Reasoning with LLM can benefit from the use of more test calculations, which depends on high -quality process reward models (PRMS) to select promising paths for research or classification. The PRMS scores pairs of problematic solution to indicate whether the solution is correct and has been implemented as discriminating classifiers. However, these models require extensive resources, in particular human annotation, gold -step -by -step solutions or intensive deployments in calculation. LLM-AA-AA-JUDGE approaches offer advantages in terms of data efficiency and interpretation, but they work badly compared to specialized reward models for complex reasoning tasks, not recognizing incorrect reasoning. This creates a challenge to maintain the advantages of data efficiency and interpretability while achieving the higher performance of PRM discriminants.
Research approaches to resolve processes' verification challenges followed three main paths. Discriminating PRMs work as classifiers who predict digital accuracy scores for each reasoning stage, requiring in -depth level annotations. The generative verification of the PRMs of the PRMs as a task of language generation, producing accuracy decisions as chips in natural language accompanied by a chain of verification (COT). These models calculate the scores of accuracy thanks to parole probabilities like P (“correct”), which makes them intrinsically interpretable and evolving. Test scaling techniques such as selection of the best-de-n and research based on tree structures improve the performance of the reasoning using additional inference time. The effectiveness of these approaches strongly depends on the quality of the verifier for rating solutions.
Researchers from the University of Michigan, Mila, LG AI Research and the University of Illinois Urban-Champaign proposed ThinkPRM, a long cot verifier refined on process labels much less than those required by the discriminating prits. It uses the inherent reasoning capacities of long-cot models to surpass both LLM-Aa-Aa-Judge and Verifiant Discriminative while using only 1% of process labels in PRM800K through several difficult landmarks. As part of the equal token budgets, the verification of ThinkPRM scales calculates more effectively than LLM-as-after-judge, the outperforming it of 7.2% on a process bench subset, highlighting the value of long generative COT PRMs for checking the test test test with minimum supervision.
ThinkPRM is evaluated compared to DiscPRM, the same basic model focused on a binary cross entropy on the whole PRM800K data set containing 712K Process labels from 98K problem solution pairs. Additional comparisons include the voting of the unwindered majority and the weighted majority according to the auditor for the best experiences. The results are presented on three tasks of mathematical reasoning: 100 math-500 problems covering all the difficulty levels, 2025 American Invitational Mathematics Examination (LOVE) Problems and tasks out of the field, including GPQA-Diamond physics and a subset of 200-Problem Problems from LiveCodebench V5. For Math-500, the researchers used ThinkPRM-1.5B and ThinkPRM-14B with two different generators.
On the selection of the best of N with Math500, ThinkPRM reaches a higher reasoning precision or comparable to discprm in all sampling budgets. Under research guided by the auditor on Math-500, ThinkPRM-1.5B surpasses the discprm of approximately 5 percentage points and exceeds LLM-A-A-Judge using the same basic model (R1-QWEN-1.5B). The ThinkPRM-1.5B scaling curve exceeds all the basic lines compared to strict strong PRMs like RLHFFLOW-DEEPSEEK-PRM and MATH-SHEPHERD-PRM, surpassing RLHFFLOW-DEEPSEEK-PRM more than 7% at 16 bundles. For the evaluation outside the field, ThinkPRM shows a better scaling than the DiscPRM on GPQA-Physics, outperforming it by 8%, while on Livecodebench, ThinkPRM exceeds the discprm of 4.5%.
In conclusion, the researchers introduced ThinkPRM, a reward model for generative process formed with minimum supervision on synthetic data, allowing an effective and evolving verification of the reasoning step by step. Researchers show that generative LIM light adjustment on as little as 8K process labels can improve the LLM-AAAA-Judge reference bases. ThinkPRM also exceeds PRM discriminants formed with the orders of magnitude of process labels, highlighting the advantages of the use of the generative objectives of language modeling for interpretability, scalability and efficiency of data. The results underline the generative PRM potential for verification at the calculation scale at the time of the test effectively, benefiting to difficult areas such as mathematical and scientific reasoning.
Discover the Paper. Also, don't forget to follow us Twitter And join our Telegram And Linkedin Group. Don't forget to join our 90K + ML Subdreddit.
Sajjad Ansari is a last year's first year of the Kharagpur Iit. As a technology enthusiast, he plunges into AI's practical applications by emphasizing the understanding of the impact of AI technologies and their real implications. It aims to articulate complex AI concepts in a clear and accessible way.
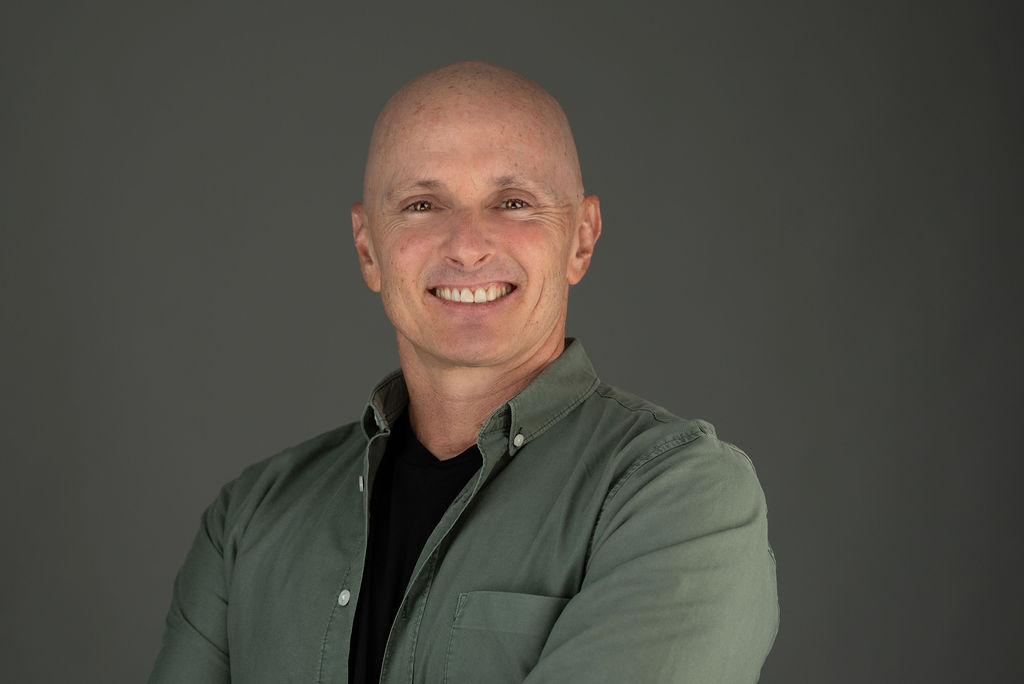