomaterials and biomedical sciences. With a strong experience in material science, he explores new progress and creates opportunities to contribute.
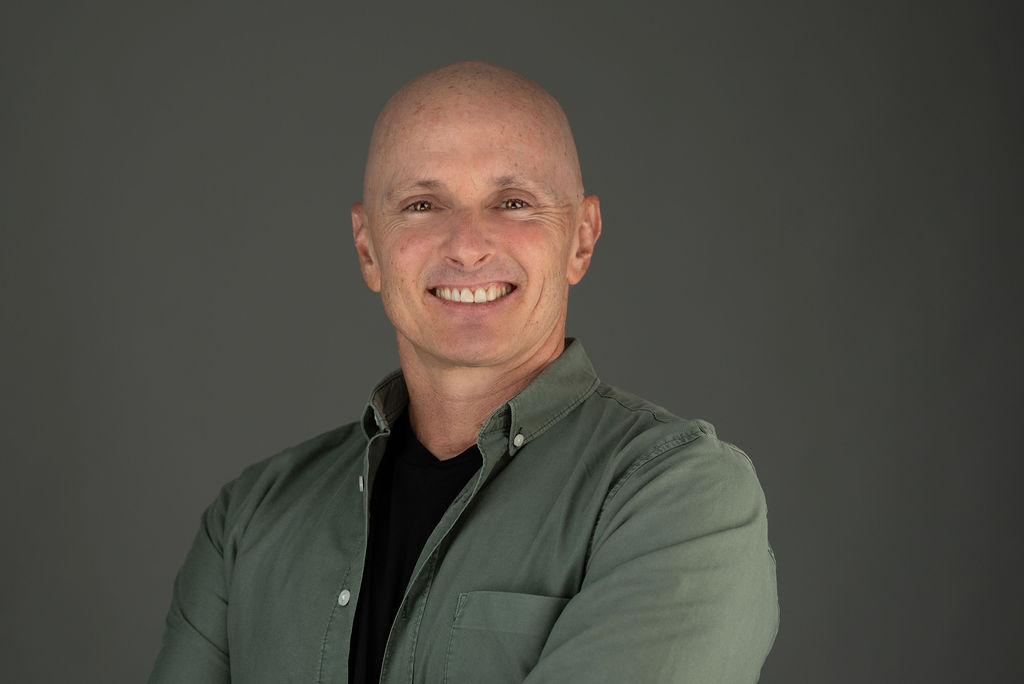