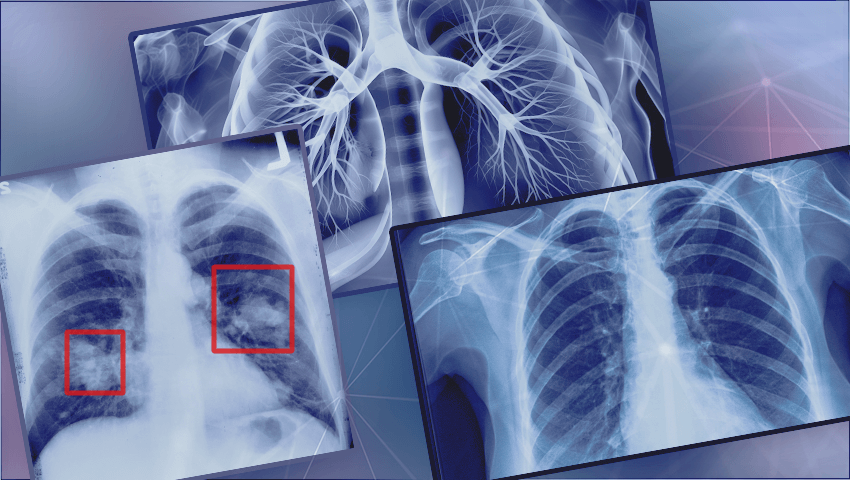
A new artificial intelligence system ITPCTRL-AI promises to considerably improve X-ray diagnostics By offering both interpretability and controllability – take up the long -standing challenge for AI transparency in medical imaging. Developed by researchers from the University of Arkansas in collaboration with MD Anderson Cancer Center, ITPCTRL-AI models the models of radiologists To ensure that its decision -making process aligns with human expertise.
AI diagnostic tools have demonstrated remarkable precision in the detection of medical anomalies, such as the accumulation of liquid in the lungs, extended hearts and the first signs of cancer. However, many of these AI models work as “black boxes”, which makes health professionals difficult to understand how the conclusions have reached.
According to NGAN LE, assistant teacher in computer science and computer engineering at the University of Arkansas, transparency is essential to the adoption of AI in medicine. “When people understand the reasoning process and the limits behind AI decisions, they are more likely to trust and adopt technology,” said the.
ITPCTRL-AI, short for interpretable and controllable artificial intelligence, was designed to fill this gap by reproducing how radiologists analyze the thoracic X-rays. Unlike conventional AI systems which simply predict diagnoses, ITPCTRL -AI generates thermal cards of the gaze – visual representations of the zones on radiologists on their examination. These thermal cards provide a transparent vision of the AI decision -making process, improving both confidence and interpretability.
To develop this AI model, researchers followed the eye movements of radiologists when they examined X -ray images. They registered not only when the experts seemed, but also how long they focused on specific areas before reaching a diagnosis. The data collected were then used to train ITPCTRL-AI, which allows it to generate attention heat cards that highlight key diagnostic regions in an image.
By taking advantage of these eyes -based ideas, the system of AI filters the unrelevant areas before making a diagnostic prediction, ensuring that it considers only significant information – just like a human radiologist. This attention-based decision-making approach makes ITPCTRL-Ai much more interpretable than traditional AI models.
To support the development of ITPCTRL-AI, the researchers created diagnosegaze ++, a first data set of its kind that aligns medical results with radiologists' view data. Unlike existing data sets, diagnosed ++ provides detailed anatomical attention cards, establishing a new standard for diagnostic transparency led by AI.
By using a semi-automated approach, the research team filtered and structured radiologists tracking data, ensuring that each thermal card corresponded precisely to medical anomalies. This data set improves not only the interpretability of the AI, but also opens the way to future progress in medical imaging AI.
ITPCTRL-AI is not the only system focused on AI which advances the transparency of medical imaging. At Qudata, we also use GRAD-CAM (activation mapping mapping cartography in gradient) to generate thermal cards for Mammography analysis.
At the base, Grad-Cam highlights the most influential regions of an image This contributes to the decision of the AI model, allowing radiologists to locate areas of interest with greater precision. This technique guarantees that the detection of breast cancer assisted by AI remains explained and aligned with medical expertise. By integrating visual explanations based on the thermal card, the solutions fueled by ITPCTRL-AI and QUDATA improve confidence and conviviality in clinical environments.
Transparency in AI diagnosis is not only technical progress – it is an ethical necessity. The ability to explain AI decisions is crucial to guarantee equity, attenuation of the bias and the maintenance of responsibility in health care. With legal and ethical concerns concerning medical AI, ITPCTRL-AI offers a model that allows doctors to take responsibility for the AI diagnosis.
The research team now strives to improve ITPCTRL-AI to analyze the three-dimensional CT scanners, which require even more complex decision-making processes. By incorporating depth information and wider anatomical structures, the AI system could further improve diagnostic accuracy in critical medical applications.
To encourage additional research and adoption, the source code, the models and all annotated data of the project will be implemented publicly. This initiative aims to define a new reference for transparency and responsibility focused on AI in medical imaging.