Imagine a coffee business trying to optimize its supply chain. The company supplies on three suppliers, the roasts in two dark or light coffee installations, then ships roasted coffee with three retail seats. Suppliers have different fixed capacities, roasting costs and shipping costs vary from location to the next.
The company seeks to minimize costs while fulfilling a 23% increase in demand.
Wouldn't it be easier for the company to ask Chatgpt to offer an optimal plan? In fact, despite all their incredible capacities, large-language models (LLM) often work badly when they are responsible for solving such planning problems complicated by themselves.
Rather than trying to change the model to make an LLM a better planner, MIT researchers adopted a different approach. They introduced a frame that guides an LLM to break the problem like a human, then solve it automatically using a powerful software tool.
A user only needs to describe the problem in natural language – no specific example for the task is necessary to train or invite the LLM. The code model for the text prompt of a user in a format which can be untangled by an optimization solver designed to effectively remove extremely difficult planning challenges.
During the formulation process, the LLM checks its work at several intermediate steps to ensure that the plan is described correctly to the solver. If he spots an error, rather than abandoning, the LLM tries to repair the broken part of the formulation.
When the researchers tested their framework on nine complex challenges, such as the minimization of distance warehouse robots must move to perform tasks, it has reached a success rate of 85%, while the best reference basis has only reached a success rate of 39%.
The versatile frame could be applied to a range of planning tasks in several stages, such as air team planning or machine time management in a factory.
“Our research presents a framework that acts essentially as an intelligent assistant for problems planning. He can understand the best plan that meets all the needs you have, even if the rules are complicated or unusual, “explains Yilun Hao, a student graduated from the MIT Laborator for Information and Decision Systems (LIDS) and principal author of a Document on this research.
She is joined on the article by Yang Zhang, a scientific researcher at the Mit-ibm Watson Ai Lab; And the main author Chuchu Fan, associate professor of aeronautics and astronautics and principal investigator of Light. Research will be presented at the international conference on representations of learning.
Optimization 101
The group of fans develops algorithms that automatically solve what are called combinatorial optimization problems. These vast problems have many interdependent decision variables, each with several options which are quickly added to billions of potential choices.
Humans solve such problems by narrowing them in a few options, then determining which leads to the best global plan. The algorithmic solvers of researchers apply the same principles to optimization problems which are far too complex for a human.
But the resolvers they develop tend to have high learning curves and are generally only used by experts.
“We thought LLM could allow non-experts to use these resolution algorithms. In our laboratory, we take an expert problem in the field and formalize it in a problem that our solver can solve. Could we teach an LLM to do the same? ” The fan says.
Using the framework that researchers have developed, called formalized programming based on LLM (LLMFP), a person provides a description of the natural language of the problem, basic information on the task and a question that describes their objective.
Then, LLMFP invites an LLM to reason on the problem and determine the decision variables and the keys constraints that will shape the optimal solution.
LLMFP asks the LLM to detail the requirements of each variable before code information in a mathematical formulation of an optimization problem. He writes code that code the problem and calls the joint optimization solver, which arrives at an ideal solution.
“This is similar to the way we teach undergraduate students on MIT optimization problems. We do not teach them a single area. We teach them the methodology, ”adds Fan.
As long as the solver inputs are correct, it will give the right answer. All errors in the solution come from errors in the formulation process.
To ensure that he found a work plan, LLMFP analyzes the solution and modifies all the incorrect steps in the formulation of the problem. Once the plan succeeds in this self-assessment, the solution is described to the user in natural language.
Perfect the plan
This self-assessment module also allows the LLM to add all the implicit constraints it has missed the first time, says Hao.
For example, if the frame optimizes a supply chain to minimize costs for a coffee maker, a human knows that coffeeeshop cannot send a negative quantity of roasted beans, but an LLM may not realize it.
The self-assessment stage would indicate this error and encourage the model to repair it.
“In addition, an LLM can adapt to user preferences. If the model realizes that a particular user does not like to modify the time or budget of their travel plans, this may suggest modifying things that meet the needs of the user,” explains Fan.
In a series of tests, their framework has reached an average success rate between 83 and 87% on various various planning problems using several LLM. While some reference models were better for certain problems, LLMFP has reached a global success rate about twice as high as reference techniques.
Unlike these other approaches, LLMFP does not require examples specific to the field for training. He can find the optimal solution to a planning problem as soon as the box is released.
In addition, the user can adapt LLMFP for different optimization solvers by adjusting the prompts powered to the LLM.
“With LLM, we have the possibility of creating an interface that allows people to use tools from other areas to solve problems in a way they might not have thought before,” explains Fan.
In the future, researchers wish to allow LLMFP to take on entry images to complete the descriptions of a planning problem. This would help the framework to solve tasks that are particularly difficult to describe with natural language.
This work was funded, in part, by the Office of Naval Research and the Mit-ibm Watson Ai Lab.
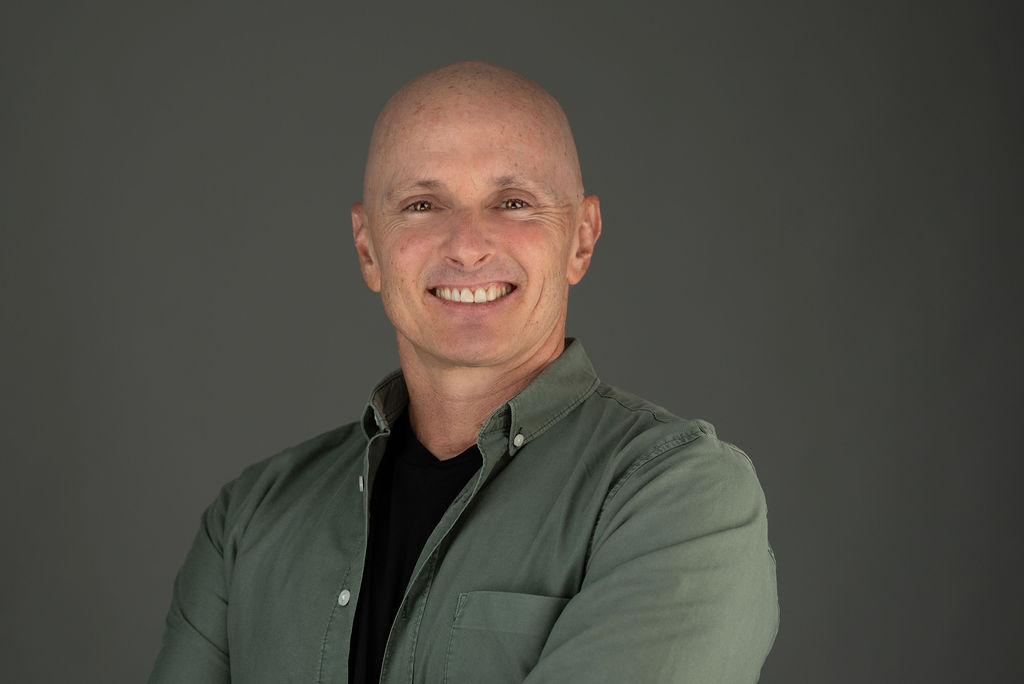