Reasoning language models, or RLMS, are increasingly used to simulate the resolution of step -by -step problems by generating long structured reasoning chains. These models decompose complex questions into simpler parts and create logical steps to reach the answers. This approach to the chain of thoughts (COT) has proven to be effective in improving the quality of production, especially in mathematical and logical tasks. Despite multilingual capacities in many major modern models, research and training have been largely focused on English, leaving a gap in understanding how these reasoning skills result in other languages.
A major challenge is that most RLMs are refined on English data, which limits their ability to reason effectively in other languages. This becomes particularly problematic for languages with low resources which have limited training examples. The models can by default models for reflection in English, producing lower quality outings when invited to another language. In addition, the differences in the structure of language can cause reasoning errors, in particular when a model formed in one language should deduce logic in another without adequate linguistic alignment.
Current techniques use strategies inviting zero-tirs or a few blows to manage these limits, often using English as a pivotal language. Certain efforts consist in presenting prompts in the same language as the request to preserve linguistic coherence. However, small models have minimum advantages due to limited capacity, and even large models have incoherent performance during reasoning in languages with low resources. Despite multilingual pre-training, the gap between training and the continuing reasoning language to hinder precise multilingual reasoning.
The Brown and Mbzuai University Research Team focused on the evaluation of how to increase the calculation of testing time, in particular through extended reasoning chains, can affect multilingual reasoning capacities centered on English. They studied the use of S1 models based on the instruct architecture of Qwen2.5 and refined 1,000 samples of Stem English. These models have been tested in various languages using benchmarks like MGSM and Global-MMLU to answer four basic questions: the effectiveness of time testing, linguistic mixing behaviors, performance under the fight against language and generalization of the cross domain.
In -depth experiences have shown that models with more parameters benefit significantly from the increase in testing tokens in testing time. The 14B S1 model, when it is set at 8,000 reflection tokens, has reached an average precision of 81% in non -English -speaking languages in MGSM. He outperformed models like Qwen2.5-14b-instruct by + 23.1% in French and + 41.6% in Swahili. Even if the model was only formed in English, its performances have exceeded that of larger models such as R1-Distill-Qwen-32B of Deepseek in several languages with high resources. The study also revealed that reasoning in high -resource languages like Chinese and English is more effective, requiring fewer tokens and offering better results than in low -resource languages such as Swahili or Telugou.
A key observation was the behavior of “quotation and thought”, where the model cited non -English -speaking sentences from invites and reasoned in English. This coherent scheme through languages like Japanese and Russian suggests that the model used its multilingual understanding to interpret non -English -speaking contributions without direct translation. Linguistic forcing experiences have also confirmed that forcing reasoning in high -resource languages has given better results, while strict reasoning in languages with low resources has led to significant precision cuts and calculation ineffections.
Despite strong results in STEM -related tasks, performance gains are not transferred to fields such as cultural common sense or human sciences. In benchmarks like Fork, the increase in reflection tokens has sometimes reduced performance, which indicates too much consumption. The study concludes that if the time scaling is improving multilingual reasoning in high -resource languages, it does not effectively generalize tasks outside the field or languages with low resources, indicating the need for additional research on balanced multilingual training and adaptation of the field.
Discover the Paper. All the merit of this research goes to researchers in this project. Also, don't hesitate to follow us Twitter And don't forget to join our 90K + ML Subdreddit.
Here is a brief overview of what we build on Marktechpost:
Nikhil is an intern consultant at Marktechpost. It pursues a double degree integrated into materials at the Indian Kharagpur Institute of Technology. Nikhil is an IA / ML enthusiast who is still looking for applications in fields like biomaterials and biomedical sciences. With a strong experience in material science, he explores new progress and creates opportunities to contribute.
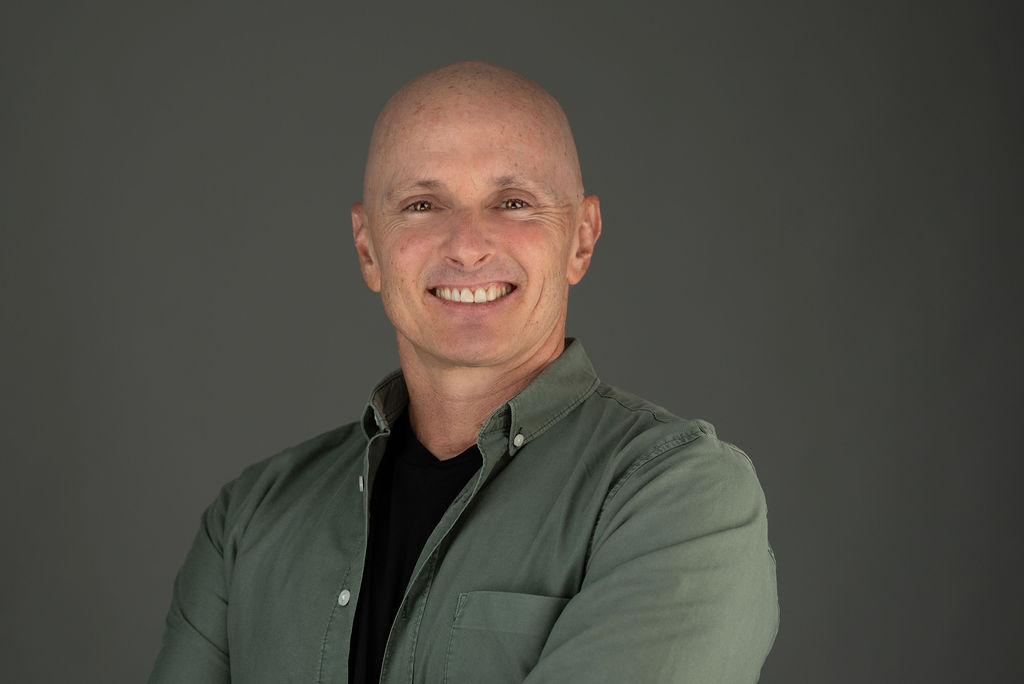