Return the challenges of recovery of reasoning
Despite notable progress in generation with recovery (CLOTH) Systems, the recovery of information relevant for complex reasoning tasks and in several stages remains an important challenge. Most retrievers are today trained on data sets made up of short factual questions, which align well with lexical or semantic overlaps at the document. However, they fail when they are faced with longer, abstract or inter -community queries that require synthesizing dispersed knowledge. In such cases, recovery errors can spread through the pipeline, altering the reasoning downstream by large -language models (LLM). Although LLM -based reframing can improve relevance, their substantial cost of calculation often makes them impractical in real deployments.
Meta Ai presents Reasonir-8b, a retriever built for reasoning
Meta Ai came out Reasonir-8bA retriever model designed explicitly for the search for information with high reasoning intensity. Formed from LLAMA3.1-8B, the model establishes new performance standards on the brilliant reference, achieving a cumulative gain at reduced prices (ndcg @ 10) of 36.9 When used with a light Qwen2.5 Reranker. In particular, it exceeds the main models of replay such as RANK1-32B while offering 200 × lower calculation in lower timeThis makes it much more practical for cloth applications on a scale.
Reasonir-8B is formed using a new data generation pipeline, Reasoning-synthesiswhich builds synthetic requests and pairs of documents that reflect the challenges posed by real reasoning tasks. The model is published on the opening Faceas well as the training code and synthetic data tools, allowing additional research and reproducibility.

Model architecture, training pipeline and key innovations
Reasonir-8B uses a Bi-encumer architectureWhere requests and documents are coded independently in interest and have marked via the similarity of the Cosinus. The formation of the model is based strongly on data generated by synthesis Adapted to reasoning scenarios. The Pipeline Raisonirir-Synthesizer produces two main types of training instances:
- Varied length requests (VL): These are long and information -rich requests (up to 2000 tokens), associated with corresponding documents, encouraging the retriever to effectively manage extended contexts.
- Hard requests (HQ): Derivative of documents organized with a high educational value, these requests are designed to require logical inference. Multi-tours prompts are used to build negative hard—Cocuments which seem superficially relevant but do not contain the necessary reasoning paths.
This approach contrasts with conventional negative sampling methods, which are often based on lexical overlap and are less effective for abstract or multi-hop issues.

In addition, the model's attention mask is changed from the Causal configuration of Llama to a bidirectionalallowing the coder to consider the complete context of the symmetrically request, which is beneficial for non -sequential semantic alignment.
Empirical results on IR and RAG references
Reason-8B suffering from solid performance in several benchmarks:
- Brilliant benchmark (high intensity reasoning recovery):
- 24.4 ndcg @ 10 on original requests
- 29.9 with GPT-4 rewritten requests
- 36.9 with qwen2.5 rerrankingoutperforming the greatest LLM redirankers to a cost fraction
- Generation tasks (RAG) of recovery:
- + 6.4% MMLU improvement on a closed reference basis
- + 22.6% GPQA improvement
These gains are consistent in both standard and rewritten requests, with other improvements observed during the combination of reasoning of 200

Above all, the model continues to improve as REQUIVE LANDSUnlike other retrievers including performance trays or decrease. This suggests that Reasonir-8B can better use the requests rich in information, which makes it particularly well suited to test techniques such as rewriting of a request.
Conclusion
Reasoni-8B addresses a key bottleneck in the recovery of information focused on reasoning by introducing an optimized retriever not only for relevance but also for calculation efficiency. Its design – rooted in a synthetic formation adapted to reasoning, coupled with architectural improvements and centered on data – consists of consistent gains in recovery and cloth tasks.
By publishing the code generation of code data, code base and training generation as open source tools, Meta IA encourages the research community to extend this work to more robust, multilingual and multimodal retrievers. For applications requiring profitable and high quality recovery under reasoning constraints, Reasonir-8B represents a convincing and practical solution.
Discover the Paper,, Cuddling page And GitHub page. Also, don't forget to follow us Twitter And join our Telegram And Linkedin Group. Don't forget to join our 90K + ML Subdreddit.
Asif Razzaq is the CEO of Marktechpost Media Inc .. as a visionary entrepreneur and engineer, AIF undertakes to exploit the potential of artificial intelligence for social good. His most recent company is the launch of an artificial intelligence media platform, Marktechpost, which stands out from its in-depth coverage of automatic learning and in-depth learning news which are both technically solid and easily understandable by a large audience. The platform has more than 2 million monthly views, illustrating its popularity with the public.
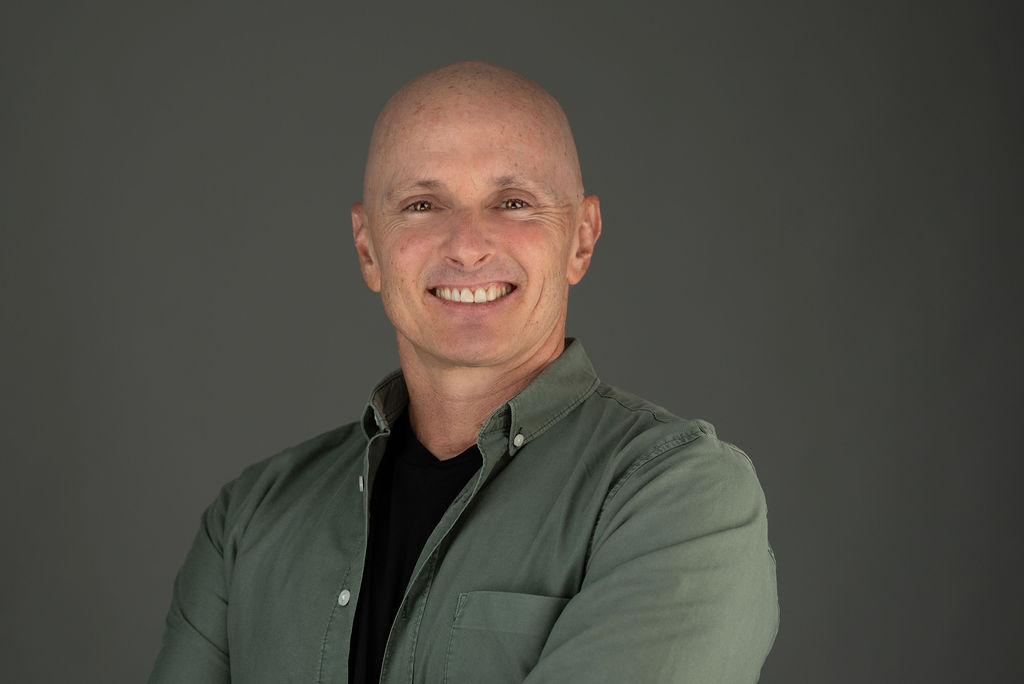