Border AI companies show progress towards general artificial intelligence (AG), creating a need for techniques to ensure that these powerful systems remain controllable and beneficial. A major approach to this challenge implies methods such as recursive reward modeling, iteral amplification and evolutionary surveillance. They aim to allow weaker systems to effectively supervise stronger systems. A key idea is that evolutionary surveillance can be recursive, which is called nested evolutionary monitoring (NSO). However, although discussions on the ONS focus on qualitative guarantees and conceptual executives, other high -risk technologies are held according to quantitative safety standards, for example, civil aircraft must maintain death rates below 10 –5 per flight hour and nuclear reactors must maintain the frequency of damage to the heart within 10-4 per year.
Evolutionary surveillance processes where AI systems monitor the strongest include iteral amplification, recursive reward modeling, IA security via debate, market manufacturing, advice, self -criticism and double effective debate. Research on forecasting and scaling laws focused on how model performance improves with size, data and calculation resources. However, these approaches can also apply to behavior such as surveillance and deception. In addition, long -term surveillance roadmaps include the OpenAi supper plan for “Automated human level researchers” and a complete Safety Cadre. In addition, previous studies have examined the performance of the linguistic model in scenarios related to surveillance such as social deduction games, AI debate, stolen door code detection and wargame simulations.
MIT researchers have proposed a framework to quantify the probability of successful monitoring depending on the capacities of the monitoring and supervision system. This model surveillance frame as a game between capacity players, where everyone has specific Elo scores and specific to the deception which follows a linear function in pieces of their general intelligence. A theoretical study of nested evolutionary surveillance (NSO) is conducted, where trust models supervise more reliable models. This identifies the conditions of success NSO and derives the optimal number of surveillance levels to maximize the probability of success. Examples show NSO success rates below 52% when supervising 400 Elo systems stronger than the basic supervisor.
The search examines four surveillance games:
- In the Mafia game, five villagers (guards) try to identify a mafia player (Houdini), creating a natural environment to test the skills of deception and collaboration.
- In the debate, two contradictory agents support opposite views while a judge (guard) determines the good side. Test cases are derived from truthful, Boolq, quality and pronttoqa data sets.
- The stolen door code tests the capacities of language models to insert and detect hidden behaviors in the code. A pipeline is designed to generate drifts using 225 python samples from the apps data set at the level of competition difficulty.
- Wargames simulate a fictitious company called Openmind approaching AG development. These are three players: the Openmind AI (Houdini), the Openmind monitor (Guard) and a narrator.
The performance of the mafia evolves approximately linearly with general information for the roles of the mafia (Houdini) and the villagers (guard), where the guards have stronger improvement slopes. In debate evaluations using TrueQA, guard's performance scales with general information while Houdini's performance remains relatively constant. In the stolen door code tests, Guard and Houdini Elos are on a scale with general intelligence but asymmetrically. The guards maintain higher interceptions, indicating that the task is intrinsically more difficult for the Houdinis, even with the strongest misleading models not exceeding the success rates of 50% against the lower guards. In Wargames, the systematic analysis of 100 summaries of random narrator by Gemini 2.5 has identified eight strategy categories, most models showing similar strategy profiles regardless of the level of capacity.
In conclusion, the researchers introduced a framework to quantify the probability of successful monitoring according to the capacities of the monitoring and supervision system. Although the surveillance parameters aim for realism, they remain simplified approximations. Each game has a fundamental constraint to simulate only surveillance in a single step without immediate generalization at several stages. Future research could explore surveillance channels in several steps, similar to solid models which are successively refined on the behavior of lower models. Another significant limitation implies the modeling of deceptive behavior. The current approach invites models to act in a deceptive way rather than using fine settings or reinforcements to develop truly deceptive models.
Discover the Paper. Also, don't forget to follow us Twitter And join our Telegram And Linkedin Group. Don't forget to join our 90K + ML Subdreddit. For promotion and partnerships, Please talk to us.
Sajjad Ansari is a last year's first year of the Kharagpur Iit. As a technology enthusiast, he plunges into AI's practical applications by emphasizing the understanding of the impact of AI technologies and their real implications. It aims to articulate complex AI concepts in a clear and accessible way.
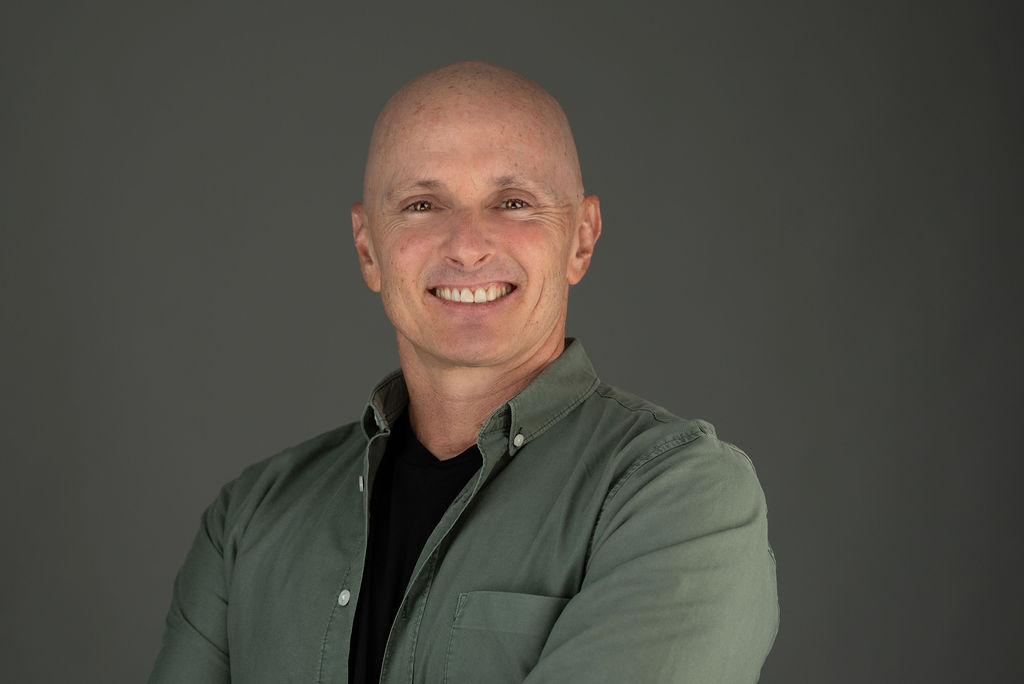