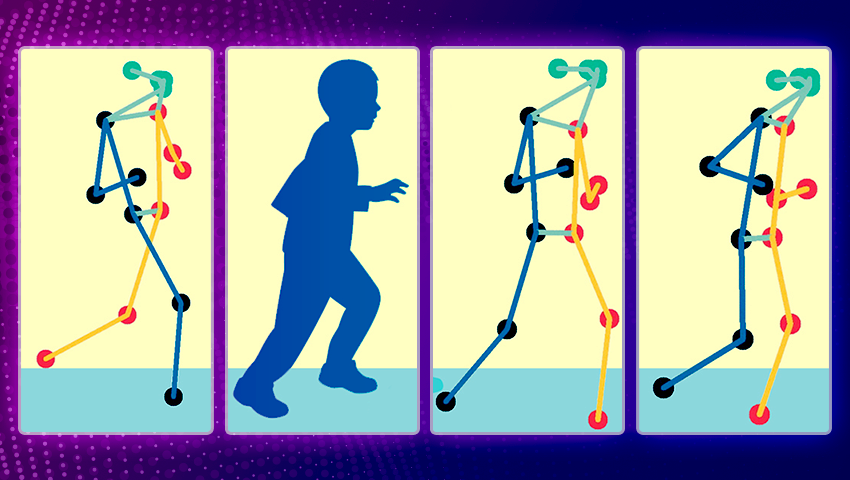
In the field of medical diagnostics, innovation does not know any limits. Recently, an exciting breakthrough has emerged at the intersection of computer vision and automatic learning, promising to revolutionize the way we assess and assess patients, especially those who suffer from motor disorders such as cerebral paralysis. This revolutionary development, known as Pose mapping techniqueshould reshape the landscape of medical diagnosis and patient care.
Traditionally, the evaluation of the motor function of patients, in particular for conditions such as cerebral paralysis, requires frequent visits in person in the doctor's office. This process can not only be bulky but also financially heavy and emotionally trying, especially for children and their parents. However, thanks to this cutting -edge technique developed by MIT engineers, we are now at the dawn of a transformer leap in front in the medical assessment.
At the heart of this innovation is the transparent integration of computer vision and automatic learning. By exploiting these advanced technologies, the installation mapping technique has released the potential to remotely assess the motor function of patients. The method analyzes the real -time videos of patients, deciphering specific poses models in these videos. This analysis, driven by automatic learning algorithms, calculates a clinical motor function score.
To develop a technique of analyzing skeletal installation data in patients with brain paralysis, a condition generally evaluated using the classification system for the gross motor function (GMFC), scientists use a five -level scale representing the global motor function of a child (lower numbers indicate higher mobility).
The team used a public set of skeletal installation data provided by the neuromuscular biomechanics laboratory of the University of Stanford. This set of data contained videos featuring more than 1,000 children with brain paralysis, each demonstrating various exercises in a clinical environment. In addition, each video was marked with a GMFCS score assigned by a clinician following an evaluation in person. The Stanford group has processed these videos through an installation estimate algorithm to generate skeleton installation data, serving as a basis for the subsequent MIT study.
Remarkably, the installation mapping technique had a precision rate greater than 70% which corresponds to the assessments of clinicians during evaluations in person. This level of precision is a huge promise to rationalize patient assessments and reduce the need for frequent and arduous travel in medical facilities.
The potential applications of the installation mapping technique extend far beyond cerebral paralysis. The research team currently adapts the approach to assess children with metachromatic leukodystrophy, a rare genetic disorder affecting the nervous system. In addition, they actively work on the adaptation of the method to assess patients who have experienced a stroke.
Hermano Krebs, principal researcher of the MIT mechanical engineering department, is considering a future where patients can reduce their dependence on hospital visits for assessments. “We believe that this technology could potentially be used to remotely assess any condition that affects engine behavior,” he said. This implies a seismic change in the way we approach medical assessments and patient care.
The road to this remarkable achievement began with computer vision and algorithms designed to estimate human movements. The installation estimation algorithms paved the way for the translation of video sequences in skeleton poses. These poses, represented in the form of lines and points, were then mapped in coordinates for a more in -depth analysis.
The research team has operated a network of convolutionary neurons of spatial-temporal graph to decipher the models in cerebral paralysis data, classifying the levels of mobility of patients. Surprisingly, network training on a wider set of data, which included healthy adult videos performing daily activities, has considerably improved its precision in the classification of patients with cerebral paralysis.
The real wonder of this innovation is its accessibility. The method can be carried out transparently on a multitude of mobile devices, guaranteeing generalized availability and real -time processing of videos. The MIT team actively develops an application that could allow patients to take control of their self-assessments. This application would allow parents and patients to record videos in the comfort of their homes. The results could then be shared effortlessly with health professionals, paving the way for more informed and timely interventions. In addition, the adaptability of the method extends to the evaluation of other neurological disorders, promising to reduce health care costs and improve patient care.
As mentioned, the integration of computer vision and automatic learning quickly transforms the landscape of medical diagnostics. At Qudata, we share this enthusiasm for innovative solutions in medical imaging, contributing to a better future for health care. Our case studies more closely provide our ML research and solutions. Learn more about our latest project Detection assisted by breast cancer – AI -based solution to improve the accuracy and efficiency of diagnostic for breast cancer.
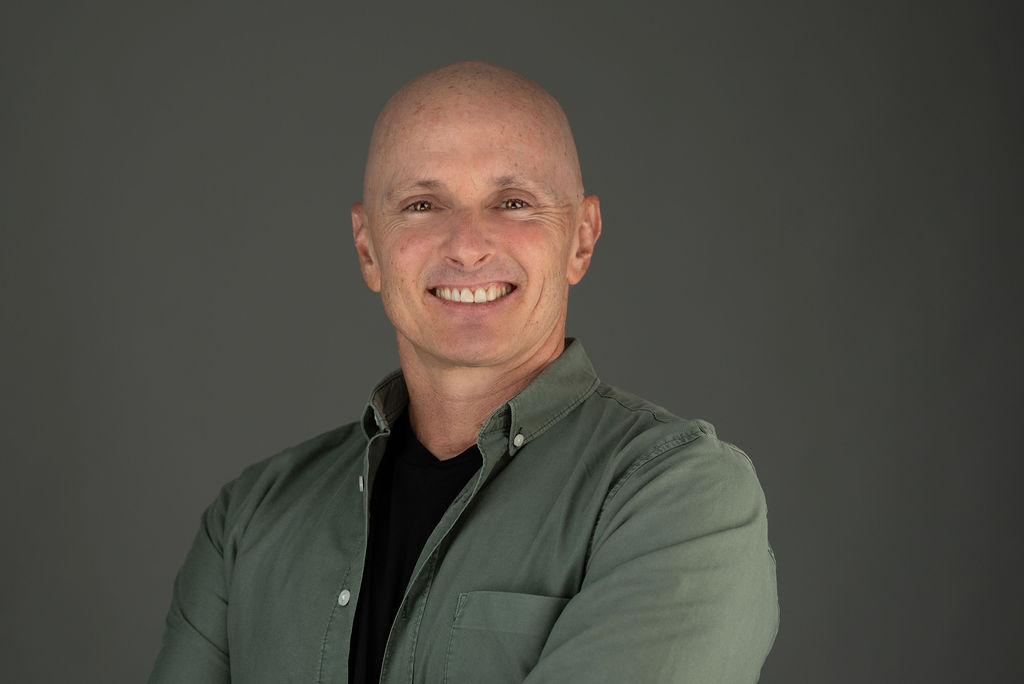