The clip frame has become fundamental in learning multimodal representation, in particular for tasks such as image text recovery. However, it faces several limitations: a strict cap at 77 on text entry, a double -encoder design which separates the processing of image and text, and a limited composition understanding which resembles models of bags. These problems hamper its effectiveness in the capture of nuanced semantics and sensitive to education. Although MLLM like Llava, Qwen2-VL and COGVLM provide significant progress in the reasoning in the language of vision, their objective of self-regressive prediction alongside Next restricts their ability to learn from generalized and transferable interests. This has aroused growing interest in the development of alternative methods that can combine the forces of contrasting learning and LLM -based reasoning.
Recent approaches aim to overcome these limits using new architectures and training strategies. For example, E5-V offers unimodal contrastive training to align intermodal characteristics, while VLM2 with the MMEB reference to convert advanced models into vision language into effective integration generators. Models such as LLM2W and NV-EMBED improve the learning of textual representation by modifying the attention mechanisms in the LLM only of the decoder. Despite these innovations, challenges such as the management of long sequences, allowing better intermodal fusion and effectively distinguishing hard negatives in contrasting learning remains. As multimodal applications develop, there is a pressing need for representation learning methods which are both evolving and capable of semantic fine -grain alignment.
Researchers from institutions such as the University of Sydney, Deepglint, Tongyi Lab in Alibaba and Imperial College London introduce Unime, a two -step frame designed to improve the learning of multimodal representation using MLLMS. The first step applies the distillation of textual discriminatory knowledge of a strong LLM teacher to improve linguistic encoder. The second step uses an adjustment of improved negative instructions, which involves filtering false negatives and sampling several difficult negatives for example to improve discriminating capacities and depending on model instructions. The evaluations on the MMEB reference and various recovery tasks show that Unime offers coherent and significant improvements both performance and understanding of composition.
The UNIME frame introduces a two -step method to learn multimodal universal incorporations using MLLMS. First, it uses a distillation of textual discriminatory knowledge, where a student MLLM is formed using text prompts only and supervised by a teacher model to improve the quality of integration. Then, a second step – adjustment of negative improved instructions – improves intermodal alignment and the performance of tasks by filtering false negatives and sampling hard negatives. This step also uses specific task prompts to improve instructions monitoring for various applications, such as the response to recovery and visual questions. Together, these steps considerably increase the performance of Unime on tasks at the same time and excluding distribution.
The study evaluated the Unime on Phi3.5-V and LLAVA-1.6 using Pytorch with a deep speed for effective training on 8 NVIDIA A100 GPU. The training consisted of two stages: a phase of distillation of textual knowledge using the NLI data set (273,000 pairs) and a phase of adjustment of the negative instruction lasts on 662,000 multimodal pairs. NV-EMBED V2 was the teacher model. Unime was evaluated on 36 MmeB reference data sets, making consistent improvements compared to basic lines such as E5-V and VLM2VEC. Hard negatives have considerably improved the model's ability to distinguish subtle differences, thus improving its performance, in particular in long -term recovery and composition tasks. Ablation studies have confirmed the effectiveness of training stages and adjustment parameters.
In conclusion, Unime is a two -step framework designed to improve the learning of multimodal representation using MLLMS. In the first step, Unih distills discriminating textual knowledge of a Great language model To strengthen the linguistic interests of the MLLM. In the second step, it improves learning by setting instructions with several hard negatives by lot, reducing false negative interference and encouraging the model to distinguish difficult examples. An in -depth evaluation on the MmeB and various recovery tasks show that Unime constantly stimulates performance, offering strong discriminating and composition capacities between tasks, thus exceeding the limits of previous models, such as Clip.
Discover the Paper And Code. Also, don't forget to follow us Twitter And join our Telegram And Linkedin Group. Don't forget to join our 90K + ML Subdreddit.
Sana Hassan, consulting trainee at Marktechpost and double -degree student at Iit Madras, is passionate about the application of technology and AI to meet the challenges of the real world. With a great interest in solving practical problems, it brings a new perspective to the intersection of AI and real life solutions.
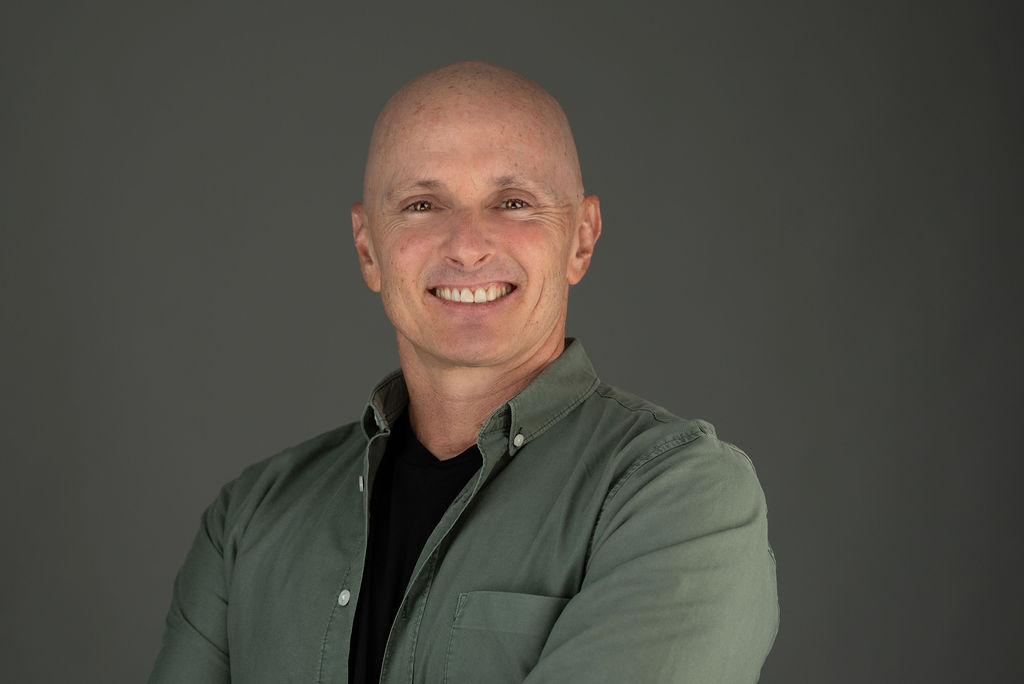