CLOTH proved to be effective in improving the factual accuracy of LLMS by founding their results in external and relevant information. However, most of the existing Rag implementations are limited to textual corpus, which limits their applicability to real world scenarios where requests may require various types of information, ranging from textual definitions to spatial understanding of images or temporal reasoning from videos. Although certain recent approaches have an extensive cloth to manage different methods such as images and videos, these systems are often forced to operate in a single corpus specific to modality. This limits their ability to effectively respond to a wide range of user queries that require multimodal reasoning. In addition, current cloths are generally recovered from all the terms without discernment which is most relevant for a given request, which makes the process ineffective and less adaptive to specific information needs.
To remedy this, recent research highlights the need for adaptive cloth systems to determine the appropriate recovery method and granularity based on the context of the query. The strategies include routing requests based on complexity, such as the decision between no recovery, recovery in a single step or in several steps, and using the model's confidence to trigger recovery only if necessary. In addition, the granularity of recovery plays a crucial role, as studies have shown that indexing of corpus at finer levels, such as specific proposals or video clips, can considerably improve recovery relevance and system performance. Therefore, for Rag to really meet the complex needs of the real world information, it must manage several methods and adapt its depth and its recovery range to specific requests for each request.
Researchers from Kaist and Deepauto.ai present Universalrag, a cloth frame that recovers and integrates knowledge from various sources specific to modality (text, image, video) and multiple levels of granularity. Unlike traditional approaches that integrate all the methods in a shared space, leading to a modality bias, Universalrag uses a routing mechanism dedicated to the modality to select the most relevant corpus dynamically based on the request. It also improves the accuracy of recovery by organizing each modality in corpus specific to granularity, such as paragraphs or video clips. Validated over eight multimodal benchmarks, Universalrag constantly surpasses the basic lines unified and specific to the modality, demonstrating its adaptability to various requests for a request.
Universalrag is a generation framework with recovery that manages requests in various methods and data granulations. Unlike standard cloth models limited to a single corpus, Universalrag separates knowledge in text, image and video, each with fine and coarse grains levels. A routing module first determines the optimal modality and granularity for a given request, choosing from options such as paragraphs, complete documents, video clips or a complete video, and recovers the relevant information accordingly. This router can be either an LLM classifier without training, or a formed model using heuristic labels from reference data sets. A LVLM then uses the selected content to generate the final response.
The experimental configuration assesses Universalrag in six recovery scenarios: no recovery, paragraph, document, image, clip and video. For non-selected, Mmlu tests general knowledge. Tasks at paragraphs use natural questions and questions, while Hotpotqa manages the recovery of multi-hop documents. Image -based queries come from webqa, and those related to video come from LVBENCH and VIDEORAG data, divided into clip and vacuum levels. The corresponding recovery companies are organized for each modality – based on the text for the text, the webqa for images and YouTube videos for video tasks. This complete reference ensures a solid assessment through various methods and recovery granularities.

In conclusion, Universalrag is a generation framework from recovery which can recover knowledge from several terms and levels of granularity. Unlike existing rag methods that are based on a single corpus, often in text only, or a single source, Universalrag dynamically rolls requests to the specific corpus to the most appropriate modality and granularity. This approach addresses problems such as modality gaps and rigid recovery structures. Assessed on eight multimodal references, Universalrag surpasses the unified basic lines specific to the modality. The study also emphasizes the advantages of fine grain recovery and underlines how the routing mechanisms formed and without train contribute to a flexible flexible and flexible multimodal reasoning.
Discover the Paper. Also, don't forget to follow us Twitter And join our Telegram And Linkedin Group. Don't forget to join our 90K + ML Subdreddit. For promotion and partnerships, Please talk to us.
Sana Hassan, consulting trainee at Marktechpost and double -degree student at Iit Madras, is passionate about the application of technology and AI to meet the challenges of the real world. With a great interest in solving practical problems, it brings a new perspective to the intersection of AI and real life solutions.
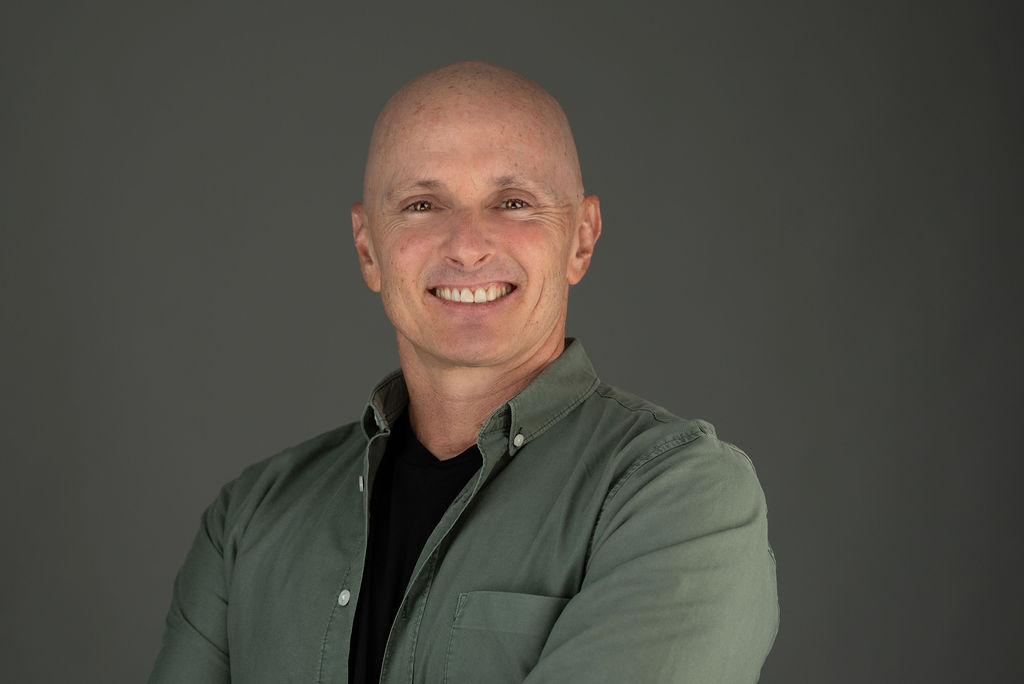