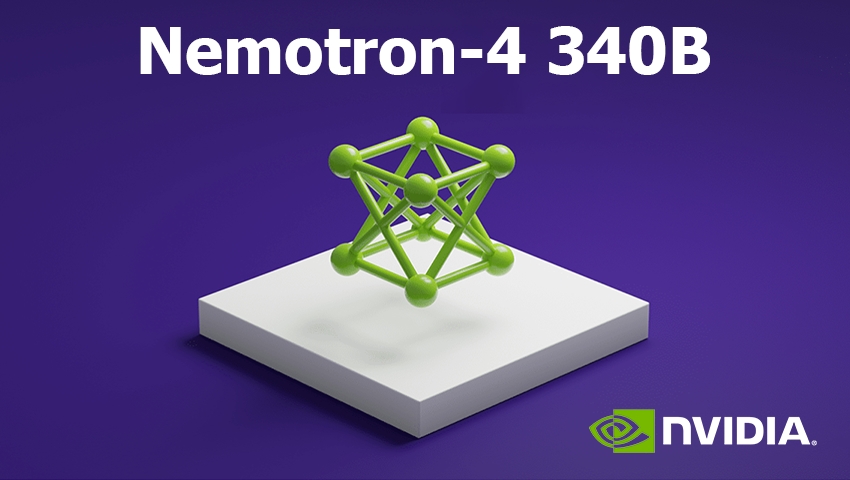
Nvidia introduced the family of the Nemotron-4 340B modelA series of powerful free access models designed to improve the generation of synthetic data and the formation of large language models (LLM). This version includes three distinct models: the Nemotron-4 340B base, the Nemotron-4 340B instruction and the Nemotron-4 340B reward. These models promise to considerably improve AI's capacities in a wide range of industries, including health care, finance, manufacturing and retail.
The main innovation of the Nemotron-4 340B lies in its ability to generate high-quality synthetic data, a crucial component for effective LLM formation. High quality training data is often expensive and difficult to obtain, but with Nemotron-4 340B, developers can create large-scale robust data sets. The fundamental model Nemotron-4 340B has been formed on a large corpus of 9 billions of tokens and can still be refined with proprietary data. The NEMOTRON-4 340B instruct model generates various synthetic data that imitate real world scenarios, while the Nemotron-4 340B reward model ensures the quality of this data by evaluating the responses according to aid, accuracy, consistency, complexity and verbosity.
A remarkable feature of the Nemotron-4 340B is its sophisticated alignment process, which uses both the direct optimization of preferences (DPO) and the optimization of rewarded preferences (RPO) to refine the models. DPO optimizes the model's responses by maximizing the reward deviation between the favorite and not preferred responses, while RPO refines this more considering the differences in reward between the responses. This double approach guarantees that models not only produce high quality outputs but also maintain the balance between various evaluation measures.
NVIDIA used a supervised fine adjustment process (SFT) to improve the capacity of the model. The first step, Code SFT, focuses on improving coding and reasoning capacities using synthetic coding data generated by a genetic instructor – a method that simulates evolutionary processes to create high quality samples. The SFT General SFT stadium has followed to train on a diverse set of data to ensure that the model works well on a wide range of tasks, while retaining its coding competence.
The NEMOTRON-4 340B models benefit from a strong to strong iterative alignment process, which continuously improves models by successive data generation and fine adjustment cycles. Starting with an initial aligned model, each iteration produces better quality data and more refined models, creating a self-reinforced improvement cycle. This iterative process uses both solid basic models and high quality data sets to improve the overall performance of instruct models.
The practical applications of the NEMOTRON-4 340B models are large. By generating synthetic data and an alignment of the refining model, these tools can considerably improve the accuracy and reliability of AI systems in various fields. Developers can easily access these models through NVIDIA NGC,, FaceAnd the next AI.NVIDIA.com platform.
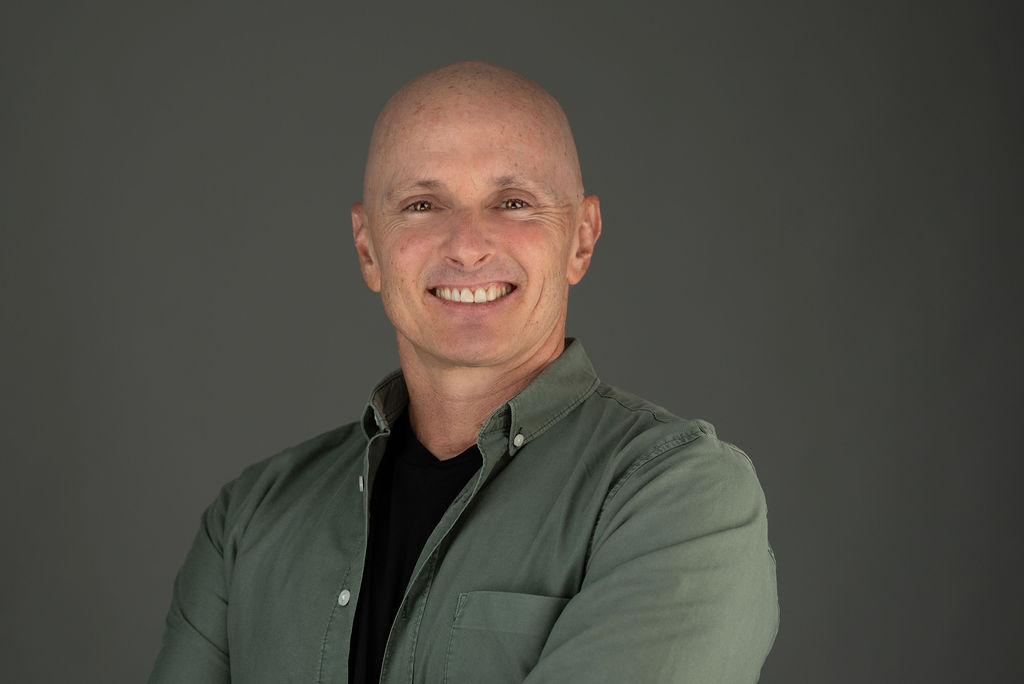