As we have matured since childhood, our vocabulary – as well as the way we use it – develops, and our experiences become richer, which allows us to think, reason and interact with others with specificity and intention. Consequently, our choices of words evolve to align with our personal values, our ethics, our cultural norms and our opinions. Over time, most of us develop an internal “guide” that allows us to learn the context behind the conversation; He also frequently directs us to share information and feelings that are or could be harmful or inappropriate. It turns out that the models of large languages (LLM) – which are formed on sets of extended public data and therefore often have biases and a cooked toxic language – can gain a similar capacity to moderate their own language.
A new MIT method, the Mit-IBM Watson AI Lab, and IBM Research, called self-regressive self-regressive sampling (SASA), makes it possible to detoxify their own results, without sacrificing mastery.
Unlike other detoxifying methods, this decoding algorithm learns a border between the toxic / non-toxic subspaces in the LLM own internal representation, without modifying the models of the model, the need for recycling or an external reward model. Then, during inference, the algorithm assesses the toxicity value of the partially generated sentence: the tokens (words) already generated and accepted, as well as each new potential token which could reasonably be chosen for the proximity of the border of the classifier. Then, it selects a word option that places the sentence in the non -toxic space, ultimately offering a quick and effective way to generate a less toxic language.
“We wanted to discover a means with any existing linguistic model (this), during the generation process, decoding can be subject to certain human values; The example here that we take is toxicity, “explains the main author of the study, Ching-Yun,” Irene “Ko Phd '24, a former graduate trainee in the Mit-Ibm Watson Lab and a current research laboratory of Thomas J. Watson in the New York.
The KO co-authors include Luca Daniel, professor in the Department of Electric and Computer Science (EECS) of MIT, member of the Mit-Ibm Watson AI Lab and graduate advisor to KO; And several members of the Mit-Ibm Watson Ai Lab and / or IBM Research-Pin-Yu Chen, Payel Das, Youssef Mroueh, Soham Dan, Georgios Kollias, Subhajit Chaudhury and Tejaswini Pedapati. The work will be presented at the international conference on representations of apprenticeship.
Find the “railings”
The training resources behind LLM almost always include the content collected from public spaces and the Internet and other easily available data sets. As such, cursed words and unpleasant intimidation / language are a component, although some of them are in the context of literary works. It then follows that the LLM can produce in intrigue – or be deceived in generation – dangerous and / or biased content, which often contains unpleasant words or hateful language, even from harmless invites. In addition, it has been found that they can learn and amplify a language that is not preferred or even detrimental to many downstream applications and tasks – leading to the need for attenuation or correction strategies.
There are many ways to reach a generation of solid equitable languages and aligned with value. Certain methods use LLM recycling with a set of disinfected data, which is expensive, takes time and can modify the performance of the LLM; Others use external decoding reward models, such as sampling or beam search, which take longer to execute and require more memory. In the case of SASA, KO, Daniel and the IBM research team have developed a method that takes advantage of LLM's self -regressive nature, and using a decoding strategy during LLM inference, gradually leads the generation – a token at the same time – far from the non -bounded or unwanted results and towards a better language.
The research group obtained it by building a linear classifier that works on the learned subspace of the incorporation of the LLM. When the LLMs are trained, the words with similar meanings are placed closely in the vector space and further from the different words; The researchers hypothesized that the integration of an LLM would therefore also capture contextual information, which could be used for detoxification. The researchers used sets of data containing sets of an prompt (first half of a sentence or a thought), an answer (the completion of this sentence) and an annotation attributed to humans, as toxic or not toxic, preferred or not preferred, with continuous labels of 0-1, denoting an increasing toxicity. An optimal Bayes classifier was then applied to learn and trace figuratively a line between the binary subspaces in the incorporations of sentences, represented by positive values (non-toxic space) and negative numbers (toxic space).
The SASA system then works by restarting the sampling probabilities of the new potential token depending on the value of it and the distance from the sentence generated in the classifier, in order to stay close to the original sampling distribution.
To illustrate, if a user generates a potential token # 12 In a sentence, the LLM will examine its complete vocabulary for a reasonable word, depending on the 11 words that have preceded it, and using the top-k, top-P, it will filter and produce around 10 tokens to select. SASA then evaluates each of these tokens in the sentence partially completed for its proximity to the classifier (that is to say the value of the 1-11 token, plus each potential token 12). The tokens that produce sentences in positive space are encouraged, while those in the negative space are penalized. In addition, the greater the classifier, the greater the impact.
“The objective is to modify the self -regressive sampling process by rethinking the probability of good tokens. If the following token is likely to be toxic given the context, then we will reduce the probability of sampling so that these are toxic tokens “, explains KO. The researchers have chosen to do this” because the things we say, whether it is benign or not, is subject to the context. “
Tauper toxicity for value correspondence
The researchers evaluated their method against several basic interventions with three LLM of increasing size; All were transformers and based on the self-regressive: GPT2-Gard, LLAMA2-7B and LLAMA 3.1-8B-ISTRUCT, with 762 million, 7 billion and 8 billion parameters respectively. For each prompt, the LLM was responsible for finishing the sentence / sentence 25 times, and Perspectiveapi marked them from 0 to 1, all that is toxic. The team examined two measures: the average maximum toxicity score over the 25 generations for all invites, and the toxic rate, which was the probability of producing at least one toxic sentence on 25 generations. A reduced mastery (and therefore an increased perplexity) was also analyzed. SASA was tested to complete the Realtoxicityptyptspts (RPT), Bold and Attaq data sets, which contained natural English phrases.
The researchers accelerated the complexity of their detoxification trials by SASA, starting with non -toxic prompts from the RPT data set, looking for harmful phrases completion. Then, they intensified it to more difficult RPT prompts which were more likely to produce results, and also applied SASA to the investigation model to assess whether their technique could further reduce unwanted Ouput. They also used Bold and Attaq benchmarks to examine SASA's general applicability in detoxification. With the daring data set, the researchers also looked for a gender bias in languages of languages and tried to reach a balanced gender rate. Finally, the team examined the execution, the use of memory and how SASA could be combined with the filtering of words to achieve a generation of healthy and / or useful languages.
“If we think about how human beings think and react in the world, we see bad things, it is therefore not a question of allowing the language model to see only good things. It is a question of understanding the complete spectrum – both good and bad, “says Ko,” and choose to maintain our values when we speak and act. “
Overall, SASA has made reductions in generation of significant toxic languages, performing equally with RAD, a technique of advanced external reward model. However, it was universally observed that a stronger detoxisation accompanied a decrease in control. Before the intervention, the LLM produced more toxic responses for guests marked by women than males; However, SASA was also able to significantly reduce harmful responses, which makes them more equalized. Likewise, the filtering of words above Sasa has considerably lowered the levels of toxicity, but it also embarrassed the capacity of the LLM to respond in a coherent manner.
A great aspect of this work is that this is a well -defined and constrained optimization problem, explains KO, which means that the balance between the generation of open language which seems natural and the need to reduce adverse language can be reached and regulated.
In addition, says Ko, Sasa may well work for several attributes in the future: “For human beings, we have several human values. We do not mean toxic things, but we also want to be truthful, useful and faithful … If you had to refine a model for all these values, this would require more IT resources and, of course, additional training. ” Due to the light manner of SASA, it could easily be applied in these circumstances: “If you want to work with several values, it is simply a question of checking the position of generation in several subspaces. He only added marginal general costs in terms of calculation and parameters, ”explains KO, leading to a more positive, fair and main language.
This work was supported, in part, by the Mit-ibm Watson AI Lab and the National Science Foundation.
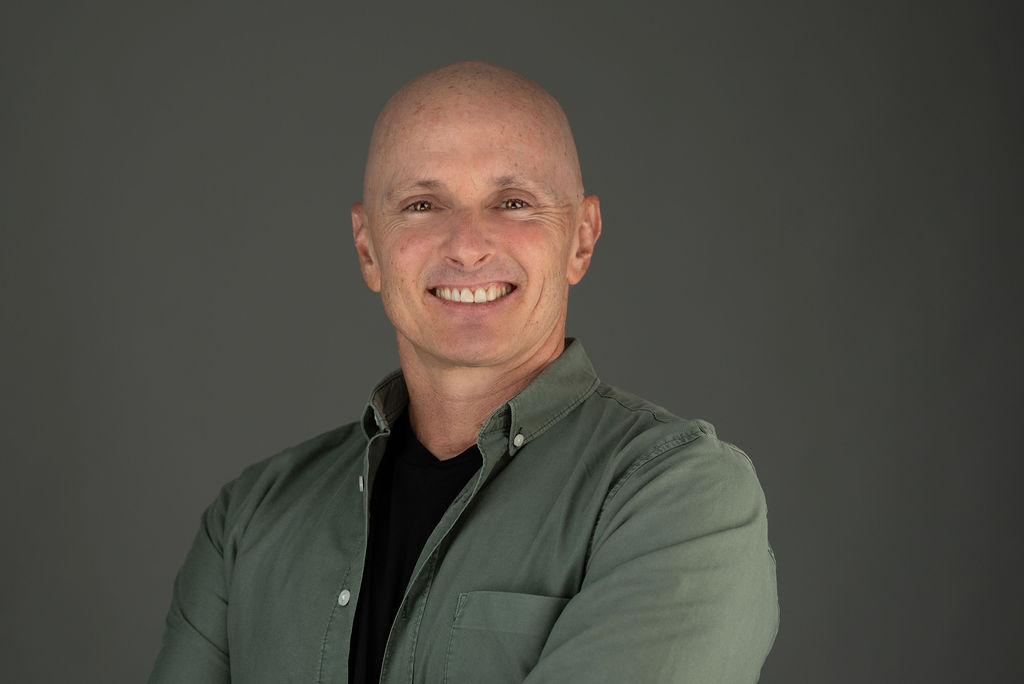