Multimodal foundation models have shown a substantial promise in activating systems that can reason through text, images, audio and video. However, the practical deployment of these models is frequently hampered by material constraints. A high consumption of memory, large counts of parameters and a dependence on high -end GPUs have limited the accessibility of multimodal AI to a close segment of institutions and businesses. As the interest in research increases in the deployment of language and vision models on the edge or on a modest computer infrastructure, there is a clear need for architectures which offer a balance between multimodal capacity and efficiency.
Alibaba Qwen publishes Qwen2.5-OMNI-3B: Expansion of access with an effective model design
In response to these constraints, Alibaba released Qwen2.5 -Mni-3BA variant of parameters of 3 billion in his family of QWEN2.5-omni models. Designed for use on GPUs of consumer quality – in particular those with 24 GB of memory – This model introduces a practical alternative for developers creating multimodal systems without large -scale calculation infrastructure.

Available at Github,, FaceAnd ModelThe 3B model inherits the architectural versatility of the Qwen2.5-Romni family. It supports a unified interface for language, vision and audio input and is optimized to operate effectively in scenarios involving long -context treatment and multimodal interaction in real time.
Model architecture and key technical characteristics
QWEN2.5-OMNI-3B is a model based on a transformer that supports multimodal understanding through text, images and audio-video input. He shares the same design philosophy as his counterpart 7B, using a modular approach where the input encoders specific to the modality are unified via a shared transformer skeleton. In particular, the 3B model considerably reduces general memory costs, reaching 50% reduction in truum consumption When handling long sequences (~ 25,000 tokens).

Key design characteristics include:
- Reduced memory imprint: The model has been specifically optimized to operate on 24 GB of GPU, which makes it compatible with largely available consumer equipment (for example, NVIDIA RTX 4090).
- Extended context treatment: Capable of effectively treating long sequences, which is particularly beneficial in tasks such as reasoning at the document and analysis of video transcripts.
- Multimodal streaming: Supports the audio and video dialogue in real time up to 30 seconds in length, with stable latency and a minimum output drift.
- Multilingual support and discourse generation: Retains the capacities of the release of natural speech with clarity and tone fidelity comparable to model 7B.
Performance observations and evaluation ideas
According to information available on Model And FaceQWEN2.5-OMNI-3B shows performance that is close to variant 7B through several multimodal landmarks. Internal evaluations indicate that it retains More than 90% of understanding capacity From the larger model in tasks involving a visual response to the answer, audio subtitling and the understanding of the video.
In long -context tasks, the model remains stable on sequences up to ~ 25k tokens, which makes it suitable for applications which require a synthesis at the document or reasoning devoted to chronology. In speech -based interactions, the model generates a coherent and natural outing on clips of 30 seconds, maintaining alignment with the input content and minimizing latency – a requirement in interactive systems and human composition interfaces.

Although the number of lower parameters naturally leads to a slight degradation of wealth or generative precision under certain conditions, the global compromise seems favorable to developers in search of a high -utility model with reduced calculation requests.
Conclusion
QWEN2.5-OMNI-3B represents a practical step in the development of effective multimodal AI systems. By optimizing performance per unit of memory, it opens up opportunities for experimentation, prototyping and deployment of language and vision models beyond traditional business environments.
This version deals with a neck of critical strangulation in the multimodal adoption of the AI ​​- the accessibility of the GPU – and provides a viable platform for researchers, students and engineers working with restrictive resources. As the interest increases in the deployment of the edges and the long-context dialogue systems, compact multimodal models such as QWEN2.5-OMNI-3B will probably form an important part of the applied AI landscape.
Discover the model on Github,, FaceAnd Model. Also, don't forget to follow us Twitter And join our Telegram And Linkedin Group. Don't forget to join our 90K + ML Subdreddit.
Asif Razzaq is the CEO of Marktechpost Media Inc .. as a visionary entrepreneur and engineer, AIF undertakes to exploit the potential of artificial intelligence for social good. His most recent company is the launch of an artificial intelligence media platform, Marktechpost, which stands out from its in-depth coverage of automatic learning and in-depth learning news which are both technically solid and easily understandable by a large audience. The platform has more than 2 million monthly views, illustrating its popularity with the public.
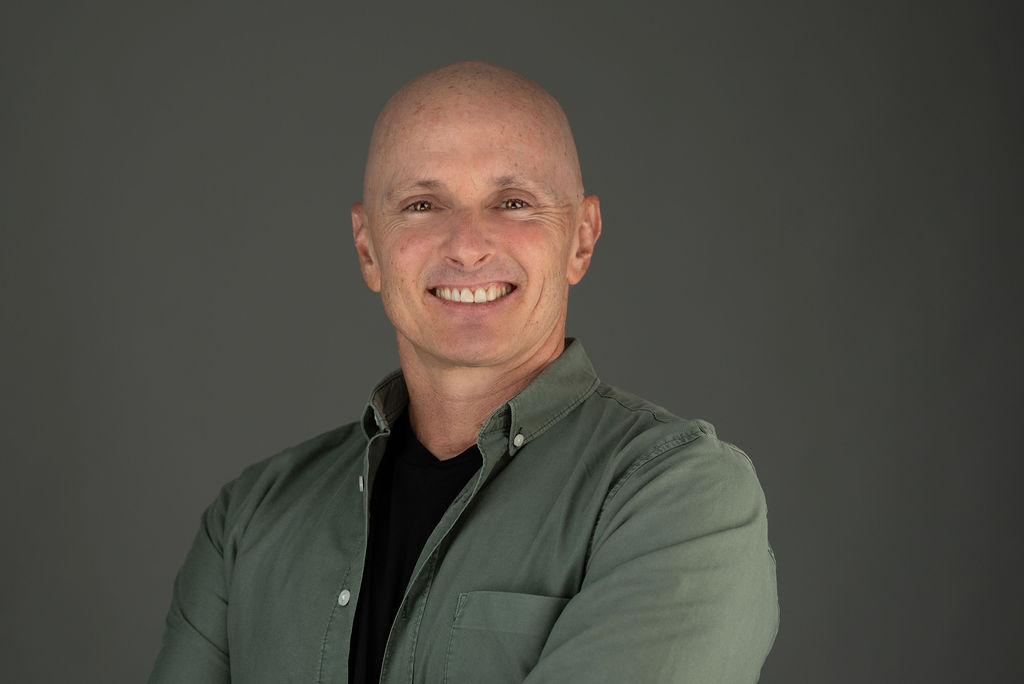