Proteins are the work horses that keep our cells on the move, and there are several thousand types of protein in our cells, each fulfilled a specialized function. Researchers have long since known that the structure of a protein determines what it can do. More recently, researchers appreciate that the location of a protein is also essential for its function. The cells are full of compartments that help organize their many residents. In addition to well -known organelles that adorn the pages of biology manuals, these spaces also include a variety of dynamic compartments without membrane which concentrate certain molecules together to perform shared functions. Knowing where a given protein is located and with whom it is colocalizing can therefore be useful for better understanding than proteins and its role in the healthy or sick cell, but researchers lacked systematic means of predicting this information.
Meanwhile, the protein structure has been studied for more than half of a century, leading to the artificial intelligence tool Alphafold, which can predict the structure of proteins from the amino acid code of a protein, the linear chain of construction blocks in it which folds up to create its structure. Alphafold and models as they have become widely used tools in research.
Proteins also contain regions of amino acids that do not fall back into a fixed structure, but are rather important to help proteins join dynamic compartments in the cell. Professor of MIT Richard Young and his colleagues wondered if the code in these regions could be used to predict the location of proteins in the same way as other regions are used to predict the structure. Other researchers have discovered certain protein sequences that code for the location of proteins, and some have started to develop predictive models for the location of proteins. However, researchers did not know if the location of a protein in a dynamic compartment could be predicted according to his sequence, and they did not have a tool comparable to Alphafold either to predict location.
Now Young, also a member of the Whitehead Institute for Biological Research; Young Lab Postdoc Henry Kilgore; Regina Barzilay, the professor of the School of Engineering for AI and Health in the Department of Electric Engineering and Computer Science and Principal Investigator of the IT intelligence and artificial intelligence laboratory (CSAIL); And colleagues have built such a model, which they call protgps. In an article published on February 6 in the newspaper ScienceWith the first authors Kilgore and the students graduated from Barzilay Lab Itamar Chinn, Peter Mikhael and Ilan Mitnikov, the interdisciplinary team launches their model. Researchers show that Protgps can predict which 12 types of known compartments, a protein will be located, as well as if a mutation associated with the disease will change this location. In addition, the research team has developed a generative algorithm which can design new proteins to locate themselves in specific compartments.
“I hope it is a first step towards a powerful platform that allows people who study proteins to do their research,” says Young, “and that she helps us to understand how humans develop in complex organisms that they are, how mutations disturb these natural processes and how to generate therapeutic hypotheses and drugs to treat dysfunction in a cell.”
Researchers have also validated many model forecasts with experimental tests in cells.
“It really excited me to be able to go from computer design to the point of trying these things in the laboratory,” explains Barzilay. “There are many exciting articles in this area of AI, but 99.9% of these are never tested in real systems. Thanks to our collaboration with Young Lab, we were able to test and really learn how well our algorithm is. ”
Develop the model
The researchers have formed and tested protgps on two lots of protein with known locations. They found that this could predict properly where the proteins are found with great precision. Researchers also tested the way PRTGPS could predict changes in the location of proteins according to mutations associated with disease within a protein. Many mutations – modifications to the sequence of a gene and its corresponding protein – have proven to be contributing or causing a disease based on association studies, but the ways whose mutations cause symptoms of the disease remain unknown.
It is important to determine the mechanism of how a mutation contributes to the disease is important because then researchers can develop therapies to repair this mechanism, prevent or treat the disease. Young people and colleagues suspected that many changes associated with the disease could contribute to the disease by modifying the location of proteins. For example, a mutation could make a protein incapable of joining a compartment containing essential partners.
They tested this hypothesis by nourishing protgos of more than 200,000 proteins with mutations associated with the disease, then asking it to predict that these mutated proteins are localized and measure how its prediction has changed for a given protein from the normal version to the mutated version. A great change in prediction indicates a likely change in location.
The researchers found many cases in which a mutation associated with the disease seemed to change the location of a protein. They tested 20 examples in cells, using fluorescence to compare where in the cell a normal protein and the mutated version of it found themselves. Experiences have confirmed Protgps predictions. Overall, the results support the suspicion of researchers that poor location can be an underestimated disease mechanism and demonstrate the value of protgps as a tool to understand the disease and identify new therapeutic avenues.
“The cell is such a complicated system, with so many components and networks of complex interactions,” explains Mitnikov. “It is super interesting to think that with this approach, we can disturb the system, see the result of this, and thus discovery of mechanisms in the cell, or even develop therapies on the basis of this.”
Researchers hope that others are starting to use protgps in the same way that they use predictive structural models like AlphaFold, making various projects progress on the function of proteins, dysfunction and disease.
Go beyond prediction to a new generation
The researchers were enthusiastic about the possible uses of their prediction model, but they also wanted their model to go beyond the forecast of the locations of existing proteins and allow them to design completely new proteins. The objective was that the model compensates for sequences of entirely new amino acids which, when formed in a cell, would locate itself in a desired location. The generation of a new protein which can really perform a function – in this case, the function of locating in a specific cellular compartment – is incredibly difficult. In order to improve the chances of success of their model, researchers have forced their algorithm to design only proteins like those found in nature. This is an approach commonly used in the design of drugs, for logical reasons; Nature has had billions of years to determine which protein sequences work well and which do not.
Due to collaboration with Young Lab, the automatic learning team was able to test if their protein generator worked. The model had good results. In one turn, it generated 10 proteins intended to locate itself in the nucleolus. When the researchers tested these proteins in the cell, they found that four of them were strongly located in the nucleolus, and others may also have had slight biases towards this place.
“The collaboration between our laboratories has been so generative for all of us,” explains Mikhael. “We have learned to talk about the languages of each other, in our case, we have learned a lot about the functioning of cells, and having the chance to test our model experimentally, we were able to understand what we have to do so that the model really works, then make it better.”
Being able to generate functional proteins in this way could improve researchers' ability to develop therapies. For example, if a drug must interact with a target that is located in a certain compartment, researchers could use this model to design a medication to locate there too. This should make the drug more effective and decrease side effects, as the drug will spend more time engaging with its target and less time interacting with other molecules, causing off -target effects.
The members of the automatic learning team are enthusiastic about the prospect of using what they have learned from this collaboration to design new proteins with other functions beyond location, which would extend the possibilities of therapeutic design and other applications.
“Many articles show that they can conceive of a protein that can be expressed in a cell, but not that the protein has a particular function,” explains Chinn. “We actually had a functional protein design and a relatively enormous success rate compared to other generative models. It is really exciting for us, and something on which we would like to build.”
All the researchers involved consider Protgps as an exciting beginning. They provide that their tool will be used to find out more about the roles of location in the function of proteins and poor location of the disease. In addition, they wish to extend the predictions of location of the model to include more types of compartments, test more therapeutic hypotheses and design increasingly functional proteins for therapies or other applications.
“Now that we know that this protein code for location exists, and that automatic learning models can give meaning to this code and even create functional proteins using its logic, which opens the door to so many potential studies and applications,” explains Kilgore.
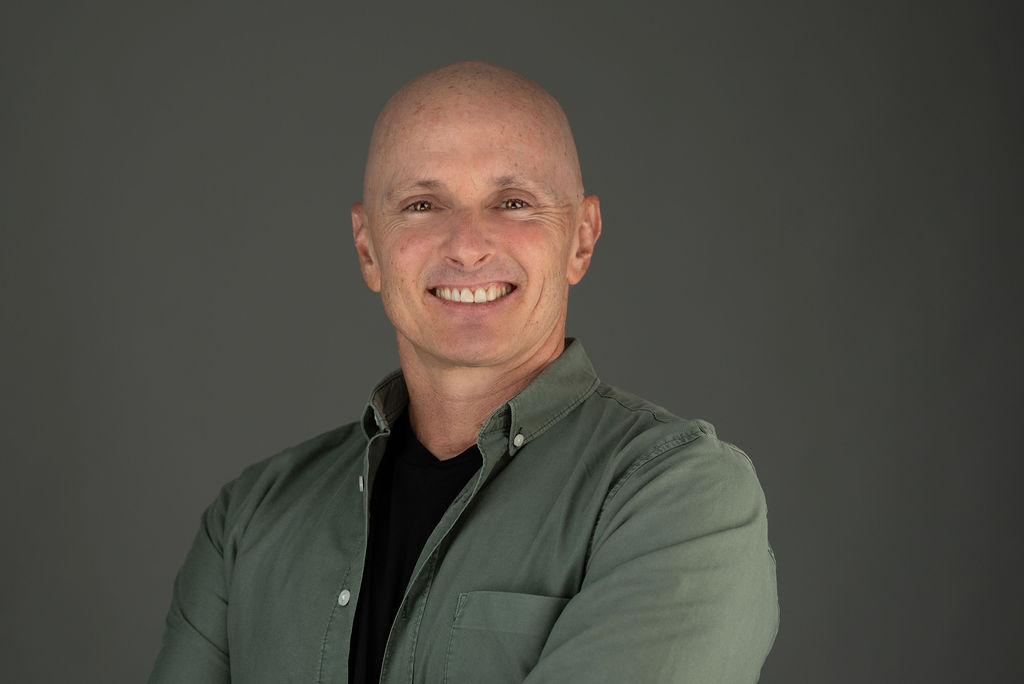