The models of large languages (LLM) have gained significant terrain in reasoning tasks, including mathematics, logic, planning and coding. However, a critical challenge emerges during the application of these models to real world scenarios. Although current implementations generally work by assuming that all the necessary information is provided initial in well -specified tasks, the reality often presents incomplete or ambiguous situations. Users frequently omit crucial details when formulating mathematical problems, and autonomous systems such as robots must operate in environments with partial observability. This fundamental inadequacy between the idealized complete information parameters and the incomplete nature of the real world problems requires LLM to develop proactive information collection capacities. Recognizing information gaps and generating relevant clarification issues represent an essential but underdeveloped functionality so that LLMs to navigate effectively in ambiguous scenarios and provide specific solutions in practical applications.
Various approaches have attempted to take up the challenge of collecting information in ambiguous scenarios. Active learning strategies acquire sequential data thanks to methods such as Bayesian optimization, learning to strengthen and planning robots with partially observable states. Research on ambiguity in natural language has explored semantic uncertainties, answers to factual questions, dialogues focused on tasks and personalized preferences. The methods of upgrading questions for LLM include direct incentive techniques, information gain calculations and clarification frames in several stages. However, most existing benchmarks focus on subjective tasks where multiple valid clarification issues exist, which makes objective evaluation difficult. These approaches deal with ambiguous or knowledge-based tasks rather than under-specified reasoning problems, when an objectively correct question is determinable.
Questbench presents a robust approach to assess the capacity of LLMS to identify and acquire missing information in reasoning tasks. The methodology formalizes under-specified problems such as Constraint satisfaction problems (CSP) When a target variable cannot be determined without additional information. Unlike semantic ambiguity, where several interpretations exist, but each gives a resolble response, subcupecification makes problems insoluble without additional data. Questbench focuses specifically on “CSPS, 1 -sufficiently” – problems requiring knowledge of an unknown variable value to solve the target variable. The reference includes three separate areas: Logic-q (logical reasoning tasks), planning-q (blocks global planning problems with partially observed initial states) and GSM-Q / GSME-Q (School mathematical problems in verbal and equation forms). The framework strategically categorizes the problems along four axes of difficulty: number of variables, number of constraints, required research depth and expected assumptions necessary for raw force research. This classification offers an overview of LLMS reasoning strategies and performance limitations.
Questbench uses a formal framework of a problem of satisfaction of stress constraints, identify and precisely assess information gaps in reasoning tasks. A CSP is defined as a tuple ⟨x, D, C, A, Y⟩ where x represents variables, D designates their areas, C includes constraints, A consists of variable assignments and is the target variable to be resolved. The framework introduces the “known” predicate, indicating when the value of a variable is determinable either by direct attribution or in bypass from existing constraints. A CSP is classified as sub-specialized when the target variable cannot be determined from the information available. The methodology is specifically focused on “CSP 1-Suffering”, where knowledge of a single additional variable is sufficient to resolve for the target.
The reference measures the performance of the model on four axes of difficulty which correspond to the algorithmic complexity: total number of variables (| x |), total number of constraints (| c |), depth of the rear research tree (D) and expected number of required random assumptions (????BF). These measures provide quantitative measures of the complexity of the problem and help differentiate semantic ambiguity (valid multiple interpretations) and sub-specialty (missing information). For each task, the models must identify the unique sufficient variable which, once known, allows the resolution of the target variable, requiring both the recognition of information gaps and strategic reasoning on constraint relationships.
Experimental assessment of Quest reveals that variable capacities among the main models of large -scale language in information collection tasks. Overview GPT-4O, GPT-4-O1, Claude 3.5 Sonnet, Gemini 1.5 Pro / Flash, Gemini 2.0 Flash Thinking Experimental and the provisional Gemma Open models were tested on zero-shot parameters, chain of thoughts and four strokes. Tests have been carried out on representative subsets of 288 GSM-Q and 151 GSME-q tasks between June 2025 and March 2025. Performance analysis along the axes of difficulty shows that the models are most fighting with problems stalking depths of research and complex relationships. The incentive in chain of thoughts has generally improved the performance of all models, which suggests that explicit reasoning pathways help identify information gaps. Among the models evaluated, Gemini 2.0 Flash Thinking Experimental has reached the greatest precision, in particular on planning tasks, while open-source models have shown competitive performance on logical reasoning tasks but fought with complex mathematical problems requiring more in-depth research.
Questbench provides a single framework to assess the ability of LLMS to identify sub-specialized information and generate appropriate clarification issues in reasoning tasks. Current advanced models demonstrate reasonable performance on simple algebra problems, but considerably fight with complex logic and planning tasks. Performances deteriorate as the complexity of the problem increases along the key dimensions such as the depth of research and the expected number of brute force assumptions. These results emphasize that if the reasoning capacity is necessary for an effective questioning, it may not be sufficient. Important advancement opportunities exist in the development of LLM which can better recognize the shortcomings of information and request clarification when they operate in uncertainty.
Discover the Paper. Also, don't forget to follow us Twitter And join our Telegram And Linkedin Group. Don't forget to join our 90K + ML Subdreddit.
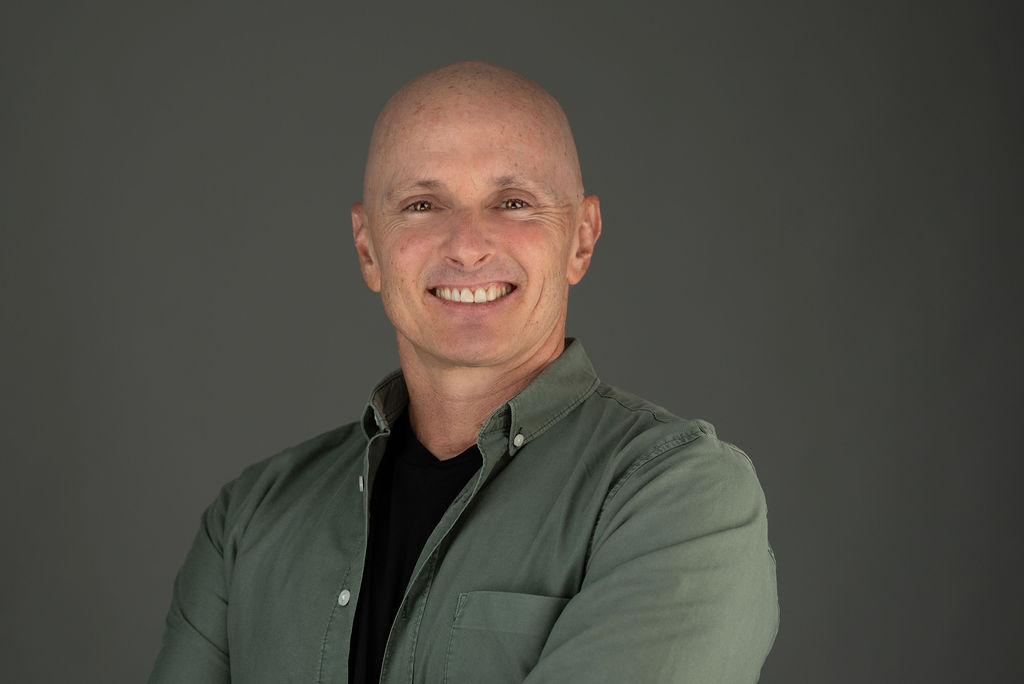