Agent Development Kit (ADK) is an open source python framework that helps developers create, manage and deploy multi-agent systems. It is designed to be modular and flexible, which makes it easy to use for applications based on simple and complex agents.
In this tutorial, we will create a simple AI agent using ADK. The agent will have access to two tools:
- Get_company_overView
- Get_earnings
Google API key
To use Google AI services, you will need an API key:
Alphavant Api key
To access financial data, we will use the Alpha Vantage API:
- Go to https://www.alpha further.co/
- Click “Get your free API key” or visit This direct link
- Enter your email and follow the instructions
- Once you have received your API key, copy it and save it safely. We will use it to authenticate the requests for financial terminals.
Python libraries
We only need one package:
Configure your project file with the following structure:
parent_folder/
│
└───multi_agent/
├── __init__.py
├── agent.py
└── .env
__init__.py
Paste the following code in Multi_agent / __ Init__.Py:
.G
Create a .VED file in the multi_agent folder and glue the following:
GOOGLE_GENAI_USE_VERTEXAI=FALSE
GOOGLE_API_KEY=""
ALPHA_VANTAGE_API_KEY="
Replace Spaces reserved with your real API keys
agent.py
Paste the following code in the Agent.Py file:
from google.adk.agents import Agent
import requests
import os
from typing import Optional
ALPHA_VANTAGE_API_KEY = os.getenv("ALPHA_VANTAGE_API_KEY")
def get_company_overview(symbol: str) -> dict:
"""
Get comprehensive company information and financial metrics
Args:
symbol: Stock ticker symbol (e.g., IBM)
Returns:
dict: Company overview data or error
"""
if not ALPHA_VANTAGE_API_KEY:
return {"status": "error", "error": "Missing API key"}
base_url = "https://www.alphavantage.co/query"
params = {
"function": "OVERVIEW",
"symbol": symbol,
"apikey": ALPHA_VANTAGE_API_KEY
}
try:
response = requests.get(base_url, params=params)
response.raise_for_status()
data = response.json()
if "Error Message" in data:
return {"status": "error", "error": data("Error Message")}
# Filter key metrics
key_metrics = {
"Description": data.get("Description"),
"Sector": data.get("Sector"),
"MarketCap": data.get("MarketCapitalization"),
"PERatio": data.get("PERatio"),
"ProfitMargin": data.get("ProfitMargin"),
"52WeekHigh": data.get("52WeekHigh"),
"52WeekLow": data.get("52WeekLow")
}
return {
"status": "success",
"symbol": symbol,
"overview": key_metrics
}
except Exception as e:
return {"status": "error", "error": str(e)}
def get_earnings(symbol: str) -> dict:
"""
Get annual and quarterly earnings (EPS) data with analyst estimates and surprises
Args:
symbol: Stock ticker symbol (e.g., IBM)
Returns:
dict: Earnings data with estimates or error message
"""
if not ALPHA_VANTAGE_API_KEY:
return {"status": "error", "error": "Missing API key"}
base_url = "https://www.alphavantage.co/query"
params = {
"function": "EARNINGS",
"symbol": symbol,
"apikey": ALPHA_VANTAGE_API_KEY
}
try:
response = requests.get(base_url, params=params)
response.raise_for_status()
data = response.json()
if "Error Message" in data:
return {"status": "error", "error": data("Error Message")}
# Process annual and quarterly earnings
annual_earnings = data.get("annualEarnings", ())(:5) # Last 5 years
quarterly_earnings = data.get("quarterlyEarnings", ())(:4) # Last 4 quarters
# Format surprise percentages
for q in quarterly_earnings:
if "surprisePercentage" in q:
q("surprise") = f"{q('surprisePercentage')}%"
return {
"status": "success",
"symbol": symbol,
"annual_earnings": annual_earnings,
"quarterly_earnings": quarterly_earnings,
"metrics": {
"latest_eps": quarterly_earnings(0)("reportedEPS") if quarterly_earnings else None
}
}
except Exception as e:
return {"status": "error", "error": str(e)}
root_agent = Agent(
name="Financial_analyst_agent",
model="gemini-2.0-flash",
description=(
"Agent to give company overviews with key financial metrics."
),
instruction=(
"You are a helpful AI agent that provides company overviews and earnings information"
),
tools=(get_company_overview, get_earnings),
)
In this script, we define a financial analysis agent using the Google Agent Development Kit (ADK). The agent is designed to respond to user requests by accessing real -time financial data via the Alpha Vantage API. More specifically, he exhibits two tools: Get_Ccompany_overView and Get_earnings. The Get_Ccompany_overView function recovers the details of the key company such as the sector, market capitalization, the P / E ratio and the high / low values of 52 weeks. The Get_earnings function provides annual and quarterly profit data, including reported BPA and surprise percentages. To create the agent, we use the agent class from the Google.Adk.agents module, giving it a name, a model (for example, Gemini 2.0 Flash), a description and an instructions. The agent is then equipped with the two tools mentioned above, which allows him to answer questions related to the finance of the company.
To execute the agent, go to the parent directory of your agent project (for example using CD ..)
parent_folder/ ← Navigate to this directory in your terminal
│
└───multi_agent/
├── __init__.py # Initializes the module
├── agent.py # Contains the agent logic and tools
└── .env # Stores your API keys securely
After navigation, run the following code:
Open the supplied URL (usually http: // Localhost: 8000 or http://127.0.0.1:8000) directly in your browser. You will see a simple cat interface where you can interact with your agent using the input text box.
In addition, you can inspect each stage of the agent's reasoning by clicking on Acts. This allows you to see:
- The tools called
- Entries and exits of each function
- The answers generated by the language model
You can find the entire code with the structure of the folder on this link: https://github.com/mohd-arham-islam/adk-demo
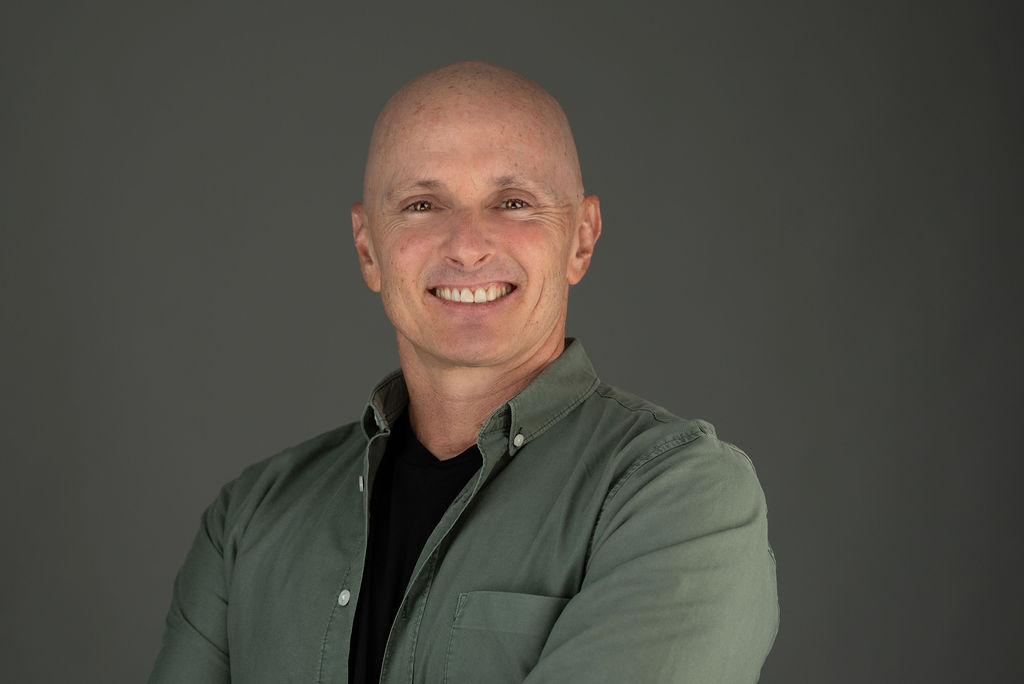