Daily writing prompt
What little improvement you can make in your life?
By Kavita Dehalwar
Modeling user behavior for public transport users is an essential company in town planning, transport engineering, behavioral economy and intelligent mobility systems. It helps to understand how and why individuals make certain transit choices, which can shed light on the development of infrastructure, the development of policies, forecasting demand and the design of services. This trial describes a complete approach to modeling the behavior of public transport users, encompassing theoretical foundations, methodologies, data sources, modeling techniques and practical applications.

1. Introduction
Public transport systems are essential for sustainable urban development. Understanding user behavior in these systems is necessary to design effective, friendly and environmentally friendly transport networks. The modeling of user behavior involves identifying the factors that influence the travel decisions of individuals, such as the choice of mode, the selection of routes, the departure time and the frequency of use. The precise modeling of this behavior allows an improvement in system performance, reduced congestion and increased suburban satisfaction.
2. Theoretical foundations
2.1 Behavioral theories
Several behavioral theories underlie the modeling of travel behavior:
- Rational choice theory Supposes that individuals make decisions that maximize their usefulness according to journey time, cost, convenience and reliability.
- Planned behavior theory (TPB) Includes attitudes, subjective standards and behavioral control perceived to predict intention and behavior.
- Usual behavior theory emphasizes that not all decisions are aware or rational; Many are usual and influenced by routine.
- Delimited rationality suggests that decision -makers are targeting satisfactory rather than optimal solutions due to cognitive limitations.
2.2 Theory of utility
In the modeling of discreet choices, users are supposed to choose the option with the highest perceived utility. Utility is generally a function of measurable variables such as travel time and cost, as well as non -observable preferences.
3. Collection and data sources
Effective modeling requires high quality data. Current sources include:
- Surveys (For example, travel newspapers, indicated preferences (SP) and preferably revealed surveys (RP))
- Smart cards data (For example, time and treadpower times and locations)
- Mobile phone data data
- Social media and web scratching For feeling and location
- Automatic passenger metering systems (APC)
- CCTV and Wi-Fi / Bluetooth monitoring
Each data source offers different ideas and granularity, and often several sources are integrated for full modeling.
4. Modeling methodologies
4.1 Descriptive analysis
Basic statistical analysis helps to understand general models, such as peak hours of use, favorite routes and user demographic data.
4.2 Discreet choice models (DCMS)
These are the most used tools to model individual travel decisions. Examples include:
- Multinomial logit (MNL)
- Nested logit
- Mixed logit / Logit random parameters
These models estimate that a user will choose a special option from a finished alternative set.
4.3 Modeling based on agents (ABM)
Abms simulates individual agents (users) and their interactions within a transport network. This method captures emerging phenomena, such as congestion and modal change, based on user rules and preferences.
4.4 Automatic learning approaches
Recent progress includes the use of:
- Decision trees, random forests
- Neural networks
- Support vector machines (SVM)
- In -depth learning for model recognition
These are data -based methods that often surpass traditional models in prediction precision but can lack interpretability.
4.5 hybrid models
The combination of statistical methods with automatic learning or behavioral theory allows more robust and explainable models.
5. Factors influencing user behavior
Several variables influence the behavior of transportation users:
- Train and reliability
- Cost (price, fuel, tolls)
- Comfort and convenience
- Frequency and service coverage
- Structure and security
- Environmental awareness
- Socioeconomic characteristics (age, income, profession)
- Weather conditions
- Availability of information in real time
Understanding the relative importance of these factors is crucial for targeted interventions.
6. Applications of user behavior models
6.1 Transport planning
Behavior models help optimize routes, schedules and capacity planning.
6.2 Program forecast
The models predict how many people will use certain services in variable scenarios, such as tariff changes or new infrastructure.
6.3 Political simulation
Scenarios such as congestion pricing, subsidies or vehicle restrictions can be tested practically.
6.4 Integration of intelligent mobility
Behavior modeling informs the integration of services such as the sharing of bicycles, carpooling and micro-transit.
6.5 Personalized travel recommendations
The modeling of real -time behavior supports personalized route suggestions and service alerts.
7. Challenges and limitations
- Data confidentiality concerns
- Transferability of the model in cities
- Behavioral complexity and non-linearity
- Technological and infrastructure constraints
- Equity considerations
Efforts must be made to meet these challenges, in particular by ensuring ethical use of data and avoiding biases.
8. Future directions
- Real -time adaptive models This update with live data
- Integration with intelligent city platforms
- Use of portable devices and IoT sensors
- Explanable IA for transparent decision -making
- Behavioral and gamification cuts to influence the choice
The future of transport behavior modeling lies in the dynamic, personalized and predictive systems supported by the AI ​​and omnipresent data.
9. Conclusion
The modeling of user behavior in public transport is a multifaceted task requiring a mixture of theoretical insight, empirical data and advanced analyzes. As cities develop and mobility requests are changing, robust user behavior models will be essential to create adaptive, effective and user transport systems. By adopting interdisciplinary methods and emerging technologies, stakeholders can not only predict how people move, but also shape the future of urban mobility.
References
Buling, RN and Kanaroglou, PS (2007). Research on Transport activity behavior: conceptual problems, advanced and emerging perspectives on behavioral analysis and simulation modeling. Transport reviews,, 27(2), 151-187.
Clifton, KJ and Handy, SL (2003). Qualitative methods in travel behavior research. In Survey quality and transport innovation (pp. 283-302). Emerald Group Publishing Limited.
Dehalwar, K. and Sharma, SN (2025). Social injustice inflicted by spatial changes in vernacular contexts: an analysis of published literature.
Dehalwar, K. and Sharma, SN (2025). Fundamentals of writing research and use of research methodologies. EDUDODIA PUBLICATIONS PVT Ltd.
Dehalwar, Kssn and Sharma, SN (2025). Exploration of distinctions between quantitative and qualitative research methods. Think India Journal,, 27(1), 7-15.
Jones, PM, ten, MC, Clarke, Mi and Heggie, IG (1983). Understanding travel behavior (No. Monograph).
Kutter, E. (1973). An individual travel behavior model. Urban studies,, 10(2), 235-258.
Pel, AJ, Bliemer, MC and Hoogendoorn, SP (2012). An examination of the modeling of travel behaviors in dynamic traffic simulation models for evacuations. Transportation,, 3997-123.
Sharma, SN and Dehalwar, K. (2025). Evaluate the development and transit -oriented travel behavior of residents of developing countries: a case of Delhi, India. Journal of Urban Planning and Development,, 151(3), 05025018.
Sharma, SN, Kumar, A. and Dehalwar, K. (2025). Precursors of transit oriented development. EPW Economic & Political Weekly,, 59(16), 14.
Sharma, SN (2019). Examination of most urban growth models used. International Journal of Advanced Research in Engineering and Technology (Ijaret),, 10(3), 397-405.
Van Acker, V., Van Wee, B., and Witlox, F. (2010). When the geography of transport meets social psychology: towards a conceptual model of travel behavior. Transport reviews,, 30(2), 219-240.
Wardman, M. (1988). A comparison of the revealed preference and the preferably indicated models of travel behavior. Journal of Transport Economics and Policy71-91.
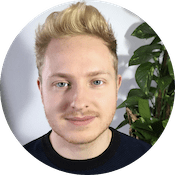
At Learnopoly, Finn has championed a mission to deliver unbiased, in-depth reviews of online courses that empower learners to make well-informed decisions. With over a decade of experience in financial services, he has honed his expertise in strategic partnerships and business development, cultivating both a sharp analytical perspective and a collaborative spirit. A lifelong learner, Finn’s commitment to creating a trusted guide for online education was ignited by a frustrating encounter with biased course reviews.