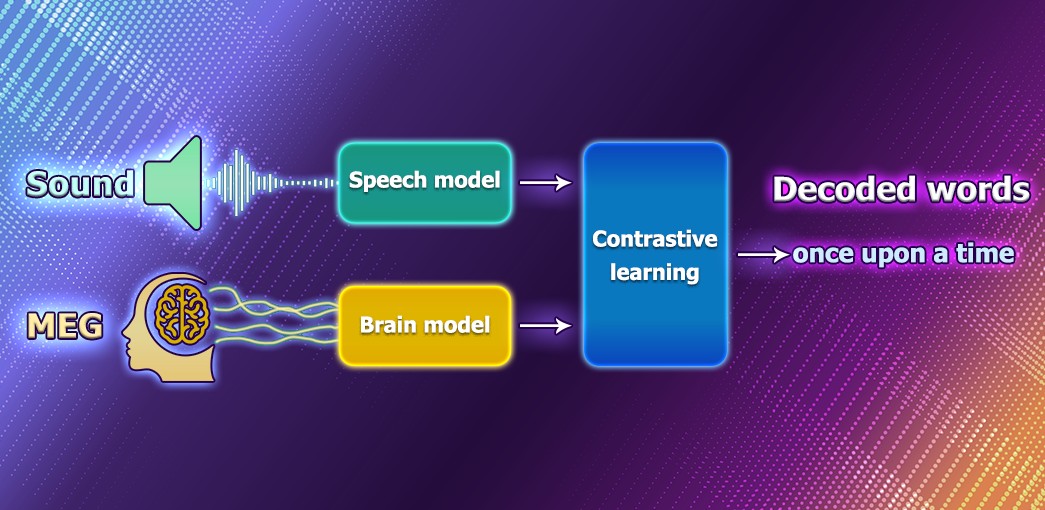
The company shared its research on an AI model which can decode the word from non -invasive recordings of brain activity. It has the potential to help people after a traumatic cerebral lesion, which has left them unable to communicate by speech, dactylography or gestures.
The decoding of speech based on brain activity has been a long -standing objective of neuroscientists and clinicians, but most progress has relied on invasive brain recording techniques, such as stereotactic electroencephalography and electrocorticography.
In the meantime, Meta researchers think that conversion of speech via non -invasive methods would provide a safer and more scalable solution that could ultimately benefit more people. Thus, they created an in -depth learning model formed with contrastive learning, then used it to align non -invasive brain recordings and speech sounds.
To do this, scientists have used an open source self-supervised learning model Wave2 with 2.0 Identify the complex representations of speech in the brain of volunteers while listening to audio books.
The process includes an input of electroencephalography and magnetoecephalography records into a “brain” model, which consists of a standard deep convolutional network with residual connections. Then, the architecture created learns to align the exit of this brain model to the deep representations of the sounds of speech which were presented to the participants.
After training, the system does what is called the classification of zero blows: with an extract from brain activity, it can determine from a large pool of new audio files that the person really heard.
According to Meta: “The results of our research are encouraging because they show that self-surveillance trained can successfully decode the speech perceived from non-invasive recording of brain activity, despite the noise and variability inherent in this data. These results are only a first step. Patients must potentially include new ways of interacting with computers. »»
Learn more about research here
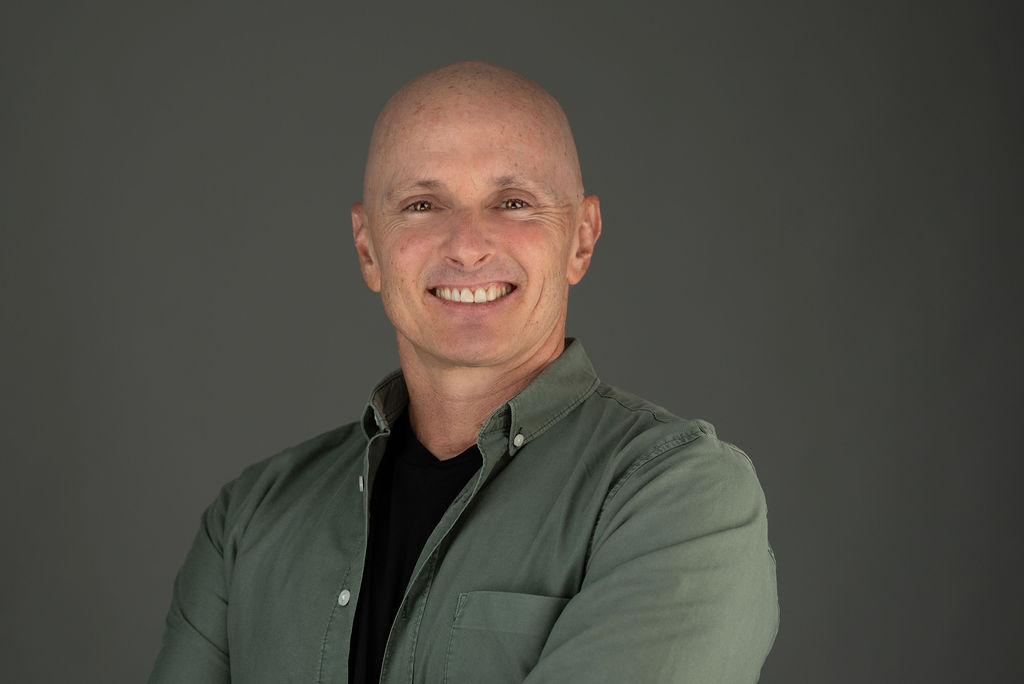