Figure 1 Also indicates the potential of the AI ​​(the robot icon) to contribute to the development of the underlying educational objectives (salt development in this example), as well as to help to mediate both the balancing and the strengthening of the feedback loops (B1 and R2) associated with emotions. Some of these interaction points of interaction are autonomous, where the robot icon appears alone, and some are supervised, where the robot and the mortar coexist. It is important to note that these potential AI support points are entirely indicative and that the examples presented here are general suggestions.
Before exploring Fig. 1 In detail, it is important to underline the cyclical nature of this model. Learning does not occur as a unique event. Education objects (salt development in this example) rather lead to a series of changes in other quantities which are then available for use in future cycles. It is also essential to recognize that the system presented in Fig. 1 is incomplete and forms only one subsystem in a much more complex process. In other words, there will be other factors in the largest system connected to the nodes of our model which are not represented here (for example, resilience). For this reason, it is important that students are experiencing the two successes in learning and difficulties. Everyone will cause different emotional responses, and the wider system will respond in different ways potentially in the development of attributes such as perseverance that are not represented here. All AI implementations that are incorporated into this system must ensure that they are designed to respond appropriately to success and difficulty so as to improve underlying educational objectives without deactivation students or their teachers or shorten learning processes.
Work from our conceptual model (fig. 1), we offer six general areas where AI can be able to provide mechanisms or approaches that support the learning process. From these, we have identified specific learning design elements which can be targeted for research, improvement or modification. These transformation objects and their associated design goals are presented in the table 1 as well as possible AI application strategies and examples of implementation.
First, support for teachers to provide personalized mathematical learning activities at an appropriate level of complexity to correspond to the cognitive capacities of individual students is essential. The forces of current AI approaches are in their ability to imitate human processes, using much greater amounts of data, and with much greater speed than humans can reach. When an AI is presented with a problem similar to those he has already “seen”, it is reasonable to expect the algorithm to follow a similar process in exit production. Consequently, the implementation of a form of semi-sub-supervised algorithm, or even not supervised, to analyze performance and / or mastering data of the past and current learning of each student and suggest learning ways to follow or dynamically adjust the task requests to a appropriate level would be potentially effective use for AI learning support. Such an implementation would appear to â‘ in the figure. 1 And provide personalized challenges that maintain an optimal balance between demand for tasks and the cognitive quality of teaching. These individualized learning experiences will align with the level of competence of each student, minimizing their frustration and improving the probability of successful learning results. However, personalization should not be limited to the difficulty of tasks alone. AI can help identify specific moments in which students fight, providing comments not only focused on tasks but which also supports the positive assessments of their efforts. For example, AI -oriented systems can identify when a student shows signs of frustration or disengagement and offer support comments that highlights their progress and their efforts rather than focusing solely on the completion of tasks. This can help students reinterpret their experiences as learning opportunities, thus promoting a more positive concept of self and increasing their motivation. An algorithm like this would be best suited to the general implementation by resource producers, because they would have access to the large amounts of data necessary to establish the parameters of the algorithm. However, in a semi-sub-supervised arrangement, certain systems or processes should be developed to allow the class teacher to provide entries and refine the algorithm in a simple and intuitive way.
The extension of the idea of ​​performance monitoring to student self-monitoring, AI algorithms can be used to improve the perceptions of agency and control learners and can be used to ② in the figure. 1. Using a large set of learning performance data, a resource producer could develop a predictive model that gives students a selection of next steps recommended for their own personalized learning, but remains in the same area of ​​knowledge that the teacher has allocated. In this conception, the AI ​​algorithm essentially creates a decision tree to build learning paths but leaves the last step of the process, the decision itself, to the student. Built in such a predictive system would be the possibility of learning the analysis that could strengthen the cognitive strengths and weaknesses of each student and provide boost to help the student achieve his own declared objectives. AI compatible tools could also be used in creative learning environments encourage cooperation and thus cultivate positive social interactions. These tools may include interactive and collaborative platforms where students can explore mathematical concepts independently and engage in cooperative problem -solving activities. AI can also contribute to the development of improved feelings of control and growth thanks to structures and expectations of individualized success objectives; approaches that are essential to reduce mathematical anxiety.
AI can also help teachers improve the induction of students' value ③ which is an important facet of the success of learning. AI search engines have access to an almost infinite volume of information that could be used to select contexts or situations that highlight real applications and mathematical problems in a relevant way for individual students. Such an approach can contribute to the understanding of students of the intrinsic value of mathematical knowledge15.
Chatbots based on large languages ​​models (LLM) have become particularly effective in recent years. Although chatbots are still far from perfect, the possibility of relaunching an LLM with an appropriate set of background data files offers educators the possibility of using these algorithms in a safe and closed environment while ensuring that the chatbot has access to only appropriate additional information and not to the whole of the non -filtered web. The adoption of such an approach has the additional advantage that the LLM itself can be smaller, which allows it to operate on local equipment at the prosumer level and delete the need to operate on a massive cloud infrastructure. The use of an AI approach like this to help students moderate feedback loops, such as that ④has great research and impact potential16. As indicated, there is a reinforcement loop between perceptions of control and mathematical anxiety (R1 in Fig. 1). In a traditional classroom, this feedback loop could be motivated by a general reflection on the task and its success criteria analyzing what the student was wrong and how to solve this problem. This type of feedback must be carefully supervised to ensure that unnecessary self-discourse does not dominate the process. An AI chatbot can help crop these reflections to examine how an individual has developed in several ways while engaging with learning activities and reducing the dichotomous accent on success in relation to failure.
In addition, recycling the notions of failure and success of students becomes feasible thanks to AI interventions which emphasize the iterative nature of learning and the value of the underlying educational objective. Such AI tools placed at ⑤ Could promote the use of personal micro-targets for students and keep the cognitive process of learning activity and on this deeper learning objective, AI-focused interventions can lead to a change in students by learning to consider reverse as growth opportunities and reduce anxiety associated with performance.
However, although current AI tools are capable of “making decisions” using large amounts of mainly structured data, they do not have the capacity to understand and adapt truly to the emotional and cognitive needs of students. AI models tend to focus more on teaching mechanics rather than the learner's emotional well-being. In other words, the AI ​​tools currently available are more focused on learning products than on the human learning process. To really exploit the potential of AI in the transformation of math teaching, we must prioritize different research objectives. Researchers should therefore aim to develop new systems and approaches that promote a deeper and intuitive understanding of mathematical concepts, rather than simply improving the efficiency of content delivery.
For example, we know that self -regulation of emotions is essential in order to manage unfavorable situations such as mathematical anxiety. Natural language treatment algorithms can be developed to identify fingerprints of positive and negative emotional meaning in the extensive textual responses of students. This can be supplemented by data collected via multimodal learning analysis – such as computer vision and audio analysis – to follow non -slippery clues such as facial expressions, tonal variation and gestures17. Together, such a set of data could be used to provide support and strategies, virtual and real, which can help students develop their self -regulation and their emotional moderation. Such technology could have a place to ③ Or ⑥ in the figure. 1.
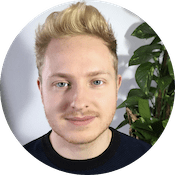
At Learnopoly, Finn has championed a mission to deliver unbiased, in-depth reviews of online courses that empower learners to make well-informed decisions. With over a decade of experience in financial services, he has honed his expertise in strategic partnerships and business development, cultivating both a sharp analytical perspective and a collaborative spirit. A lifelong learner, Finn’s commitment to creating a trusted guide for online education was ignited by a frustrating encounter with biased course reviews.