Why better prompts lead to better learning and why
What I found is that generative AI (Gen AI) becomes a real partner in learning to design when we approve it with a goal. It's not just saving time. It is a generator prototype, a resonance box and, when he has invited – a rich, personalized and reusable co -active of learning assets. The key is not only in the use of artificial intelligence (AI) – it is in the way we invite it and, more importantly, with whom we invite it. As an educational designer, I constantly seek means to extend our work without compromising quality or intention. The demand for apprenticeship content in a timely manner, engaging and aligned with the results continues to grow between departments, campuses and organizations. Responding to this request is not only wondering to work faster; It is a question of working smarter and more in collaboration.
Some of the most effective prompts I used were not designed in isolation. They came out of the live boxing sessions with teachers, experts in matters (SMEs), team leaders and even learners themselves. Because when we invite ourselves together, we do not only generate content – we build a shared understanding. This understanding is transformed into models, not unique. In systems, not just solutions. Let's explore how to do it using three interconnected frames – starting with the one I come back the most.
The invitation is design – not just an order
In the educational design, we use frames such as added, sam and bloom taxonomy to provide a structure and clarity to what we build. Inviting, when it is done well, is no different. It is not a question of a line that we throw to a machine – it is an intentional design movement.
When we align the creation of prompts with reflected frames, we get better outings. But more importantly, we create evolutionary, reproducible and teachers systems than other members of our team can use and adapt. One of the simplest and most powerful tools I use to do so is the Pentagon model.
The Pentagon model: make the prompts transferable
The Pentagon model breaks down the key ingredients with a well -structured prompt into five central components: character, context, task, outing and constraint. When each of them is clearly defined, the prompt becomes specific enough to provide relevant and general enough results to be reused in different learning scenarios. Decompos that:
- Persona is a question of role
Who is the AI ​​who responds? A teacher, a nurse, a coach, a historian? Giving AI a defined character gives his voice, his perspective and his exit credibility. - The context supervises the environment or the situation
Is the content intended for integration, clinical practice, student projects or leadership coaching? About this context guarantees that AI understands how to adapt its answer. - The task clarifies the goal
Ask us to summarize, generate a dialogue, simulate a scenario or create a outline? A clearly defined task maintains the focused and useful output. - The output defines the format
Do we need a flea list, a dialogue script, a quiz, a graphic? By defining this expectation, we reduce edition and improve conviviality. - The constraint adds railings
Should the tone be conversational or academic? Should the answer be within a limit of 200 words? Should he be appropriate for learners with different levels of reading?
Using the Pentagon model, the teams can be rapid co-models which are not linked to a situation but can be adapted between the departments and the use cases. For example, an prompt that we originally created to generate case of nursing case studies was later adapted to HR integration materials, simply by refining the role, the public and the context. The structure remained the same, which meant that the process did not have to start from scratch. This is how we extend content creation with intact consistency and quality.
Design thought: encourage as a team process
While the Pentagon model provides the anatomy of a good prompt, design thinking provides the state of mind. He invites empathy, iteration and collaboration, which all makes more significant and lasting incentives. Design thought is not only for product development – it is a creative way and centered on man to write better IA prompts. Instead of jumping directly at the exit, you enter the user's shoes, experience and refine. The goal? Prompts that make answers in AI more useful, personalized and usable.
When educational designers work side by side with teachers, staff and learners to create prompts, something important happens: we stop guessing what people need and start to build with them. Incitation becomes less a solo act and the more a co-creation process.
In a project, we have developed a set of IA prompts to simulate scenarios for resolution of real world conflicts for a professional development course. But rather than simply design the content ourselves, we invited managers, support staff and even trainees in the incentive session. Their lived experiences have shaped the tone, the complexity and the vocabulary of the scenarios. The result? Contents that immediately seemed real and useful – because it was.
This collaborative approach accelerates iteration and increases membership. Instead of revisiting and revising the content after it has missed the brand, you align yourself from the start. And because knowledge is shared, the process becomes evolving. Other members of the organization can adopt the same design approach and generate new content without depending on a single goalkeeper or a team.
Arrorerated design: align prompts on learning objectives
If the Pentagon model gives you the structure and design thought brings collaboration, the backward design guarantees that everything we create supports the learning results. The backward design for IA prompts is well -known Wiggins and MCTIGHE framework, but with a torsion: it is a question of making prompts that get the results you really need. Whether you ask the AI ​​to help design a lesson, write a script, generate images or break down the data, this approach helps you stay focused on the results, not just outings.
The backward design begins at the end of the mind: what should know, do or feel the learners after this experience? From there, we decide how we will measure success (evaluation), and it is not until this time that we conceive the learning experience and the prompts to support it.
For example, in customer service training, we needed learners to demonstrate empathy and problem solving skills in real -time conversations. Instead of starting by asking AI to “write a scenario”, we started with the learning objective: “employees will defuse a frustrated customer using active listening techniques.” This has led the task (“Create a realistic conversation”), the context (“in a retail framework with long waiting times”), and the exit (“a game script with tagged loudspeakers”).
Because we have linked the invitation to a performance objective, the exit was immediately aligned. Better still, the structure could be reused in different industries – simply substitute a hospital, a university or a call center as a framework, and the same executive applies. The invites rooted in the results do not derive. They evolve, translate and evolve.
Why invitation should be a habit of collaboration
Working with AI can feel quickly, but working with AI together, using a fast shared model, is not only faster but smarter. When we involve stakeholders at the start of the incitement process, we avoid the typical back and forth that comes from poorly aligned expectations. Co-metal prompts reflect real needs, use shared language and generate reusable formats. Over time, these prompts are part of your design toolbox – a library of modular components that you can mix, match and adapt.
Even more powerful? Facing in collaboration is a form of update. Professors, staff and designers learn to talk together about the language of AI. They begin to think in frames, articulate the tasks more clearly and use the AI ​​more effectively by themselves. Incitation becomes shared literacy – and that's what makes it durable.
Build a culture of evolutionary incentive
Content scaling does not mean creating more from scratch. This means creating smarter and reusable systems thanks to collaboration. AI can help you, but only when we use it with intention and when we invite for the purpose. Here is what I learned really works:
- Use frameworks such as pentagon model, design thinking and back design to structure your prompts
- Involve the stakeholders early, not just at the exam stages
- Build shared prompt models and store them where others can easily access and adapt them
- Hostez Jam Pristles sessions during planning or sprint cycles to normalize practice
In short: Treat incentive as design. Make it collaborative, useful and reproducible. You will move faster. You will align yourself better. And above all, you will build an learning ecosystem where the content is not only generated – it is strategically created, built in the community and done on a scale.
Read more in -depth:
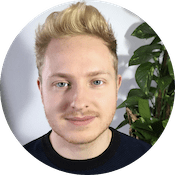
At Learnopoly, Finn has championed a mission to deliver unbiased, in-depth reviews of online courses that empower learners to make well-informed decisions. With over a decade of experience in financial services, he has honed his expertise in strategic partnerships and business development, cultivating both a sharp analytical perspective and a collaborative spirit. A lifelong learner, Finn’s commitment to creating a trusted guide for online education was ignited by a frustrating encounter with biased course reviews.