Each cell of your body contains the same genetic sequence, but each cell only expresses a subset of these genes. These models of gene expression specific to cells, which guarantee that a brain cell is different from a skin cell, are partly determined by the three -dimensional structure of the genetic material, which controls the accessibility of each gene.
MIT chemists have now found a new way of determining these 3D genome structures, using generative artificial intelligence. Their technique can predict thousands of structures in a few minutes, which makes it much faster than existing experimental methods to analyze structures.
Using this technique, researchers could more easily study how the 3D organization of the genome affects the models and the gene expression functions of individual cells.
“Our objective was to try to predict the structure of the three-dimensional genome of the underlying DNA sequence,” explains Bin Zhang, associate professor of chemistry and the main author of the study. “Now that we can do it, which puts this technique tied with advanced experimental techniques, it can really open many interesting opportunities.”
Students from MIT Greg Schuette and Zhuohan Lao are the main authors of the article, which appears today in Scientific advances.
From sequence to structure
Inside the cell nucleus, DNA and proteins form a complex called chromatin, which has several levels of organization, allowing cells to grasp 2 meters of DNA in a nucleus which is only one hundredth in a millimeter. Long strands of DNA wind around proteins called histones, giving birth to a structure a bit like pearls on a chain.
The chemical labels called epigenetic modifications can be attached to DNA at specific places, and these labels, which vary depending on the type of cell, affect the folding of chromatin and the accessibility of neighboring genes. These differences in the conformation of chromatin help to determine which genes are expressed in different types of cells, or at different times in a given cell.
Over the past 20 years, scientists have developed experimental techniques to determine the structures of chromatin. A widely used technique, known as Hi-C, works by connecting neighboring DNA strands in the nucleus of the cell. Researchers can then determine which segments are located to each other by shredding DNA in many tiny pieces and sequencing it.
This method can be used on large cell populations to calculate an average structure for a section of chromatin, or on unique cells to determine the structures in this specific cell. However, HI-C and similar techniques are with a high intensity of labor, and it can take about a week to generate data from a single cell.
To overcome these limits, Zhang and his students have developed a model that has taken advantage of the recent progress of the generative AI to create a rapid and precise means of predicting chromatin structures in unique cells. The AI ​​model they have designed can quickly analyze DNA sequences and predict the chromatin structures that these sequences could produce in a cell.
“Deep learning is really good in model recognition,” says Zhang. “This allows us to analyze very long DNA segments, thousands of base pairs, and to understand what important information coded in these DNA bases is.”
Chromogen, the model created by researchers, has two components. The first component, an in-depth learning model learned to “read” the genome, analyzes the information coded in the underlying DNA sequence and the accessibility data of the chromatin, the last of which is widely available and specific to the cell type.
The second component is a generative AI model which predicts physically precise chromatin conformations, having been formed on more than 11 million chromatin conformations. These data were generated from experiences using DIP-C (a variant of HI-C) on 16 cells from a line of human B lymphocytes.
When integrated, the first component informs the generative model of the way in which the cellular type environment influences the formation of different chromatin structures, and this scheme effectively captures sequence structure. For each sequence, researchers use their model to generate many possible structures. Indeed, DNA is a very disorderly molecule, so a single DNA sequence can give birth to many different possible conformations.
“A major complication factor in prediction of the genome structure is that there is not a single solution that we are targeting. There is a distribution of structures, whatever the part of the genome you look at. Predict this very complicated and high statistical distribution.
Rapid analysis
Once formed, the model can generate predictions on a much faster time scale than Hi-C or other experimental techniques.
“While you could spend six months to direct experiences to get a few dozen structures in a given type of cell, you can generate a thousand structures in a particular region with our model in 20 minutes on a single GPU,” explains Schuette.
After drawing their model, the researchers used it to generate structural predictions for more than 2,000 DNA sequences, then compared them to the structures determined experimentally for these sequences. They found that the structures generated by the model were identical or very similar to those observed in experimental data.
“We generally examine hundreds or thousands of conformations for each sequence, which gives you a reasonable representation of the diversity of structures that a particular region can have,” explains Zhang. “If you repeat your experience several times, in different cells, you will most likely end up with a very different conformation. This is what our model tries to predict.”
The researchers also found that the model could make precise data predictions from types of cells other than that on which it was formed. This suggests that the model could be useful for analyzing how chromatin structures differ between cell types and how these differences affect their function. The model could also be used to explore different chromatin states that can exist in a single cell, and how these changes affect the expression of genes.
“The chromogen provides a new framework for the discovery focused on the IA of the genome folding principles and demonstrates that the generating AI can fill the genomic and epigenomic characteristics with a 3D genome structure, pointing out future work on the study of the variation of the structure and the function of the genome through a wide fan of biological contexts,” explains Jian Computational biology at Carnegie Mellon University, which has not involved in research.
Another possible application would be to explore how mutations in a particular DNA sequence modify the conformation of chromatin, which could shed light on how such mutations can cause a disease.
“There are a lot of interesting questions that I think we can approach with this type of model,” says Zhang.
The researchers have created all their data and the model available to others who wish to use it.
Research was funded by the National Institutes of Health.
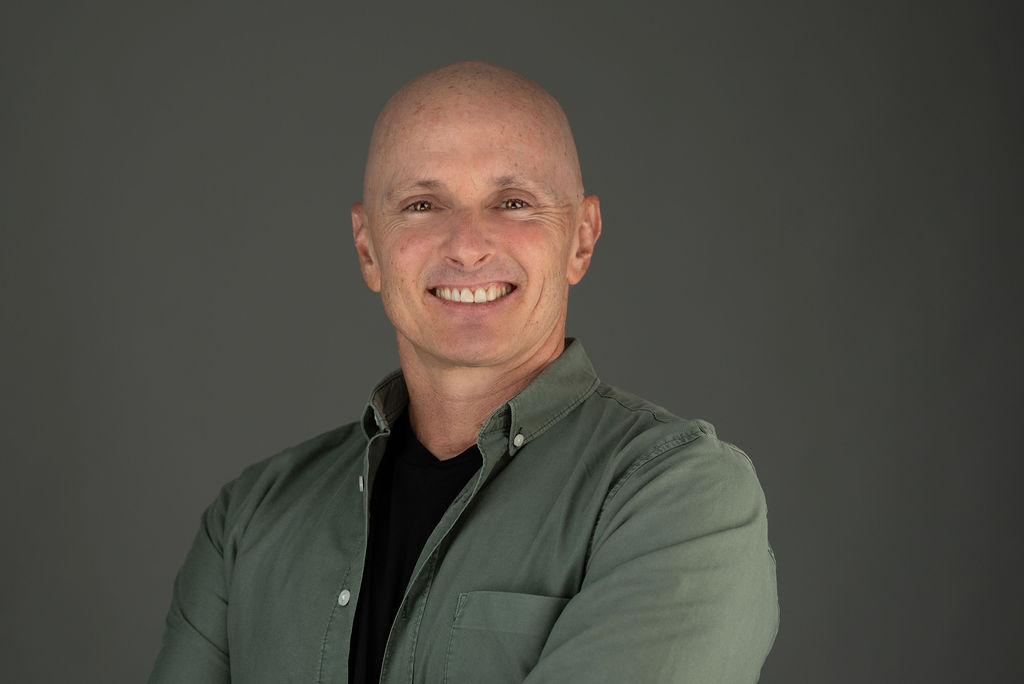