Recent progress in LLM such as OpenAi-O1, Deepseek-R1 and Kimi-1.5 have considerably improved their performance on complex mathematical reasoning tasks. Learning to strengthen verifiable reward (RLVR) is a key contributor to these improvements, which uses rules based on rules, generally a binary signal indicating whether the solution of a model to a problem is correct. Beyond improving the precision of the final outing, the RLVR has also been observed to promote beneficial cognitive behavior such as self-reflection and improve generalization between tasks. Although many research has focused on optimizing strengthening learning algorithms such as PPO and GRPO for greater stability and performance, the influence of training data – its quantity and quality – is less understood. Questions on quantity and data type are really effective for RLVR always open, despite certain work such as LIMR introducing measures to identify impactful examples and reduce the size of the data set while retaining performance.
Unlike in -depth research on the selection of data in supervised fine adjustment and strengthening learning based on human feedback, the role of data in the RLVR has experienced limited exploration. Although LIMR has shown that using a small data subset (1.4K on 8.5K of examples) could maintain performance, it did not examine the extreme case of minimum data use. Another simultaneous study revealed that even training with only four PPO examples has led to notable improvements, but this finding has not been deeply studied or compared against full data performance. Although the RLVR is very promising to improve reasoning in LLMs, a more deep and systematic study of the efficiency and the selection of data in this context is still lacking.
Researchers from the University of Washington, the University of Southern California, Microsoft, the University of California, Santa Cruz and Georgia Institute of Technology show that RLVR can considerably improve mathematical reasoning of large language models using an example of unique training, a 1 shot. Apply it to Qwen2.5-MAT-1.5B improves its MATH500 accuracy from 36.0% to 73.6%, corresponding to the much larger dataset performance. Improvements are generalized between models, tasks and algorithms. The study also reveals effects such as the generalization of the crossed domain, the increase in self-reflection and post-saturation generalization, and highlights the roles of the loss of political gradient and exploration focused on entropy.
The study examines to what extent the RLVR training data set can be reduced while retaining performance comparable to the full data set. Remarkably, the authors note that a single training example – RLVR -Shot – can considerably stimulate mathematical reasoning in LLM. The study shows that this effect is generalized between tasks, models and domains. Interestingly, training on an example often improves the performance of unrelated areas. A simple data selection strategy based on the variance in the precision of the training is offered, but the results show that even random examples can produce major gains.
The study assesses their method using Qwen2.5-math-1.5b as main model and other models such as QWEN2.5-MATH-7B, LLAMA-3.2-3 B-PLAINTT and DEEPSEEK-R1-DISTILLQWEN-1.5 BB. They use a subset of 1,209 examples of the DEEPSCALER data set for the selection of data and the mathematical data set for comparison. The formation involves the Verl pipeline, with carefully chosen hyperparameters and lots configurations. Surprisingly, the formation with only one or two examples – in particular π1 and π13 – behaves with a strong generalization, even beyond mathematical tasks. This “post-saturation generalization” persists despite the signs of over-adjustment. The study also finds an increase in the model self-reflection and shows that even simple examples can considerably improve performance between areas.
In conclusion, the study explores the mechanisms behind the success of the RLVR at 1 stroke, demonstrating that the basic models already have strong reasoning capacities. Experiences show that even a single example can considerably improve the performance of reasoning tasks, suggesting the capacity inherent in the reasoning of the model. The study stresses that the loss of political gradient is the key to RLVR's efficiency to 1 blow, the loss of entropy further improving performance. In addition, encouraging exploration through techniques such as the regularization of entropy can improve post-saturation generalization. The results also highlight the need for careful selection of data to optimize model performance, especially in data -related scenarios.
Discover the Paper And GitHub page. Also, don't forget to follow us Twitter And join our Telegram And Linkedin Group. Don't forget to join our 90K + ML Subdreddit.
Sana Hassan, consulting trainee at Marktechpost and double -degree student at Iit Madras, is passionate about the application of technology and AI to meet the challenges of the real world. With a great interest in solving practical problems, it brings a new perspective to the intersection of AI and real life solutions.
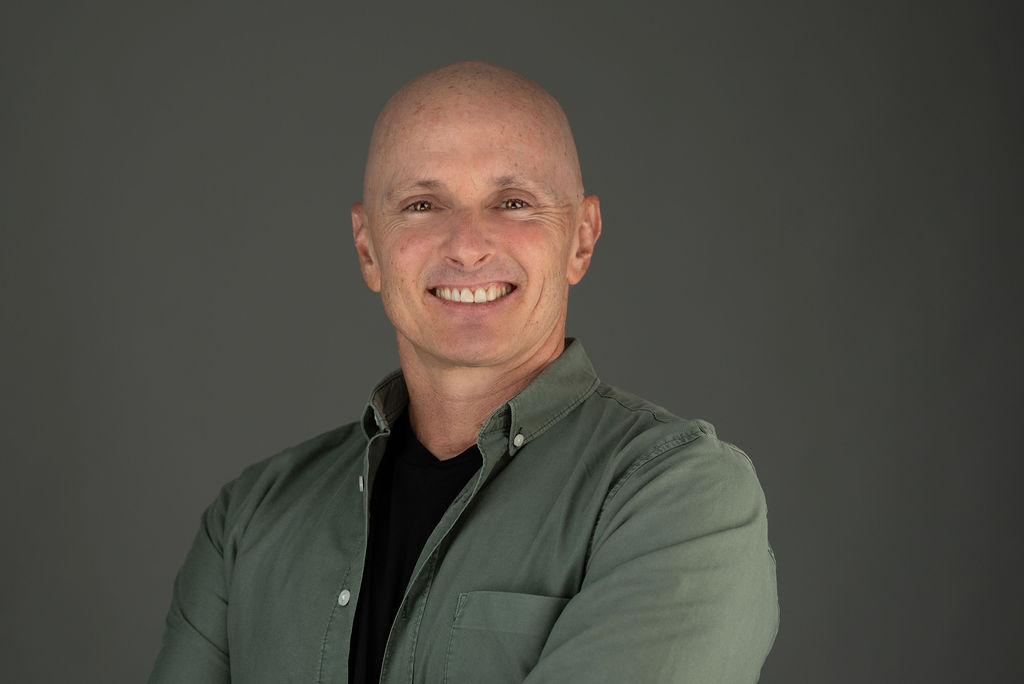